Femtosecond pulse parameter estimation from photoelectron momenta using machine learning
NEW JOURNAL OF PHYSICS(2023)
摘要
Deep learning models have provided huge interpretation power for image-like data. Specifically, convolutional neural networks (CNNs) have demonstrated incredible acuity for tasks such as feature extraction or parameter estimation. Here we test CNNs on strong-field ionization photoelectron spectra, training on theoretical data sets to 'invert' experimental data. Pulse characterization is used as a 'testing ground', specifically we retrieve the laser intensity, where 'traditional' measurements typically lead to 20% uncertainty. We report on crucial data augmentation techniques required to successfully train on theoretical data and return consistent results from experiments, including accounting for detector saturation. The same procedure can be repeated to apply CNNs in a range of scenarios for strong-field ionization. Using a predictive uncertainty estimation, reliable laser intensity uncertainties of a few percent can be extracted, which are consistently lower than those given by traditional techniques. Using interpretability methods can reveal parts of the distribution that are most sensitive to laser intensity, which can be directly associated with holographic interferences. The CNNs employed provide an accurate and convenient ways to extract parameters, and represent a novel interpretational tool for strong-field ionization spectra.
更多查看译文
关键词
convolutional neural networks,femtosecond pulse characterization,machine learning,strong-field ionization
AI 理解论文
溯源树
样例
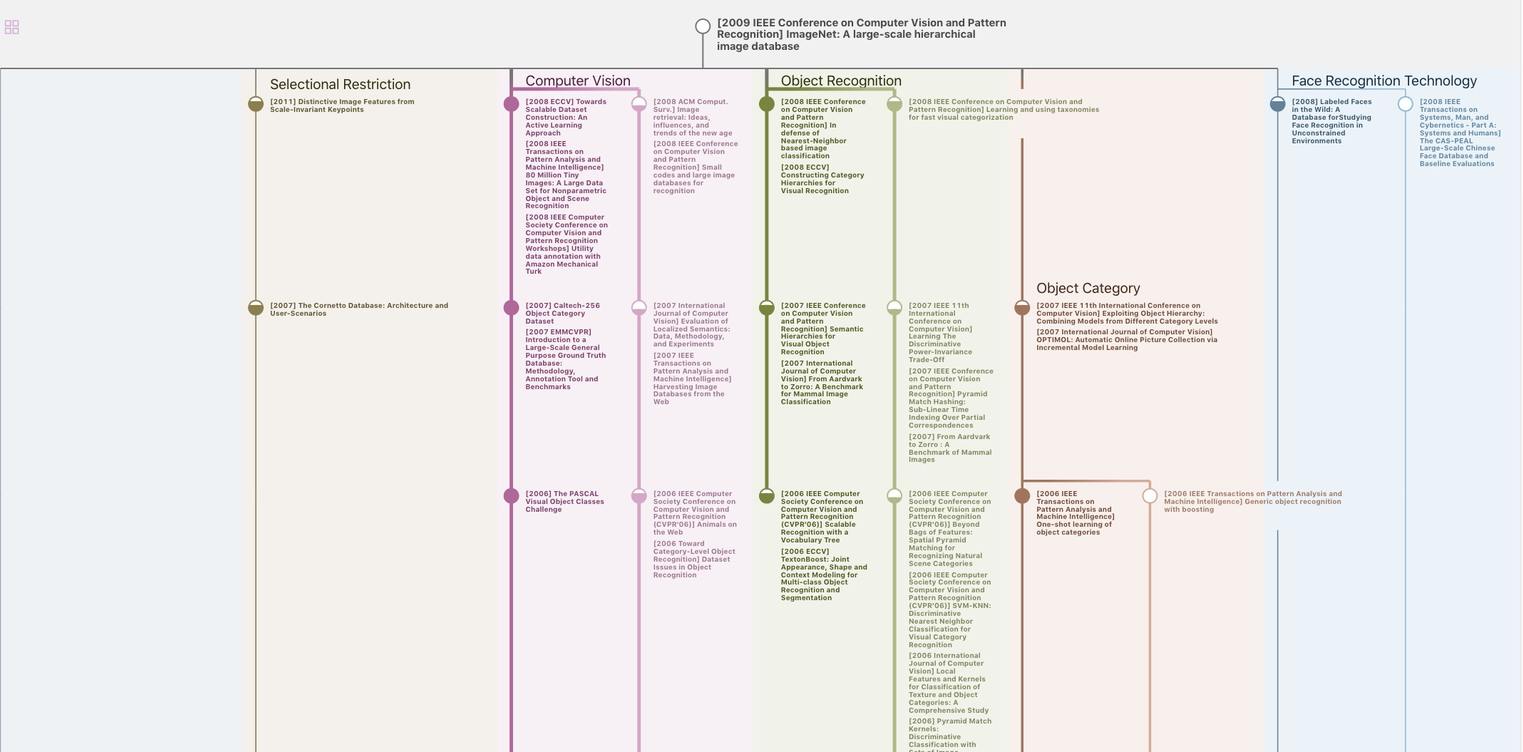
生成溯源树,研究论文发展脉络
Chat Paper
正在生成论文摘要