Inverse design of ligands using a deep generative model semi‐supervised by a data‐driven ligand field strength metric
Journal of the Chinese Chemical Society(2023)
摘要
Transition metal (TM) complexes exhibit diverse structural and electronic properties. The properties of a TM complex can be tuned by modulating the ligand field strength (LFS) inflicted by its ligands. Current quantification of the LFS of a ligand is mainly derived from experimental measurements on a subset of highly symmetrical TM complexes and is limited in ligand scope. Herein, we report a data-driven method to quantify the LFS of ligands assigned from experimental crystal structures of TM complexes. We first show that the experimental metal-ligand bond lengths of over 4,000 mononuclear Fe, Co, and Mn complexes form bimodal distributions. Using Gaussian fits on the bimodal distributions, each TM complex is assigned a spin state (SS) label. These SS labels can then be used to calculate the LFS of the ligands of the complexes. Using the obtained data-driven LFS metric, we establish that a semi-supervised deep generative model, junction tree variational autoencoder (JTVAE), can be employed to predict LFS values. Our model exhibits a mean absolute error (MAE) of 0.047 and root mean squared error of 0.072 on the training set. The model also allows the generation of novel ligands with desirable LFS values.
更多查看译文
关键词
crystal structures,data-driven discovery,deep generative model,inorganic complexes,ligand field strength,machine learning
AI 理解论文
溯源树
样例
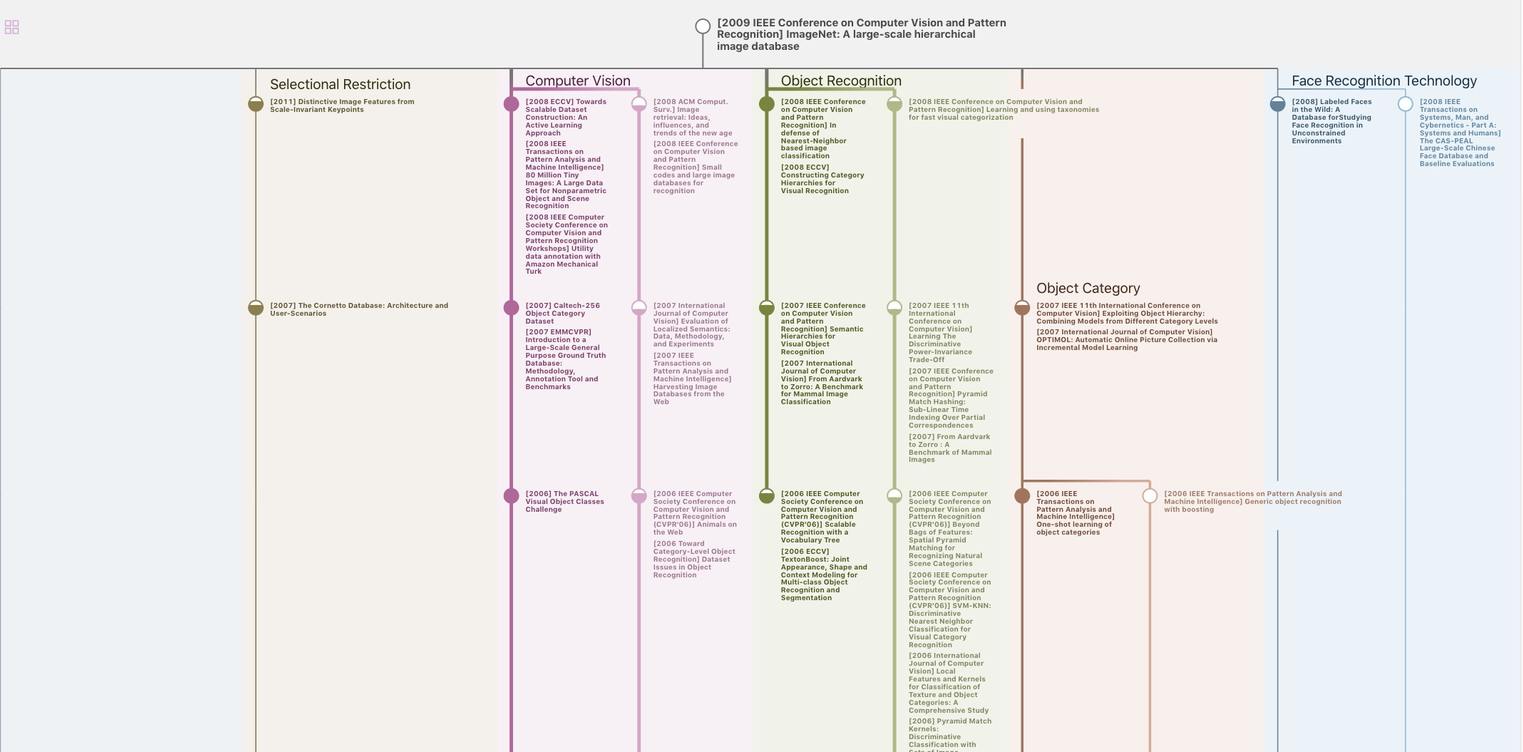
生成溯源树,研究论文发展脉络
Chat Paper
正在生成论文摘要