Remind of the Past: Incremental Learning with Analogical Prompts
CoRR(2023)
摘要
Although data-free incremental learning methods are memory-friendly, accurately estimating and counteracting representation shifts is challenging in the absence of historical data. This paper addresses this thorny problem by proposing a novel incremental learning method inspired by human analogy capabilities. Specifically, we design an analogy-making mechanism to remap the new data into the old class by prompt tuning. It mimics the feature distribution of the target old class on the old model using only samples of new classes. The learnt prompts are further used to estimate and counteract the representation shift caused by fine-tuning for the historical prototypes. The proposed method sets up new state-of-the-art performance on four incremental learning benchmarks under both the class and domain incremental learning settings. It consistently outperforms data-replay methods by only saving feature prototypes for each class. It has almost hit the empirical upper bound by joint training on the Core50 benchmark. The code will be released at \url{https://github.com/ZhihengCV/A-Prompts}.
更多查看译文
关键词
incremental learning
AI 理解论文
溯源树
样例
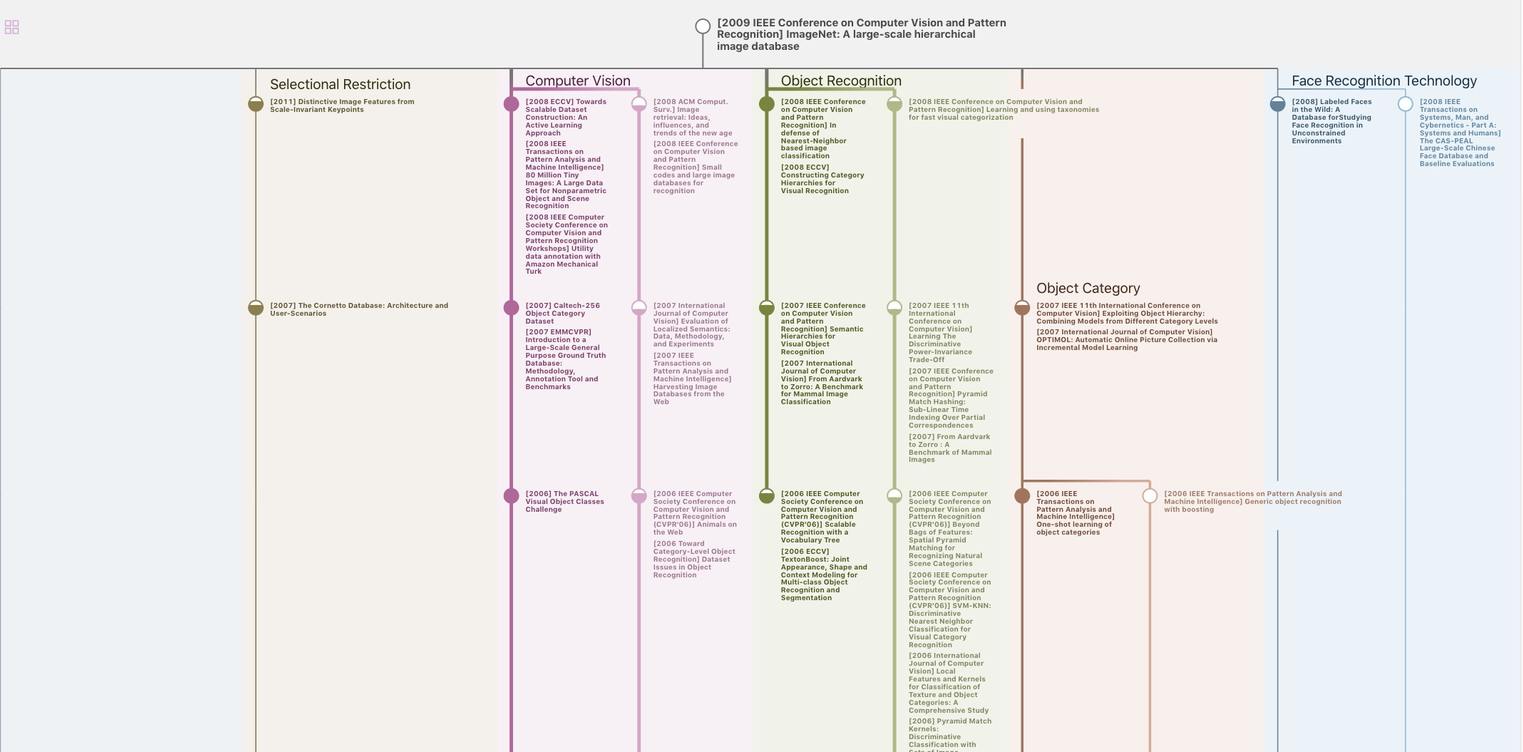
生成溯源树,研究论文发展脉络
Chat Paper
正在生成论文摘要