CA$^2$T-Net: Category-Agnostic 3D Articulation Transfer from Single Image
arxiv(2023)
摘要
We present a neural network approach to transfer the motion from a single image of an articulated object to a rest-state (i.e., unarticulated) 3D model. Our network learns to predict the object's pose, part segmentation, and corresponding motion parameters to reproduce the articulation shown in the input image. The network is composed of three distinct branches that take a shared joint image-shape embedding and is trained end-to-end. Unlike previous methods, our approach is independent of the topology of the object and can work with objects from arbitrary categories. Our method, trained with only synthetic data, can be used to automatically animate a mesh, infer motion from real images, and transfer articulation to functionally similar but geometrically distinct 3D models at test time.
更多查看译文
关键词
3d,transfer,t-net,category-agnostic
AI 理解论文
溯源树
样例
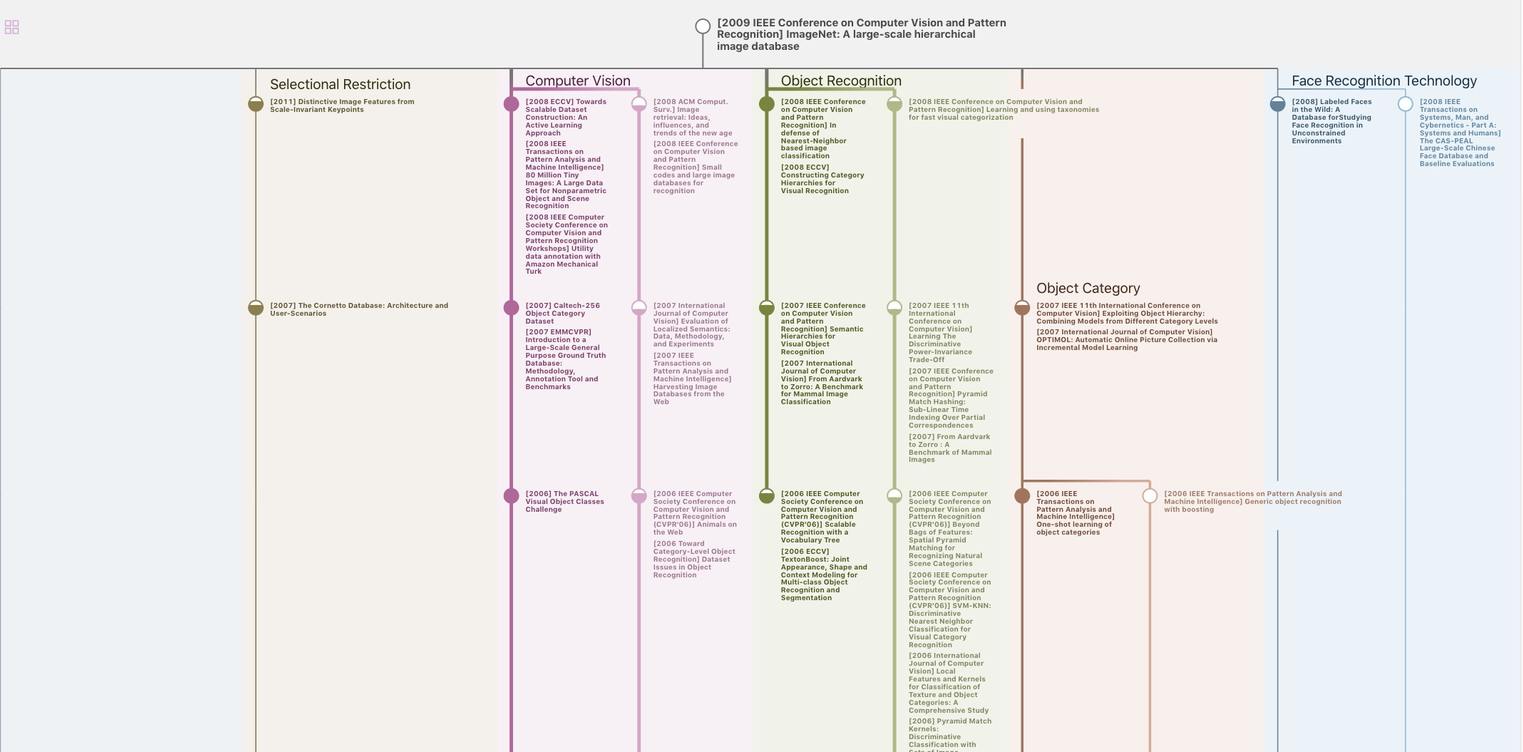
生成溯源树,研究论文发展脉络
Chat Paper
正在生成论文摘要