Retrieving canopy nitrogen concentration and aboveground biomass with deep learning for ryegrass and barley: comparing models and determining waveband contribution
Field Crops Research(2023)
摘要
Real-time crop canopy nitrogen concentration (CNC) and aboveground biomass (AGB) sensing capability can enable precision agriculture with significant economic and ecological benefits. Canopy spectral response in visible near-infrared (VNIR, 400–979 nm) has been widely used to estimate CNC and AGB of canopies but is often confounded by the soil background, crop type, phenological changes and environmental conditions. Machine (ML) and deep learning (DL) have emerged as promising techniques for mapping CNC and AGB using canopy spectral reflectance in VNIR. However, ML and DL models’ performance relies on extensive labelled training datasets, which are often limited due to being expensive and labour intensive. We estimated CNC and AGB using state-of-the-art hyperspectral (HS) modeling approaches using partial least squares regression (PLSR), random forest (RF), and one- and two-dimensional convolutional neural network (1D and 2D CNN). These models, together with multispectral (MS) approaches, were evaluated in the context of a small (422) dataset for model development collected from diverse fertilization and contrasting growth conditions. A handheld field spectroradiometer was used to collect spectral reflectance samples across a wide range (more than ten-fold difference in AGB) of canopy development stages in two seasons (winter and summer) in ryegrass, and across phenological stages (from GS-25 to GS-60) in barley. Results can be summarized in four components: (1) the median values of performance metrics in 1D CNN (R2test=0.83, NRMSEtest=0.15 for CNC; R2test=0.79 (ryegrass), 0.61 (barley), NRMSEtest= 0.29 (ryegrass), 0.30 (barley) for AGB) and PLSR (R2test=0.71, NRMSEtest= 0.20 for CNC; R2test=0.62 (ryegrass), 0.70 (barley), NRMSEtest= 0.38 (ryegrass), 0.27 (barley) for AGB) showed the best performance for CNC and AGB prediction in the test data; (2) when evaluated with the independent measurements, 1D CNN demonstrated the best (up to 56% decrease in NRMSE) performance for AGB and was comparable to PLSR for CNC (up to 25% decrease in NRMSE than their HS and MS counterparts); (3) predictive models underperformed at low canopy cover for CNC estimation and were adversely affected by the high biomass saturation effects for AGB prediction; and (4) visible spectrum is relatively more informative to CNC models whereas the red edge to NIR spectrum is more informative to AGB models. This study demonstrates the potential and limitations of commonly available field VNIR spectral reflectance data for CNC and AGB mapping across contrasting conditions in a small dataset (<500).
更多查看译文
关键词
Hyperspectral remote sensing,Deep learning,SHapley Additive exPlanations (SHAP),Crop sensing
AI 理解论文
溯源树
样例
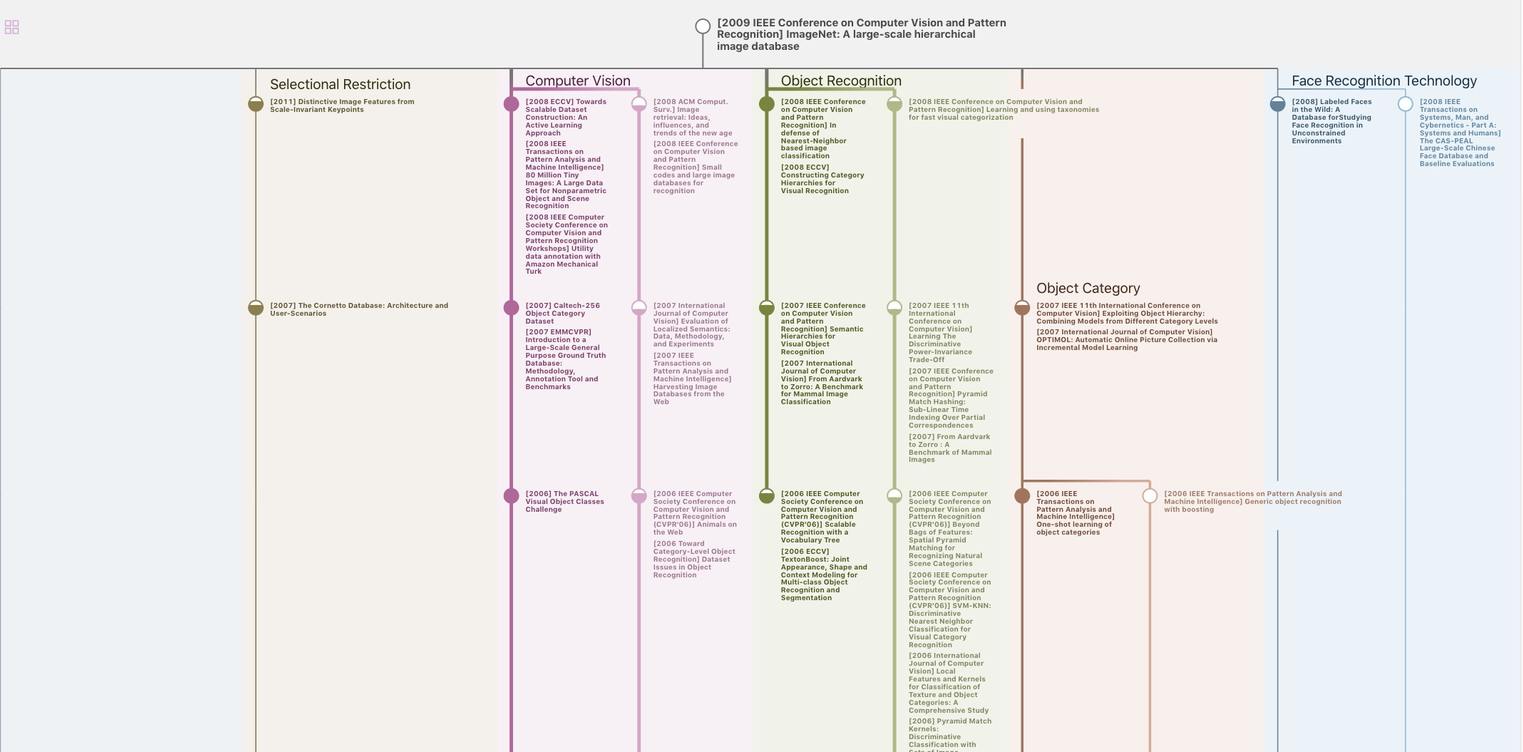
生成溯源树,研究论文发展脉络
Chat Paper
正在生成论文摘要