SEAL: Semantic Frame Execution And Localization for Perceiving Afforded Robot Actions
CoRR(2023)
摘要
Recent advances in robotic mobile manipulation have spurred the expansion of the operating environment for robots from constrained workspaces to large-scale, human environments. In order to effectively complete tasks in these spaces, robots must be able to perceive, reason, and execute over a diversity of affordances, well beyond simple pick-and-place. We posit the notion of semantic frames provides a compelling representation for robot actions that is amenable to action-focused perception, task-level reasoning, action-level execution, and integration with language. Semantic frames, a product of the linguistics community, define the necessary elements, pre- and post- conditions, and a set of sequential robot actions necessary to successfully execute an action evoked by a verb phrase. In this work, we extend the semantic frame representation for robot manipulation actions and introduce the problem of Semantic Frame Execution And Localization for Perceiving Afforded Robot Actions (SEAL) as a graphical model. For the SEAL problem, we describe our nonparametric Semantic Frame Mapping (SeFM) algorithm for maintaining belief over a finite set of semantic frames as the locations of actions afforded to the robot. We show that language models such as GPT-3 are insufficient to address generalized task execution covered by the SEAL formulation and SeFM provides robots with efficient search strategies and long term memory needed when operating in building-scale environments.
更多查看译文
关键词
semantic frame execution,actions,robot,localization
AI 理解论文
溯源树
样例
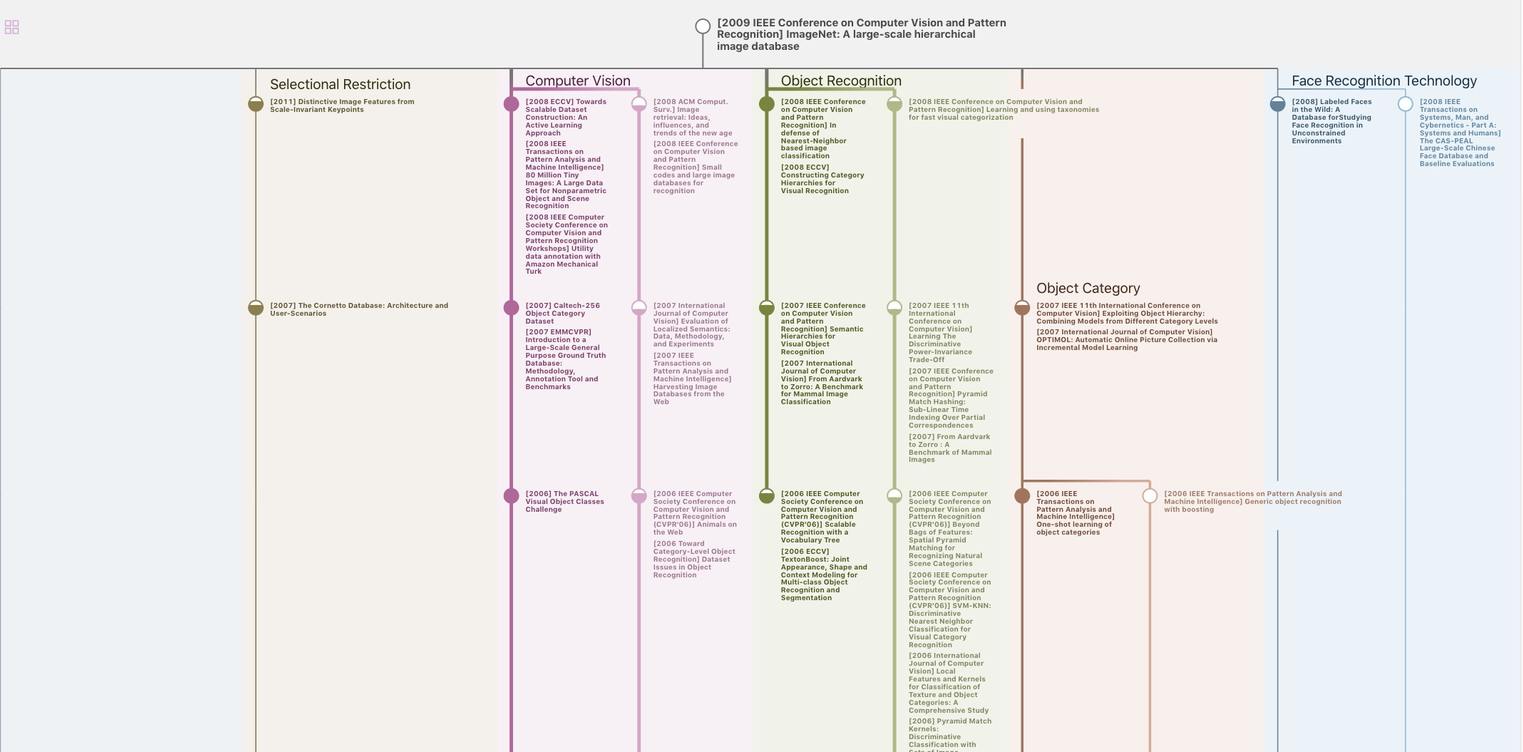
生成溯源树,研究论文发展脉络
Chat Paper
正在生成论文摘要