Meta-Calibration Regularized Neural Networks
CoRR(2023)
摘要
Miscalibration-the mismatch between predicted probability and the true correctness likelihood-has been frequently identified in modern deep neural networks. Recent work in the field aims to address this problem by training calibrated models directly by optimizing a proxy of the calibration error alongside the conventional objective. Recently, Meta-Calibration (MC) showed the effectiveness of using meta-learning for learning better calibrated models. In this work, we extend MC with two main components: (1) gamma network (gamma-net), a meta network to learn a sample-wise gamma at a continuous space for focal loss for optimizing backbone network; (2) smooth expected calibration error (SECE), a Gaussian-kernel based unbiased and differentiable ECE which aims to smoothly optimizing gamma-net. The proposed method regularizes neural network towards better calibration meanwhile retain predictive performance. Our experiments show that (a) learning sample-wise gamma at continuous space can effectively perform calibration; (b) SECE smoothly optimise gamma-net towards better robustness to binning schemes; (c) the combination of gamma-net and SECE achieve the best calibration performance across various calibration metrics and retain very competitive predictive performance as compared to multiple recently proposed methods on three datasets.
更多查看译文
关键词
neural networks,meta-calibration
AI 理解论文
溯源树
样例
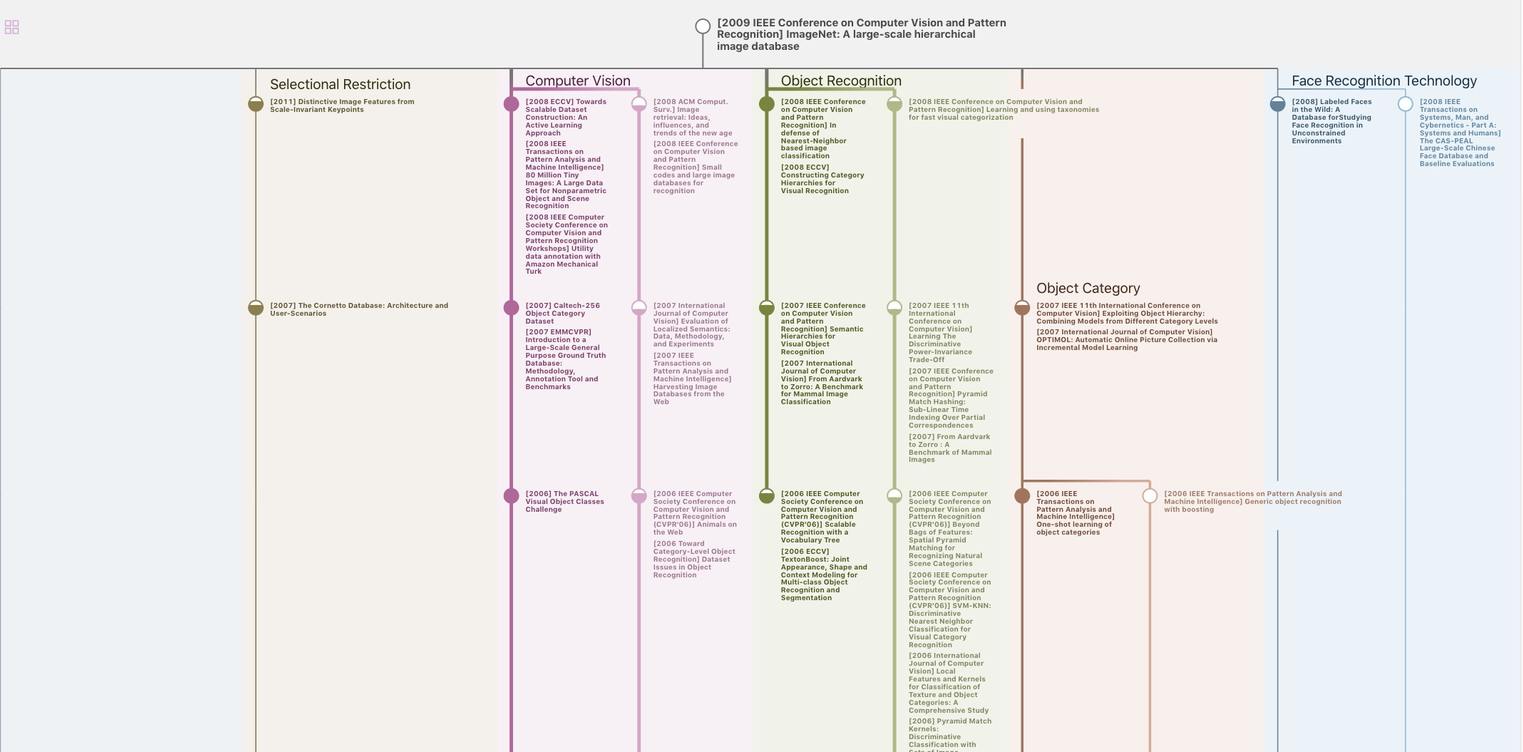
生成溯源树,研究论文发展脉络
Chat Paper
正在生成论文摘要