Hybrid physics-BP neural network-based strength degradation model of corroded reinforcements under the simulated colliery environment
Structures(2023)
摘要
This study proposes a hybrid physics-artificial neural network (ANN)-based framework for developing a physics-based strength degradation model of corroded reinforcements taken from deteriorating reinforced concrete (RC) beams respective under a single harsh colliery environment and coupled action of corrosive environment and sustained load. This proposed degradation model can well account for the impacts of random variables (e.g., the diameter of reinforcing steel bars) on the strength degradation behavior of corroded reinforcements. The main contributions of this study include the following aspects: Firstly, based on the experimental results, a BP-ANN surrogate model is established to substitute the experiment method for generating the residual yield and ultimate strengths of corroded reinforcements under different exposure conditions. In addition, a new data sample is formed with the experimental and simulated data obtained by this trained surrogate model. Applying the Bayes theorem, the physics-based strength degradation model respecting to corrosion degree and diameter of corroding reinforcements is constructed. The posterior probability distributions of unknown model parameters are developed, which is beneficial for representing the epistemic uncertainty in assessing the service reliability of deteriorating RC components and structures. Finally, a sample from experimental data is randomly selected to verify the accuracy of this physics-based strength degradation model. Analytical results indicate that the diameter of reinforcements as well as the coupled action of corrosive simulated colliery environment and sustained load have considerable influences on the strength degradation of corroded reinforcing steels. This study provides an effective approach to developing a physics-based strength degradation model of corroding reinforcements and highly improves the use efficiency of experimental results.
更多查看译文
关键词
Bayes theorem,ANN,Colliery environment,Surrogate model,Strength degradation model
AI 理解论文
溯源树
样例
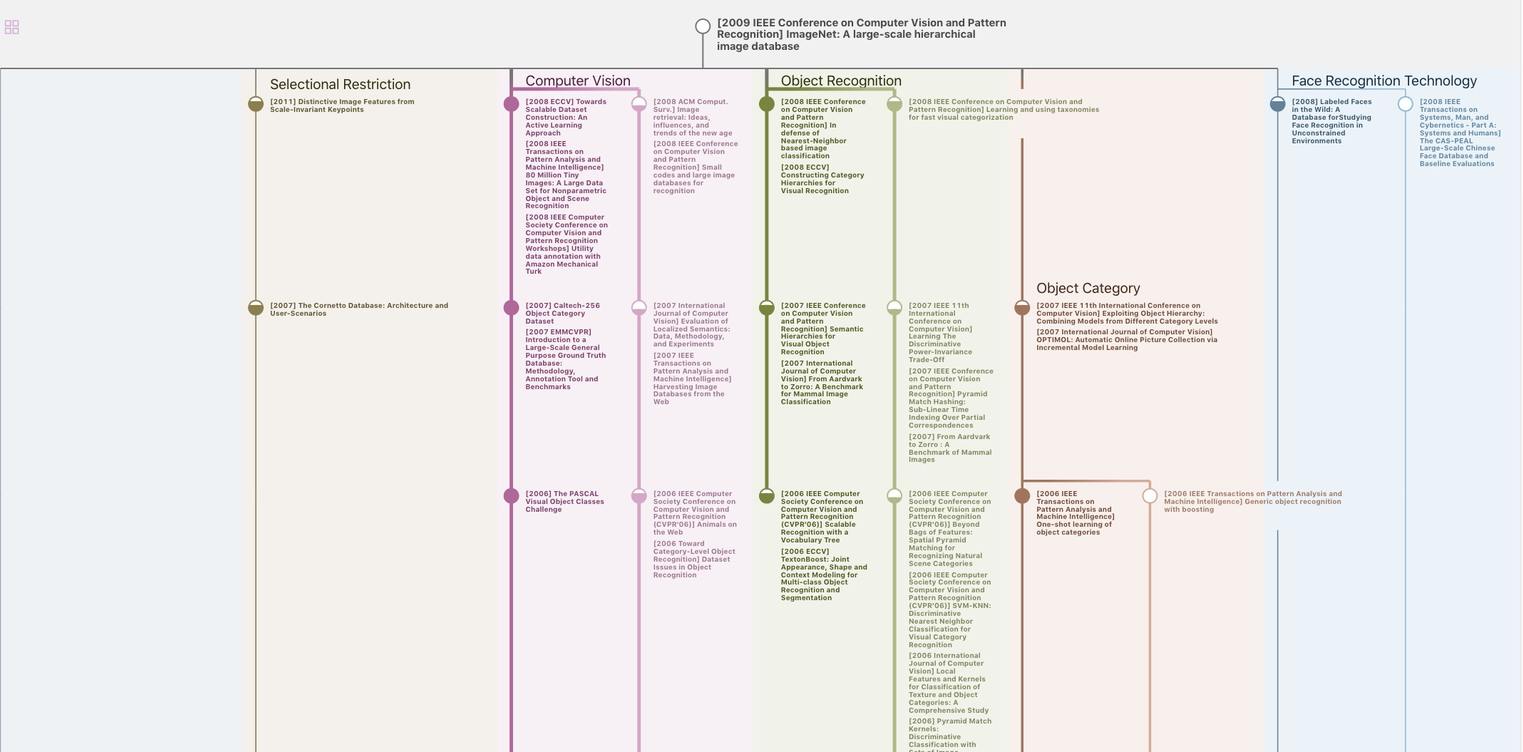
生成溯源树,研究论文发展脉络
Chat Paper
正在生成论文摘要