LEURN: Learning Explainable Univariate Rules with Neural Networks
CoRR(2023)
摘要
In this paper, we propose LEURN: a neural network architecture that learns univariate decision rules. LEURN is a white-box algorithm that results into univariate trees and makes explainable decisions in every stage. In each layer, LEURN finds a set of univariate rules based on an embedding of the previously checked rules and their corresponding responses. Both rule finding and final decision mechanisms are weighted linear combinations of these embeddings, hence contribution of all rules are clearly formulated and explainable. LEURN can select features, extract feature importance, provide semantic similarity between a pair of samples, be used in a generative manner and can give a confidence score. Thanks to a smoothness parameter, LEURN can also controllably behave like decision trees or vanilla neural networks. Besides these advantages, LEURN achieves comparable performance to state-of-the-art methods across 30 tabular datasets for classification and regression problems.
更多查看译文
关键词
explainable univariate rules,neural networks,learning
AI 理解论文
溯源树
样例
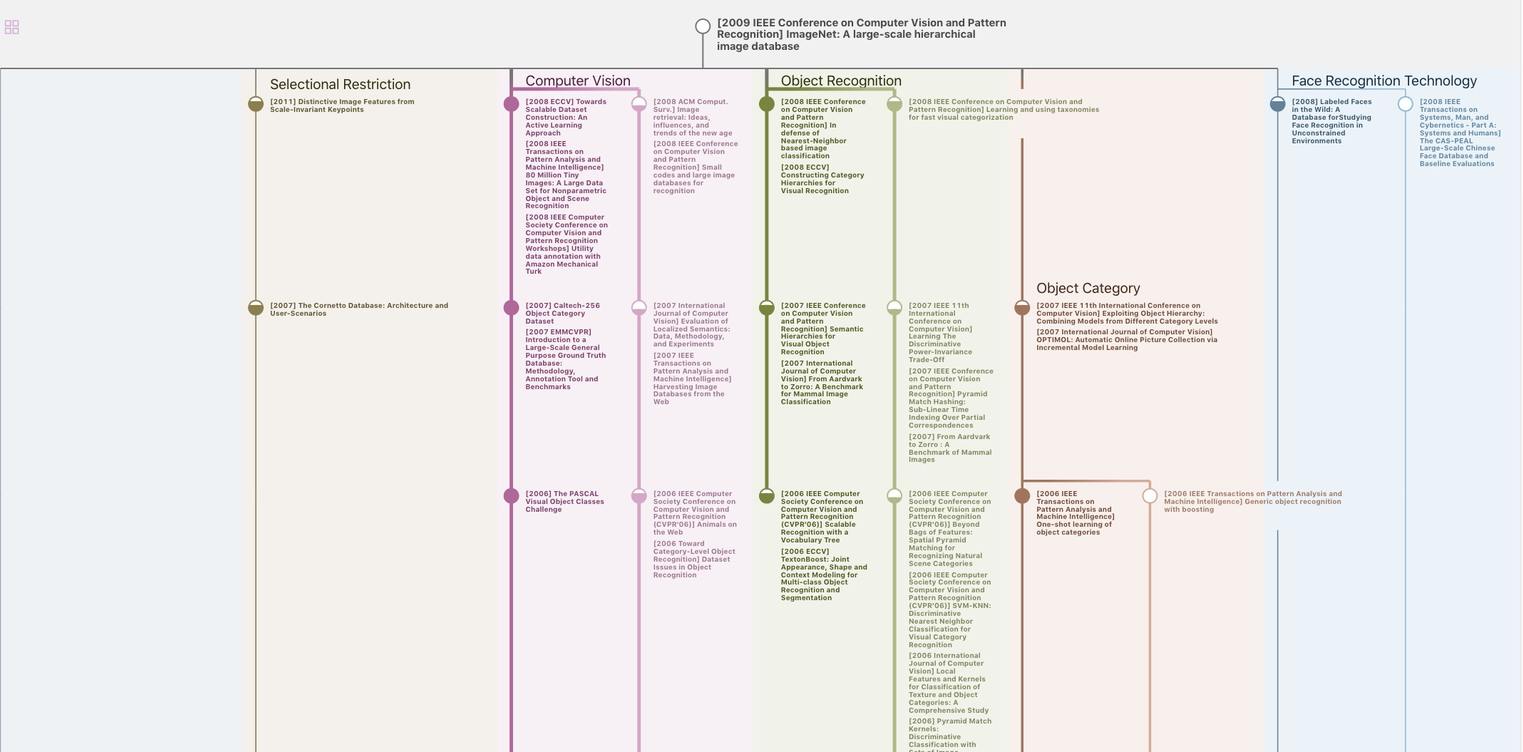
生成溯源树,研究论文发展脉络
Chat Paper
正在生成论文摘要