EGGNet, a generalizable geometric deep learning framework for protein complex pose scoring
ACS Omega(2023)
Abstract
Computational prediction of molecule-protein interactions has been key for developing new molecules to interact with a target protein for therapeutics development. Past work includes two independent streams of approaches: (1) predicting protein-protein interactions (PPI) between naturally occurring proteins and (2) predicting the binding affinities between proteins and small molecule ligands (aka drug target interaction, or DTI). Studying the two problems in isolation has limited the ability of these computational models to generalize across the PPI and DTI tasks, both of which ultimately involve non-covalent interactions with a protein target. In this work, we developed an Equivariant Graph of Graphs neural Network (EGGNet), a geometric deep learning framework for molecule-protein binding predictions that can handle three types of molecules for interacting with a target protein: (1) small molecules, (2) synthetic peptides and (3) natural proteins. EGGNet leverages a graph of graphs (GoGs) representation constructed from the molecule structures at atomic-resolution and utilizes a multi-resolution equivariant graph neural network (GNN) to learn from such representations. In addition, EGGNet leverages the underlying biophysics and makes use of both atom- and residue-level interactions, which improve EGGNet’s ability to rank candidate poses from blind docking. EGGNet achieves competitive performance on both a public proteinsmall molecule binding affinity prediction task (80.2% top-1 success rate on CASF-2016) and an synthetic protein interface prediction task (88.4% AUPR). We envision that the proposed geometric deep learning framework can generalize to many other protein interaction prediction problems, such as binding site prediction and molecular docking, helping accelerate protein engineering and structure-based drug development.
### Competing Interest Statement
The authors have declared no competing interest.
MoreTranslated text
AI Read Science
Must-Reading Tree
Example
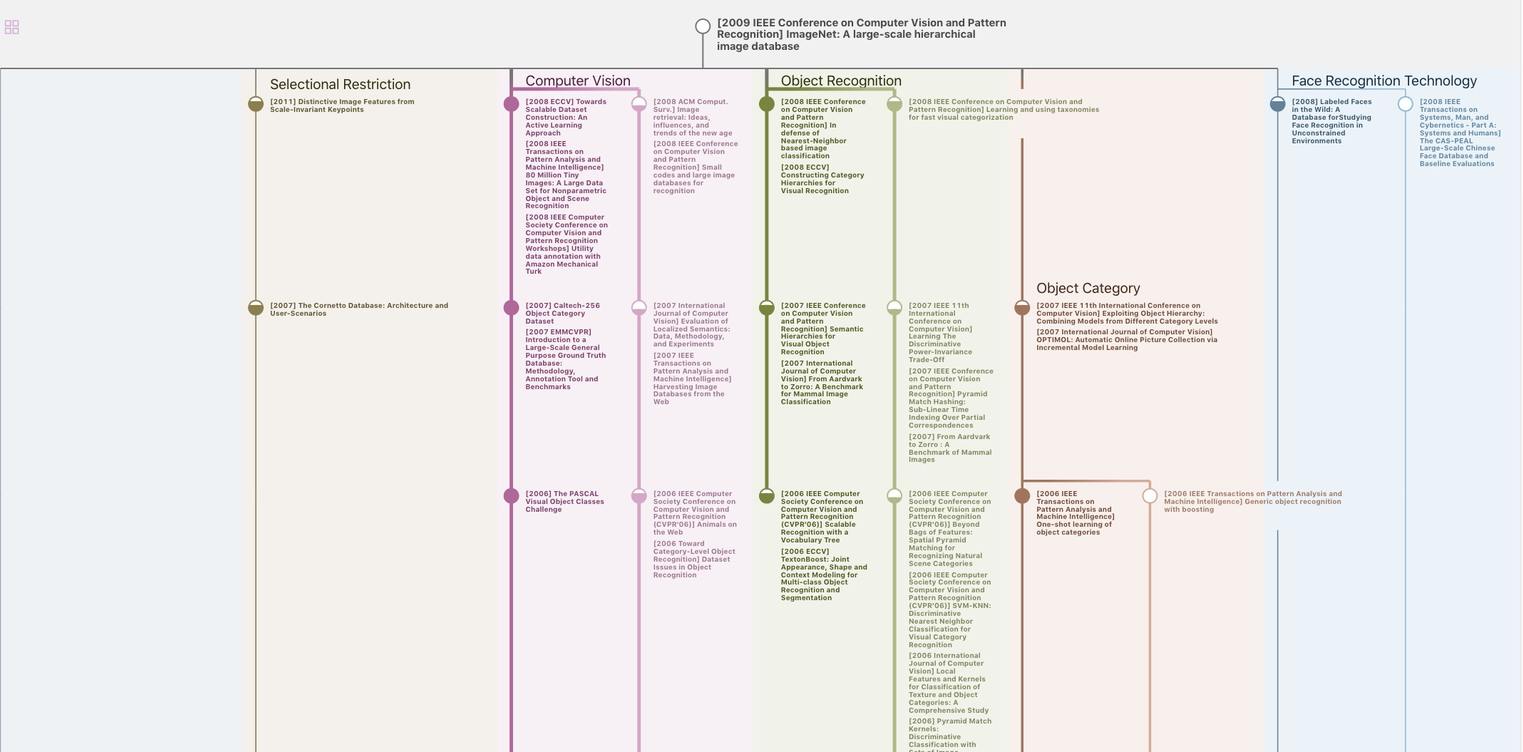
Generate MRT to find the research sequence of this paper
Chat Paper
Summary is being generated by the instructions you defined