A Lightweight Deep Learning Framework for Botnet Detecting at the IoT Edge
Computers & Security(2023)
摘要
Nowadays, a large number of Internet-of-Things (IoT) devices are exposed on the Internet. Due to the se-rious security flaws and users' misuse, they are vulnerable to various attacks, such as botnet, a major risk for IoT security. Monitoring network traffic at the IoT edge and identifying botnet activities in early-stage becomes increasingly critical. With the powerful capability of learning complex patterns, many studies pay attention to neural networks for solving this problem. However, traditional network intrusion detec-tion systems (NIDS) based on neural networks have a high resource consumption and aren't suitable for deploying in Internet gateways and routers. In this paper, we propose a novel lightweight and generic NIDS with a two-stage framework to detect botnet activities on the IoT network, only using accessible packet-length features. Our NIDS can be deployed at a resource-limited device and work in an efficient online manner. We first propose 21 statistical features which are discriminative for malicious and nor-mal traffic flows. Based on these features, we design a module based on an autoencoder to filter out a large number of normal traffic flows in the first stage. Then we propose a novel mechanism to transform the packet length sequence into a three-channel RGB image for malicious traffic classification based on a lightweight convolutional neural network (CNN). In order to demonstrate the performance, we build a real IoT environment and deploy our NIDS on a Raspberry PI. The experiment results show that our NIDS has excellent accuracy for detecting botnet activities and higher processing rate for traffic compared with the state-of-the-art ANN-based methods.(c) 2023 Elsevier Ltd. All rights reserved.
更多查看译文
关键词
Network intrusion detection, Internet-of-Things, Botnet detection, Machine learning, Deep Learning
AI 理解论文
溯源树
样例
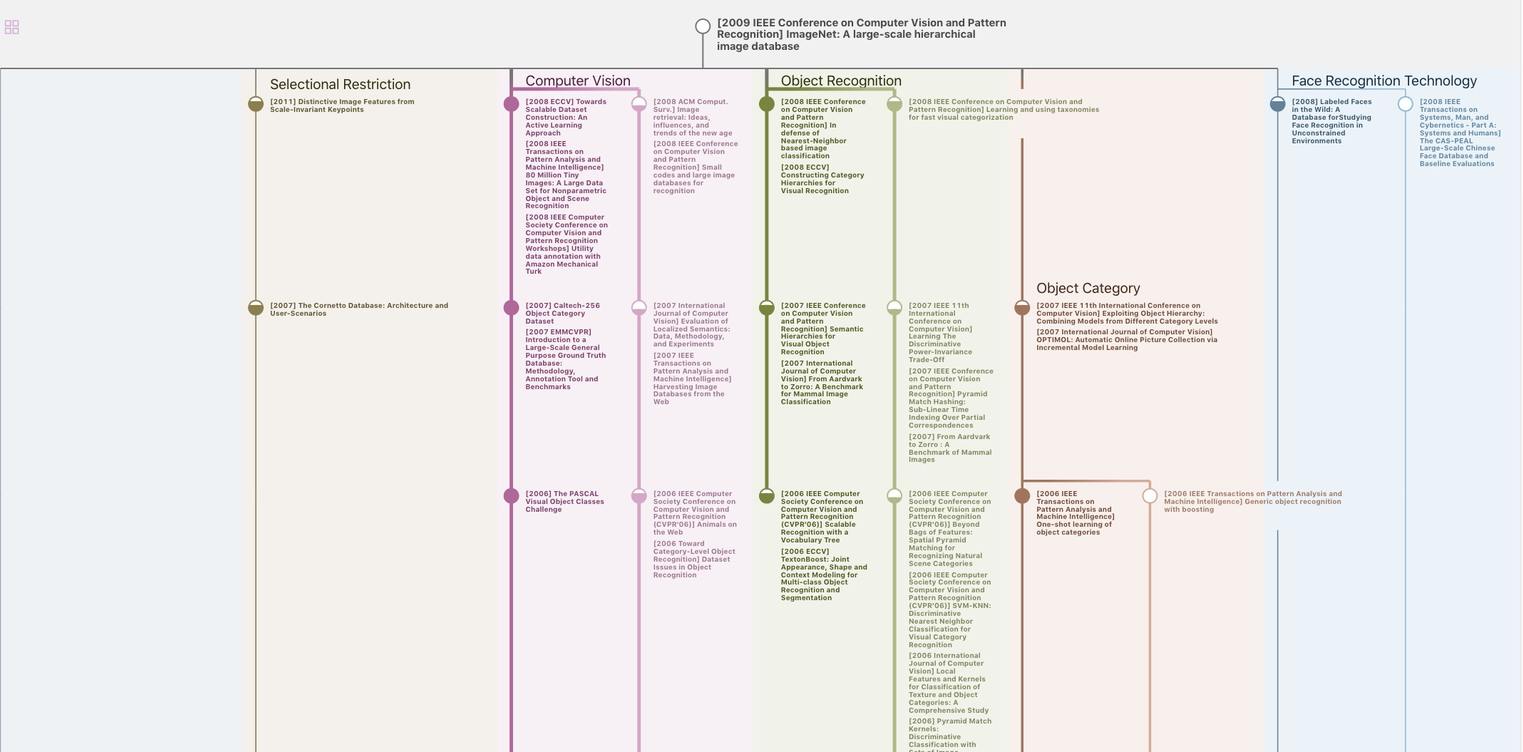
生成溯源树,研究论文发展脉络
Chat Paper
正在生成论文摘要