Local Contrastive Learning for Medical Image Recognition.
CoRR(2024)
摘要
The proliferation of Deep Learning (DL)-based methods for radiographic image analysis has created a great demand for expert-labeled radiology data. Recent self-supervised frameworks have alleviated the need for expert labeling by obtaining supervision from associated radiology reports. These frameworks, however, struggle to distinguish the subtle differences between different pathologies in medical images. Additionally, many of them do not provide interpretation between image regions and text, making it difficult for radiologists to assess model predictions. In this work, we propose Local Region Contrastive Learning (LRCLR), a flexible fine-tuning framework that adds layers for significant image region selection as well as cross-modality interaction. Our results on an external validation set of chest x-rays suggest that LRCLR identifies significant local image regions and provides meaningful interpretation against radiology text while improving zero-shot performance on several chest x-ray medical findings.
更多查看译文
关键词
local contrastive learning,recognition,medical,image
AI 理解论文
溯源树
样例
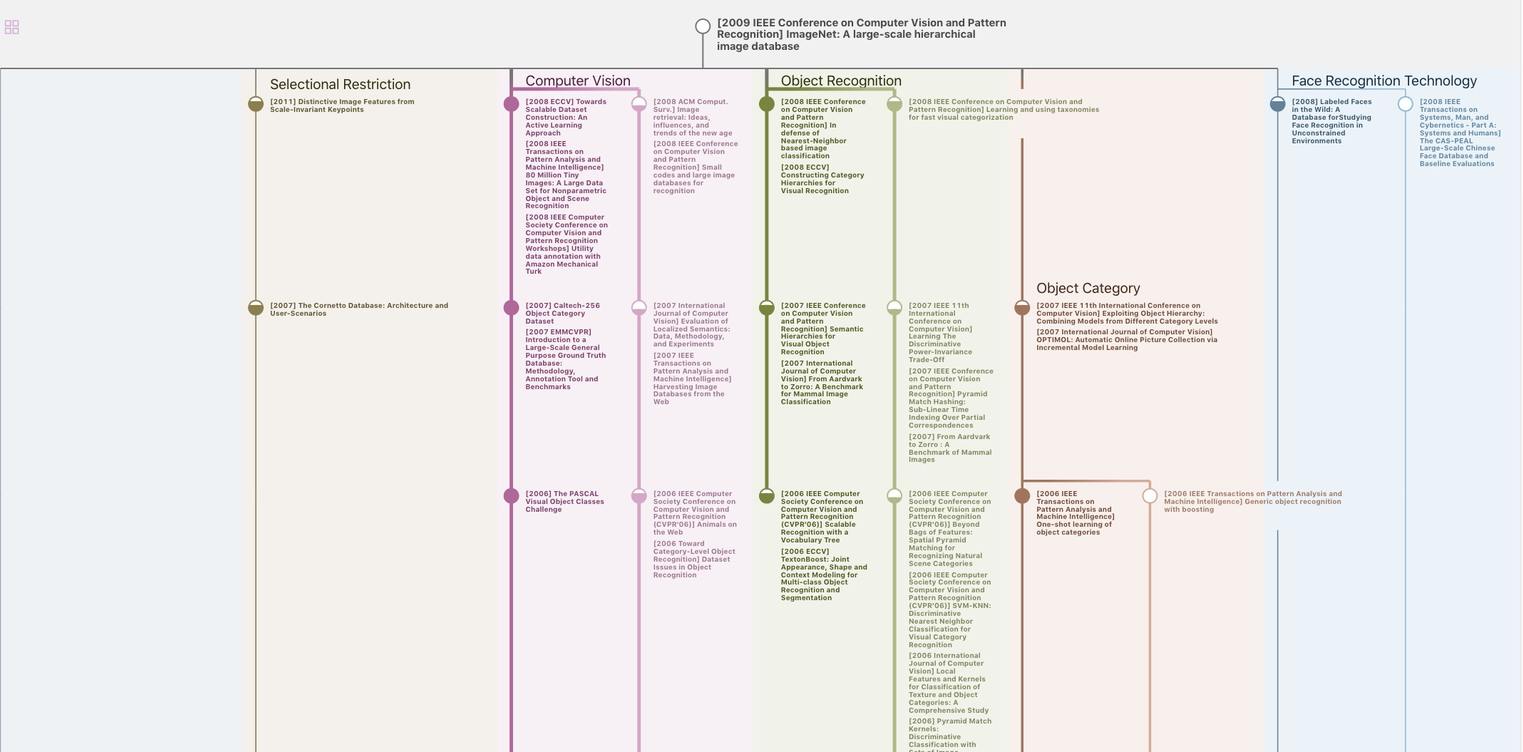
生成溯源树,研究论文发展脉络
Chat Paper
正在生成论文摘要