Deep Generative Multi-Agent Imitation Model as a Computational Benchmark for Evaluating Human Performance in Complex Interactive Tasks: A Case Study in Football
arxiv(2023)
摘要
Evaluating the performance of human is a common need across many applications, such as in engineering and sports. When evaluating human performance in completing complex and interactive tasks, the most common way is to use a metric having been proved efficient for that context, or to use subjective measurement techniques. However, this can be an error prone and unreliable process since static metrics cannot capture all the complex contexts associated with such tasks and biases exist in subjective measurement. The objective of our research is to create data-driven AI agents as computational benchmarks to evaluate human performance in solving difficult tasks involving multiple humans and contextual factors. We demonstrate this within the context of football performance analysis. We train a generative model based on Conditional Variational Recurrent Neural Network (VRNN) Model on a large player and ball tracking dataset. The trained model is used to imitate the interactions between two teams and predict the performance from each team. Then the trained Conditional VRNN Model is used as a benchmark to evaluate team performance. The experimental results on Premier League football dataset demonstrates the usefulness of our method to existing state-of-the-art static metric used in football analytics.
更多查看译文
关键词
imitation,evaluating human performance,complex interactive tasks,computational benchmark
AI 理解论文
溯源树
样例
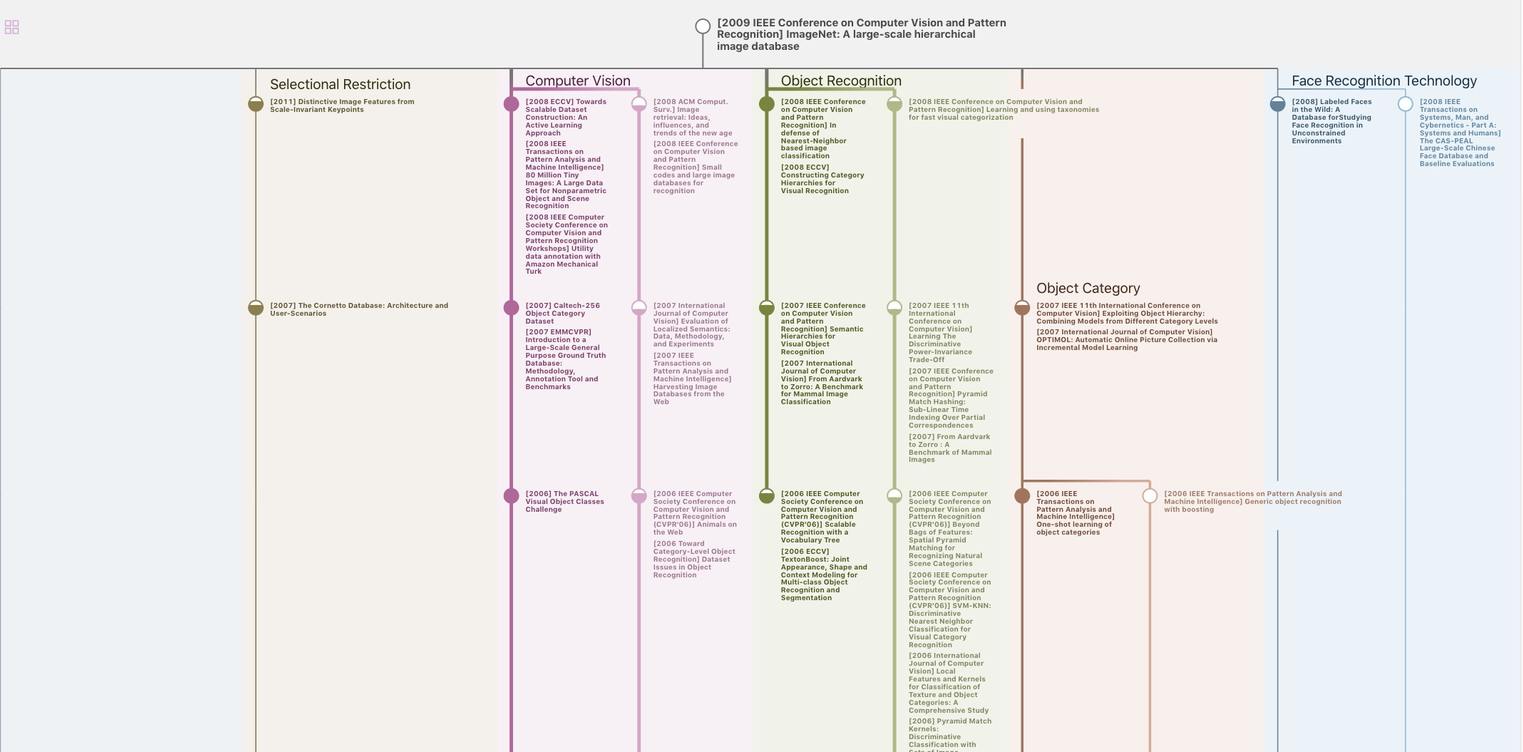
生成溯源树,研究论文发展脉络
Chat Paper
正在生成论文摘要