Preconditioned algorithm for difference of convex functions with applications to graph ginzburg-landau model
MULTISCALE MODELING & SIMULATION(2023)
摘要
In this work, we propose and study a preconditioned framework with a graphic Ginzburg-Landau functional for image segmentation and data clustering by parallel computing. Solving nonlocal models is usually challenging due to the huge computation burden. For the nonconvex and nonlocal variational functional, we propose several damped Jacobi and generalized Richardson preconditioners for the large-scale linear systems within a difference of convex functions algorithm framework. These preconditioners are efficient for parallel computing with GPU and can leverage the computational cost. Our framework also provides flexible step sizes with a global convergence guarantee. Numerical experiments show the proposed algorithms are very competitive compared to the singular value decomposition based spectral method.
更多查看译文
关键词
graph model,Ginzburg-Landau functional,difference of convex functions algo-rithms,damped Jacobi preconditioner,Richardson preconditioner,parallel computing,image seg-mentation,data clustering
AI 理解论文
溯源树
样例
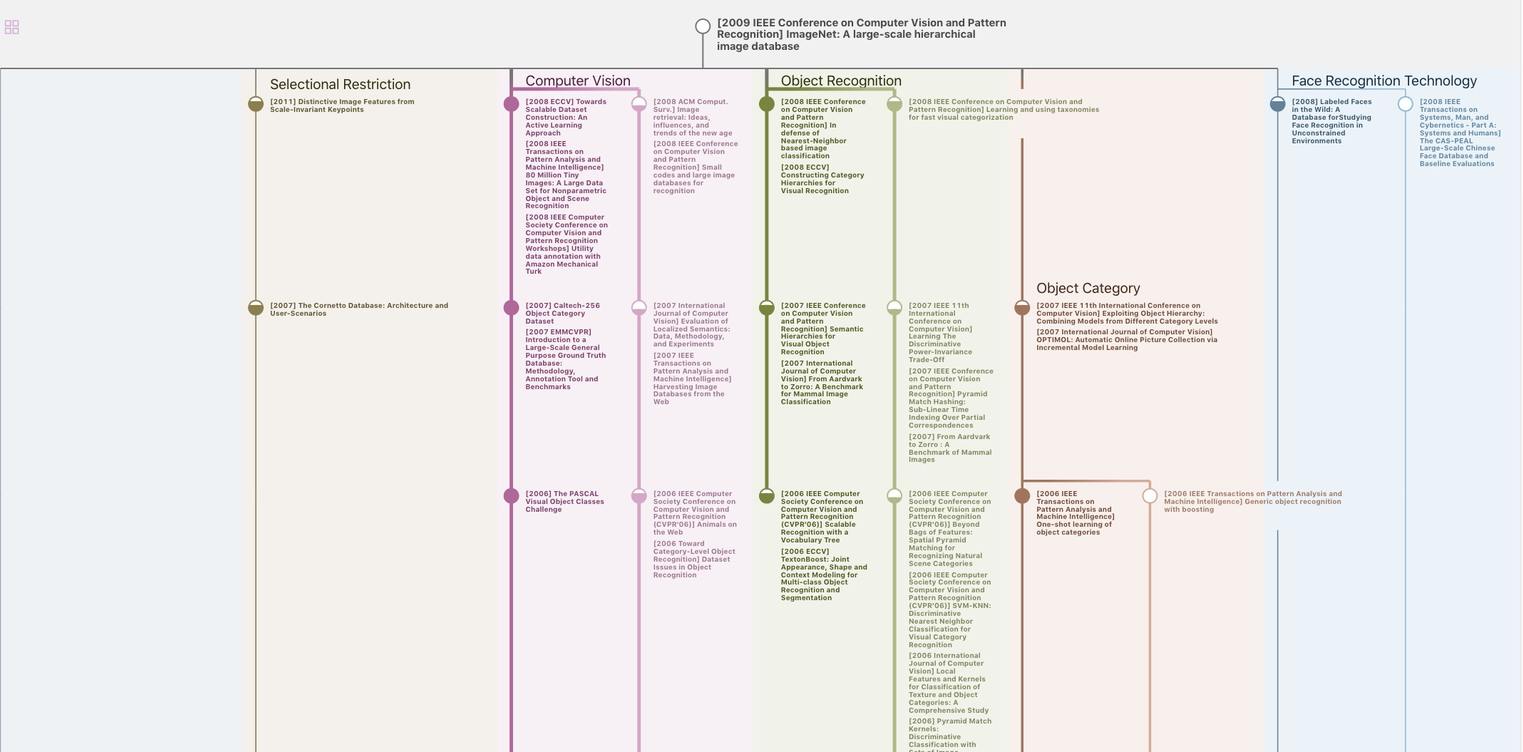
生成溯源树,研究论文发展脉络
Chat Paper
正在生成论文摘要