Hard Sample Matters a Lot in Zero-Shot Quantization
CVPR 2023(2023)
摘要
Zero-shot quantization (ZSQ) is promising for compressing and accelerating deep neural networks when the data for training full-precision models are inaccessible. In ZSQ, network quantization is performed using synthetic samples, thus, the performance of quantized models depends heavily on the quality of synthetic samples. Nonetheless, we find that the synthetic samples constructed in existing ZSQ methods can be easily fitted by models. Accordingly, quantized models obtained by these methods suffer from significant performance degradation on hard samples. To address this issue, we propose HArd sample Synthesizing and Training (HAST). Specifically, HAST pays more attention to hard samples when synthesizing samples and makes synthetic samples hard to fit when training quantized models. HAST aligns features extracted by full-precision and quantized models to ensure the similarity between features extracted by these two models. Extensive experiments show that HAST significantly outperforms existing ZSQ methods, achieving performance comparable to models that are quantized with real data.
更多查看译文
关键词
Transfer,meta,low-shot,continual,or long-tail learning
AI 理解论文
溯源树
样例
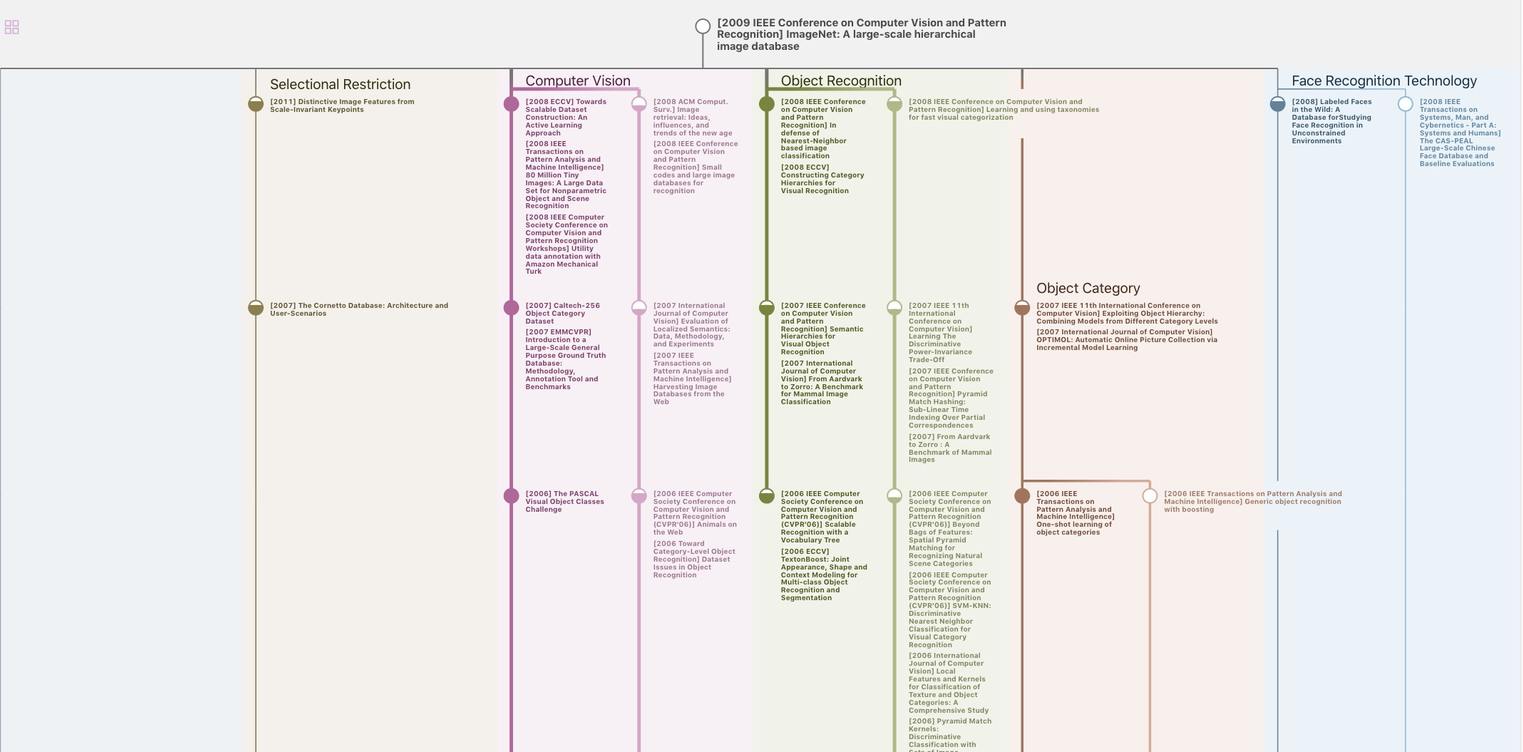
生成溯源树,研究论文发展脉络
Chat Paper
正在生成论文摘要