ProtoCon: Pseudo-Label Refinement via Online Clustering and Prototypical Consistency for Efficient Semi-Supervised Learning
CVPR 2023(2023)
摘要
Confidence-based pseudo-labeling is among the dominant approaches in semi-supervised learning (SSL). It relies on including high-confidence predictions made on unlabeled data as additional targets to train the model. We propose ProtoCon, a novel SSL method aimed at the less-explored label-scarce SSL where such methods usually underperform. ProtoCon refines the pseudo-labels by leveraging their nearest neighbours' information. The neighbours are identified as the training proceeds using an online clustering approach operating in an embedding space trained via a prototypical loss to encourage well-formed clusters. The online nature of ProtoCon allows it to utilise the label history of the entire dataset in one training cycle to refine labels in the following cycle without the need to store image embeddings. Hence, it can seamlessly scale to larger datasets at a low cost. Finally, ProtoCon addresses the poor training signal in the initial phase of training (due to fewer confident predictions) by introducing an auxiliary self-supervised loss. It delivers significant gains and faster convergence over state-of-the-art across 5 datasets, including CIFARs, ImageNet and DomainNet.
更多查看译文
关键词
online clustering,pseudo-label,semi-supervised
AI 理解论文
溯源树
样例
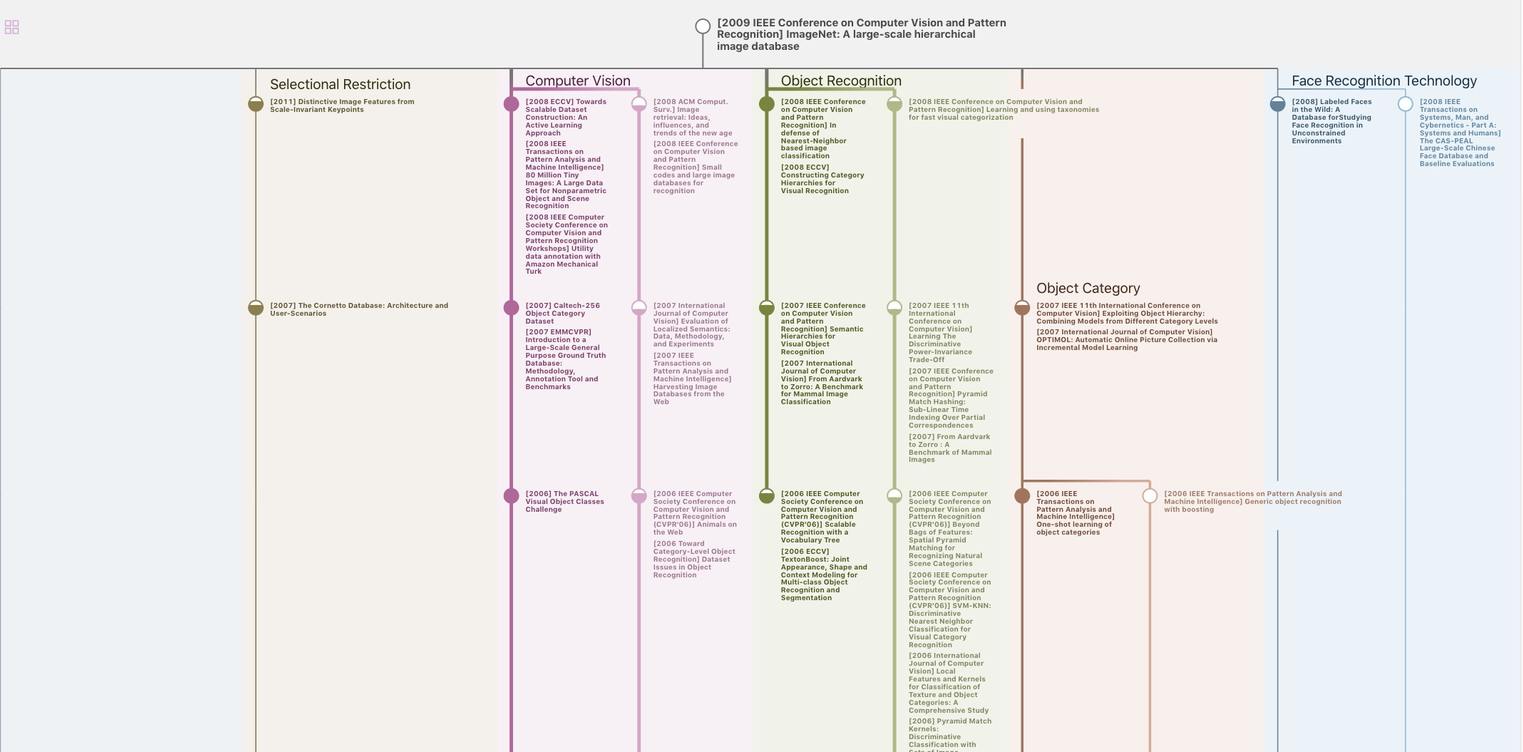
生成溯源树,研究论文发展脉络
Chat Paper
正在生成论文摘要