Optimizing the Procedure of CT Segmentation Labeling
CoRR(2023)
摘要
In Computed Tomography, machine learning is often used for automated data processing. However, increasing model complexity is accompanied by increasingly large volume datasets, which in turn increases the cost of model training. Unlike most work that mitigates this by advancing model architectures and training algorithms, we consider the annotation procedure and its effect on the model performance. We assume three main virtues of a good dataset collected for a model training to be label quality, diversity, and completeness. We compare the effects of those virtues on the model performance using open medical CT datasets and conclude, that quality is more important than diversity early during labeling; the diversity, in turn, is more important than completeness. Based on this conclusion and additional experiments, we propose a labeling procedure for the segmentation of tomographic images to minimize efforts spent on labeling while maximizing the model performance.
更多查看译文
关键词
ct segmentation labeling,procedure
AI 理解论文
溯源树
样例
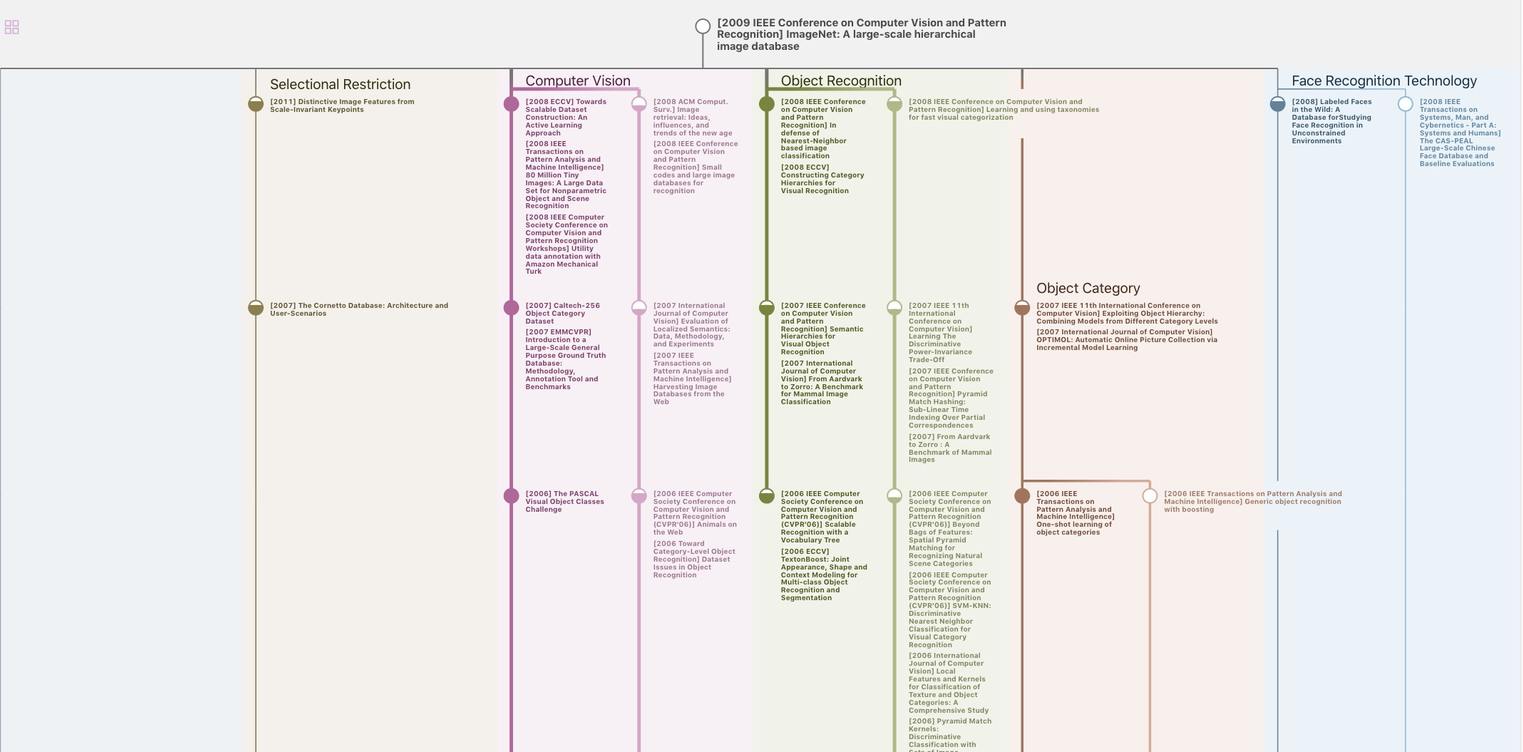
生成溯源树,研究论文发展脉络
Chat Paper
正在生成论文摘要