A sub-region Unet for weak defects segmentation with global information and mask-aware loss
Engineering Applications of Artificial Intelligence(2023)
摘要
In recent years, diverse detection methods have been proposed to achieve highly accurate image segmentation. However, low contrast and background noise interference still pose challenges to defect detection. Existing deep learning-based methods for defect detection mostly learn rich features on the whole image. This can lead to overfitting as the parameters get bigger, and it also loses the spatial connection. In order to solve this problem, we suggest a network based on spatial sub-region feature extraction and mask-aware loss that can detect metal surface defects. In this network framework, we obtain the contextual information of each region through two streams: one branch encodes the spatial sub-region information and reduces the effect of geometric and illumination deformation under u-shape architecture. A skip-connected structure in another stream is designed to learn global information and compensate for boundary features. Next, we propose a mask-aware loss to reduce background noise interference further. Finally, we validate the effectiveness of our method on two challenging datasets, reaching 88.45% and 79.16% on the NEU-Seg and USB-Seg datasets, respectively. Also, our model is only 5.48 MB, and can achieve 172 fps for processing images with 200 × 200 size defects.
更多查看译文
关键词
Surface defect segmentation,Sub-regions features,Mask-aware loss,Group convolution,Global Block,Spatial channel transform
AI 理解论文
溯源树
样例
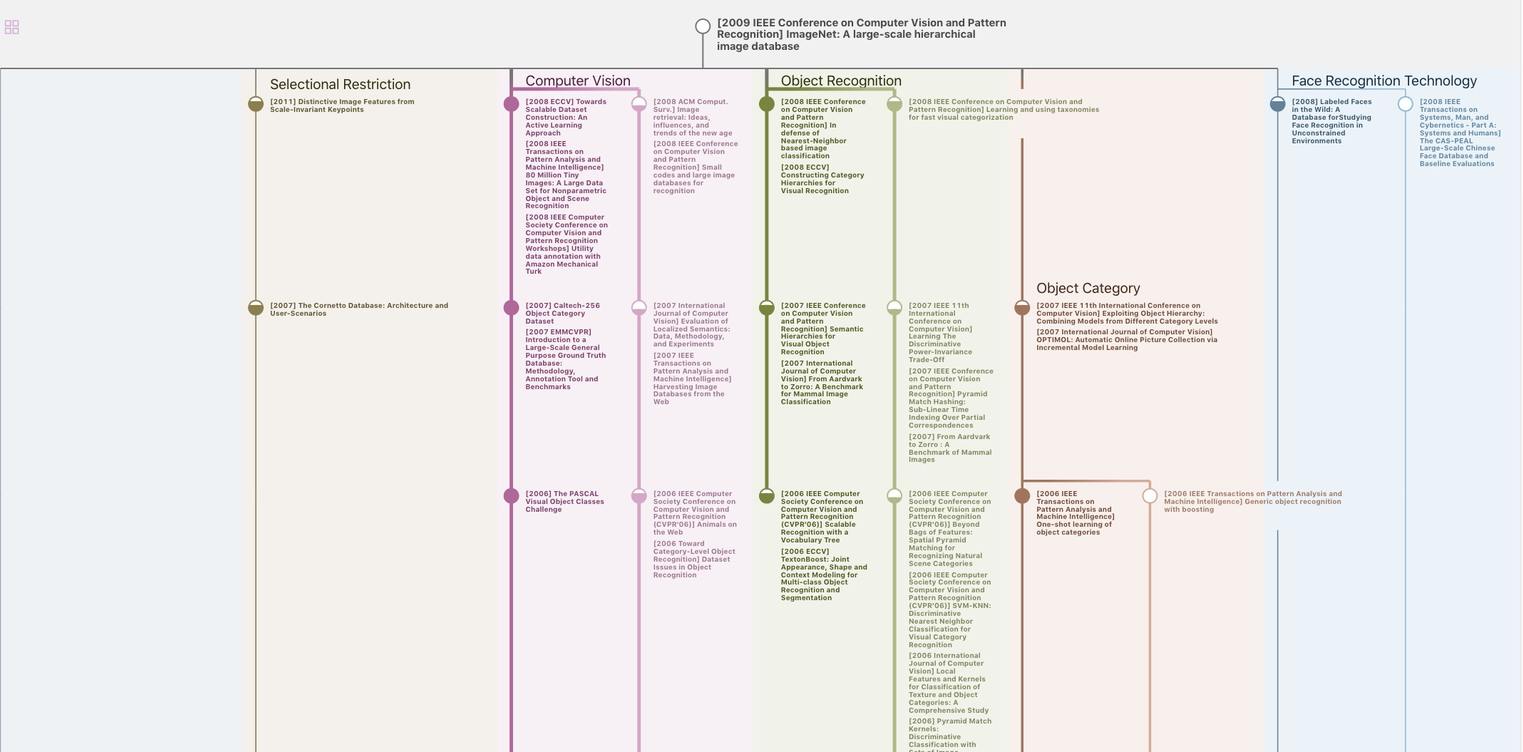
生成溯源树,研究论文发展脉络
Chat Paper
正在生成论文摘要