A Three-Player GAN for Super-Resolution in Magnetic Resonance Imaging
MACHINE LEARNING IN CLINICAL NEUROIMAGING, MLCN 2023(2023)
Abstract
Learning based single image super resolution (SISR) is well investigated in 2D images. However, SISR for 3D magnetic resonance images (MRI) is more challenging compared to 2D, mainly due to the increased number of neural network parameters, the larger memory requirement, and the limited amount of available training data. Current SISR methods for 3D volumetric images are based on generative adversarial networks (GANs), especially Wasserstein GANs. Other common architectures in the 2D domain, e.g. transformer models, require large amounts of training data and are therefore not suitable for the limited 3D data. As a popular GAN variant, Wasserstein GANs sometimes produce blurry results as they may not converge to a global (or local) optimum. In this paper, we propose a new method for 3D SISR using a GAN framework. Our approach incorporates instance noise to improve the training stability of the GAN, as well as a relativistic GAN loss function and an updating feature extractor as the third player during the training process. Our experiments demonstrate that our method produces highly accurate results using very few training samples. Specifically, we show that we need less than 30 training samples, which is a significant reduction compared to the thousands typically required in previous studies. Moreover, our method improves out-of-sample results, demonstrating its effectiveness. The code is available in https://github. com/wqlevi/threeplayerGANSR.
MoreTranslated text
Key words
Super-resolution,Brain MRI,GAN
AI Read Science
Must-Reading Tree
Example
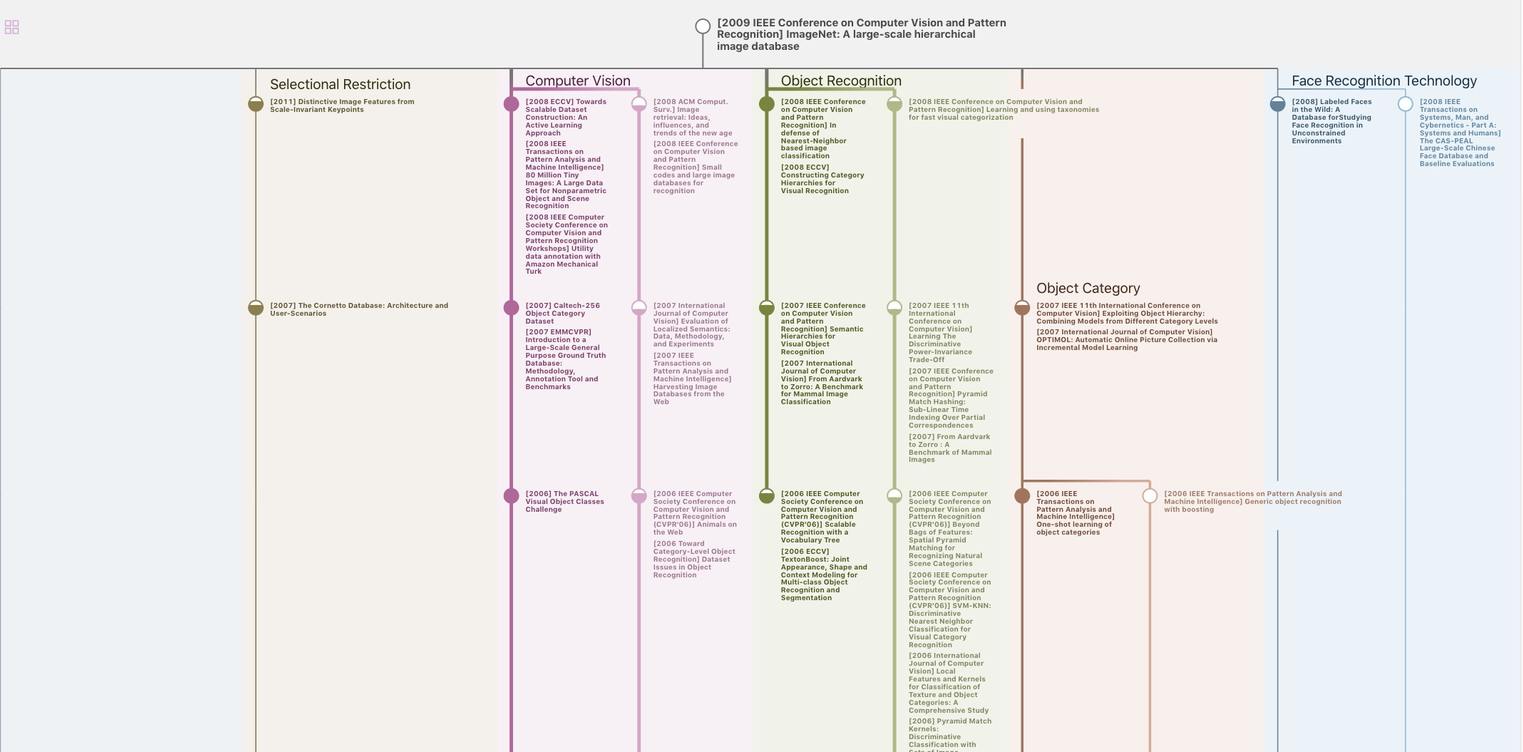
Generate MRT to find the research sequence of this paper
Chat Paper
Summary is being generated by the instructions you defined