Enhancing Embedding Representations of Biomedical Data using Logic Knowledge
CoRR(2023)
摘要
Knowledge Graph Embeddings (KGE) have become a quite popular class of models specifically devised to deal with ontologies and graph structure data, as they can implicitly encode statistical dependencies between entities and relations in a latent space. KGE techniques are particularly effective for the biomedical domain, where it is quite common to deal with large knowledge graphs underlying complex interactions between biological and chemical objects. Recently in the literature, the PharmKG dataset has been proposed as one of the most challenging knowledge graph biomedical benchmark, with hundreds of thousands of relational facts between genes, diseases and chemicals. Despite KGEs can scale to very large relational domains, they generally fail at representing more complex relational dependencies between facts, like logic rules, which may be fundamental in complex experimental settings. In this paper, we exploit logic rules to enhance the embedding representations of KGEs on the PharmKG dataset. To this end, we adopt Relational Reasoning Network (R2N), a recently proposed neural-symbolic approach showing promising results on knowledge graph completion tasks. An R2N uses the available logic rules to build a neural architecture that reasons over KGE latent representations. In the experiments, we show that our approach is able to significantly improve the current state-of-the-art on the PharmKG dataset. Finally, we provide an ablation study to experimentally compare the effect of alternative sets of rules according to different selection criteria and varying the number of considered rules.
更多查看译文
关键词
available logic rules,biological objects,biomedical data,biomedical domain,challenging knowledge graph biomedical benchmark,chemical objects,complex experimental settings,complex interactions,complex relational dependencies,considered rules,diseases,embedding representations,graph structure data,KGE latent representations,KGE techniques,knowledge graph completion tasks,Knowledge Graph Embeddings,knowledge graphs,latent space,logic knowledge,neural-symbolic approach,PharmKG dataset,relational domains,relational facts,Relational Reasoning Network,statistical dependencies
AI 理解论文
溯源树
样例
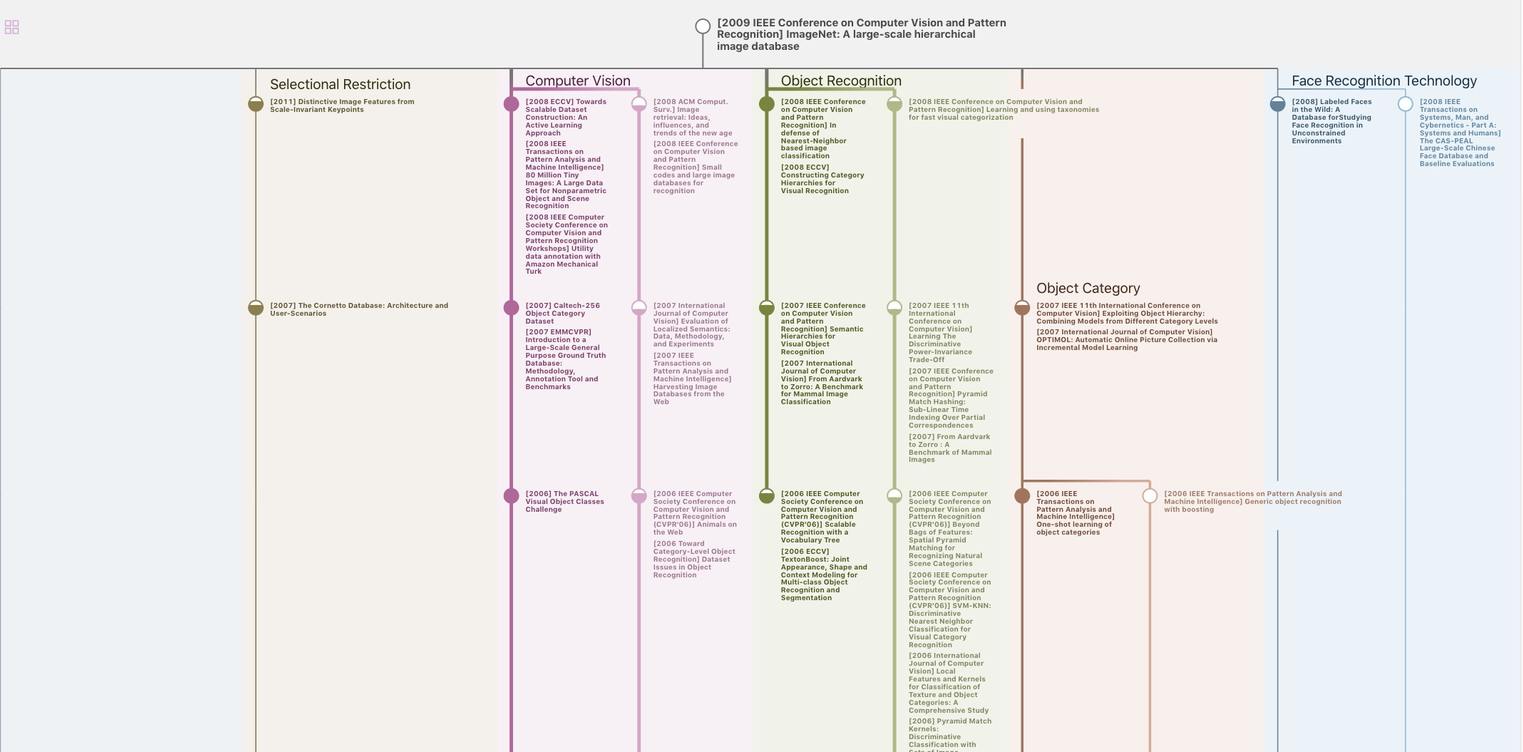
生成溯源树,研究论文发展脉络
Chat Paper
正在生成论文摘要