An explainable deep learning classifier of bovine mastitis based on whole genome sequence data - circumventing the p>>>n problem
International Journal of Molecular Sciences(2023)
Abstract
The most serious drawback underlying the biological annotation of Whole Genome Sequence data is the p>>n problem, meaning that the number of polymorphic variants (p) is much larger than the number of available phenotypic records (n). Therefore, the major aim of the study was to propose a way to circumvent the problem by combining a LASSO logistic regression model with Deep Learning (DL). That was illustrated by a practical biological problem of classification of cows into mastitis-susceptible or mastitis-resistant, based on genotypes of Single Nucleotide Polymorphisms (SNPs) identified in their WGS. Among several DL architectures proposed via optimisation of DL hyperparameters using the Optuna software, imposed on different SNP sub-sets defined by LASSO logistic regressions with different penalty values, the architecture with 204,642 SNPs was selected as the best one. This architecture was composed of 2 layers with respectively 7 and 46 units per layer as well as respective drop-out rates of 0.210 and 0.358. The classification of the test data set resulted in the AUC=0.750, accuracy=0.650, sensitivity=0.600, and specificity=0.700 was selected as the best model and thus proceeded to genomic and functional annotations. Significant SNPs were selected based on the SHapley Additive exPlanation values transformed to Z-scores to assess the underlying type I-error. These SNPs were annotated to genes. As a final result, a single GO term related to the biological process and thirteen GO terms related to the molecular function were significantly enriched in the gene set that corresponded to the significant SNPs.
Author Summary Our objective is to distinguish between cows that are susceptible and resistant to bovine mastitis by analysing their genomic data. However, we face a significant challenge due to the large number of single nucleotide polymorphisms (SNPs) and limited sample size. To address this challenge, we utilize two methods: feature selection algorithms and deep learning. We experiment with various ways of implementing these techniques and evaluate their performance on a validation set. Our findings reveal that the optimal approach can accurately predict a cow’s susceptibility or resistance status around 65% of the time. Additionally, we employ a technique to identify the most crucial SNPs and their biological functions. Our results indicate that some of these SNPs are related to immune response or protein synthesis pathways, implying that they may affect the cow’s health and productivity.
### Competing Interest Statement
The authors have declared no competing interest.
MoreTranslated text
AI Read Science
Must-Reading Tree
Example
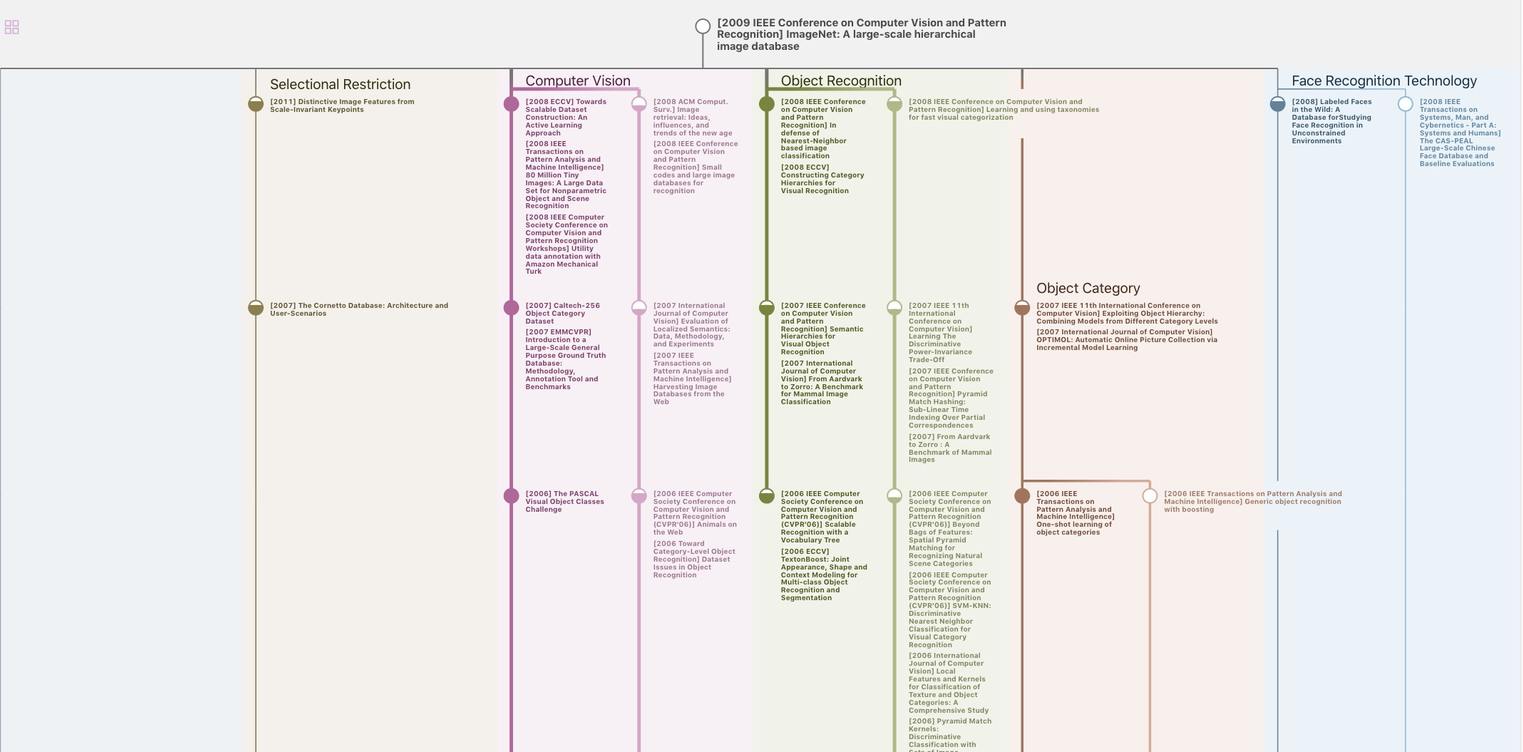
Generate MRT to find the research sequence of this paper
Chat Paper
Summary is being generated by the instructions you defined