Deep unfolding based optimization framework of fractional programming for wireless communication systems
Wireless Networks(2023)
摘要
Multiple-ratio fractional programming (FP) has been applied to the optimization of wireless communication networks, because of the signal-to-interference-plus-noise ratio terms. However, in order to find solutions, FP requires iterative processes and may include complex operations, such as binary search, eigen-decomposition, and matrix inversion. Thus, the computational complexity and delay may violate the real-time requirements of delay-sensitive applications. To tackle this challenge, we propose a deep unfolding FP (DUFP) optimization framework naturally incorporating expert knowledge and deep neural networks. By unfolding the iterative process to neural network layers, the proposed DUFP framework trains a neural network with a little number of trainable parameters offline and then finds solutions online with reduced computational complexity. In addition, the proposed DUFP approach is applied to downlink beamforming problems to validate its efficiency. Finally, simulation results show that the proposed DUFP approach can achieve a balance between performance and computational complexity.
更多查看译文
关键词
Fractional programming,Deep unfolding,Beamforming
AI 理解论文
溯源树
样例
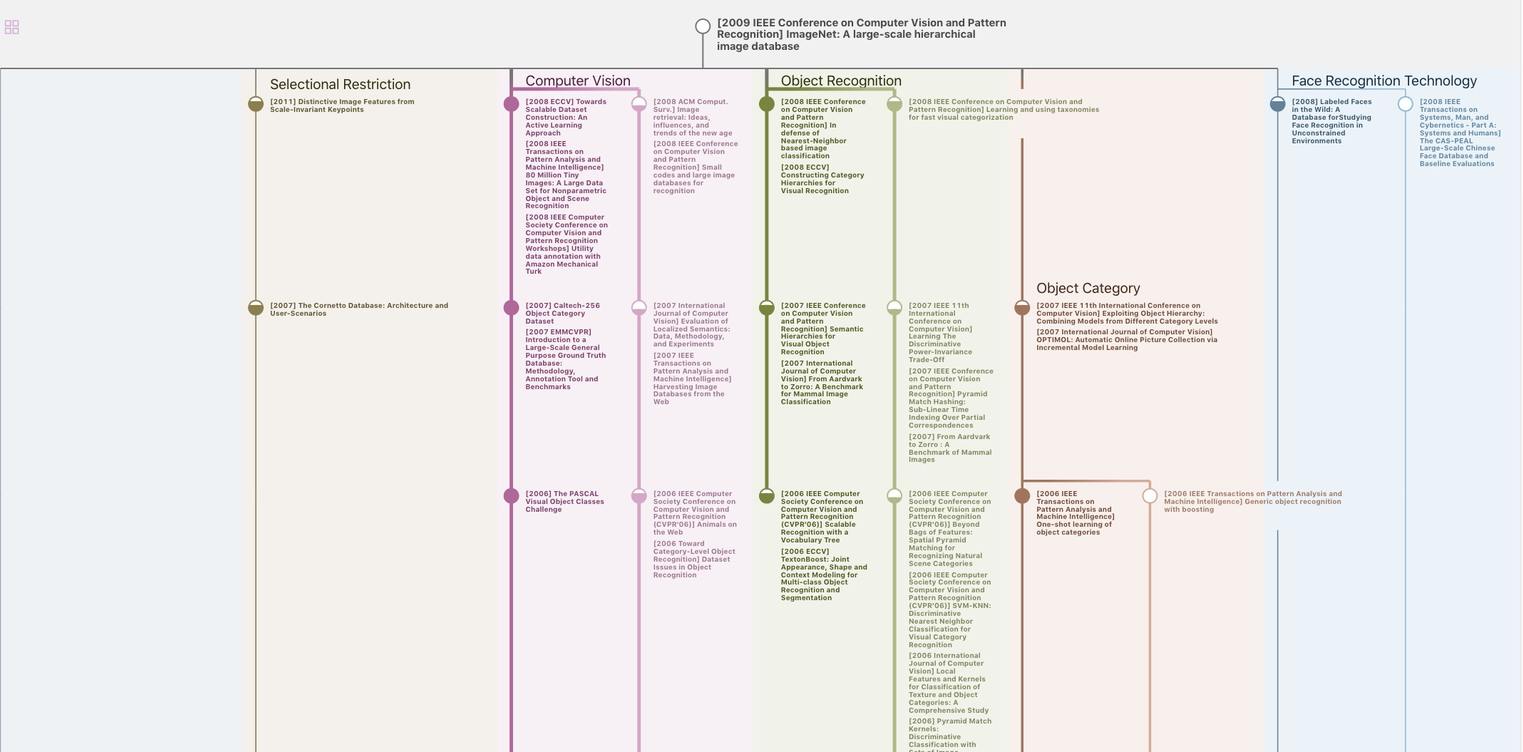
生成溯源树,研究论文发展脉络
Chat Paper
正在生成论文摘要