Adaptive Endpointing with Deep Contextual Multi-armed Bandits
arxiv(2023)
摘要
Current endpointing (EP) solutions learn in a supervised framework, which does not allow the model to incorporate feedback and improve in an online setting. Also, it is a common practice to utilize costly grid-search to find the best configuration for an endpointing model. In this paper, we aim to provide a solution for adaptive endpointing by proposing an efficient method for choosing an optimal endpointing configuration given utterance-level audio features in an online setting, while avoiding hyperparameter grid-search. Our method does not require ground truth labels, and only uses online learning from reward signals without requiring annotated labels. Specifically, we propose a deep contextual multi-armed bandit-based approach, which combines the representational power of neural networks with the action exploration behavior of Thompson modeling algorithms. We compare our approach to several baselines, and show that our deep bandit models also succeed in reducing early cutoff errors while maintaining low latency.
更多查看译文
关键词
endpointing,multi-armed bandits,automatic speech recognition,turn taking,dialog modeling
AI 理解论文
溯源树
样例
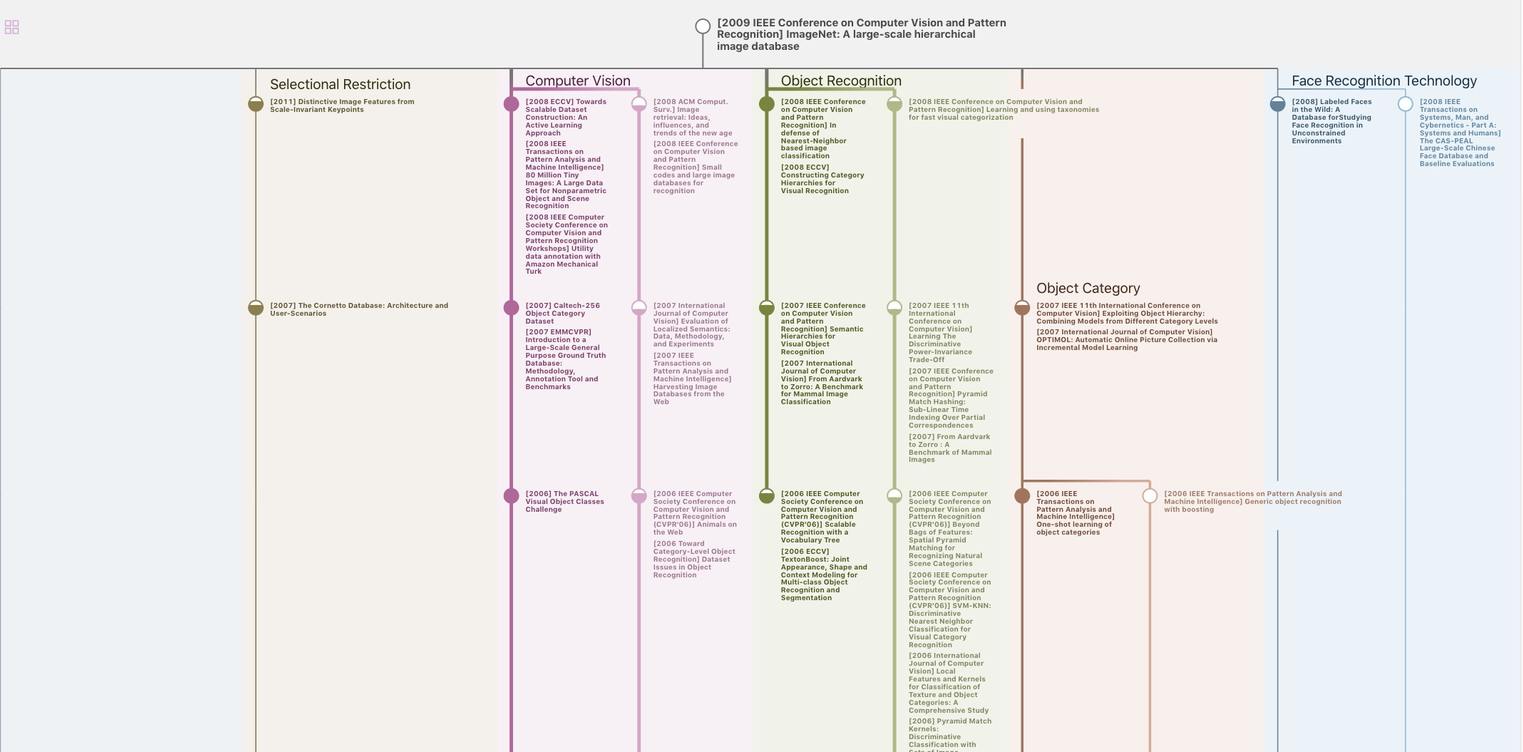
生成溯源树,研究论文发展脉络
Chat Paper
正在生成论文摘要