A machine learning methodology to quantify the potential of urban densification in the Oxford-Cambridge Arc, United Kingdom
Sustainable Cities and Society(2023)
摘要
Regional-scale urban residential densification provides an opportunity to tackle multiple challenges of sustainability in cities. But framework for detailed large-scale analysis of densification potentials and their integration with natural capital to assess the housing capacity is lacking. Using a combination of Machine Learning Random Forests algorithm and exploratory data analysis (EDA), we propose density scenarios and housing-capacity estimates for the potential residential lands in the Oxford–Cambridge Arc region (whose current population of 3.7 million is expected to increase up to 4.7 million in 2035) in the UK. A detailed analysis was done for Oxfordshire, assuming different densities in urban and rural areas and protecting lands with high-value natural capital from development. For a 30,000 dwellings-per-year scenario, the land allocated in Local Plans could cover housing growth in the four districts but not in Oxford City itself (which accounts for 48% of the demand); only 19% of the need would be covered in low but 59% in high housing density scenarios. Our study suggests a decision-support method for quantifying how the impact of housing growth on natural capital can be significantly reduced using more compact development patterns, protection of land with high-value natural capital, and use of low-biodiversity brownfield sites where available.
更多查看译文
关键词
Machine Learning,Exploratory data analysis,Oxford-Cambridge Arc,Housing density,Brownfield lands,Local Plans,Regional scale
AI 理解论文
溯源树
样例
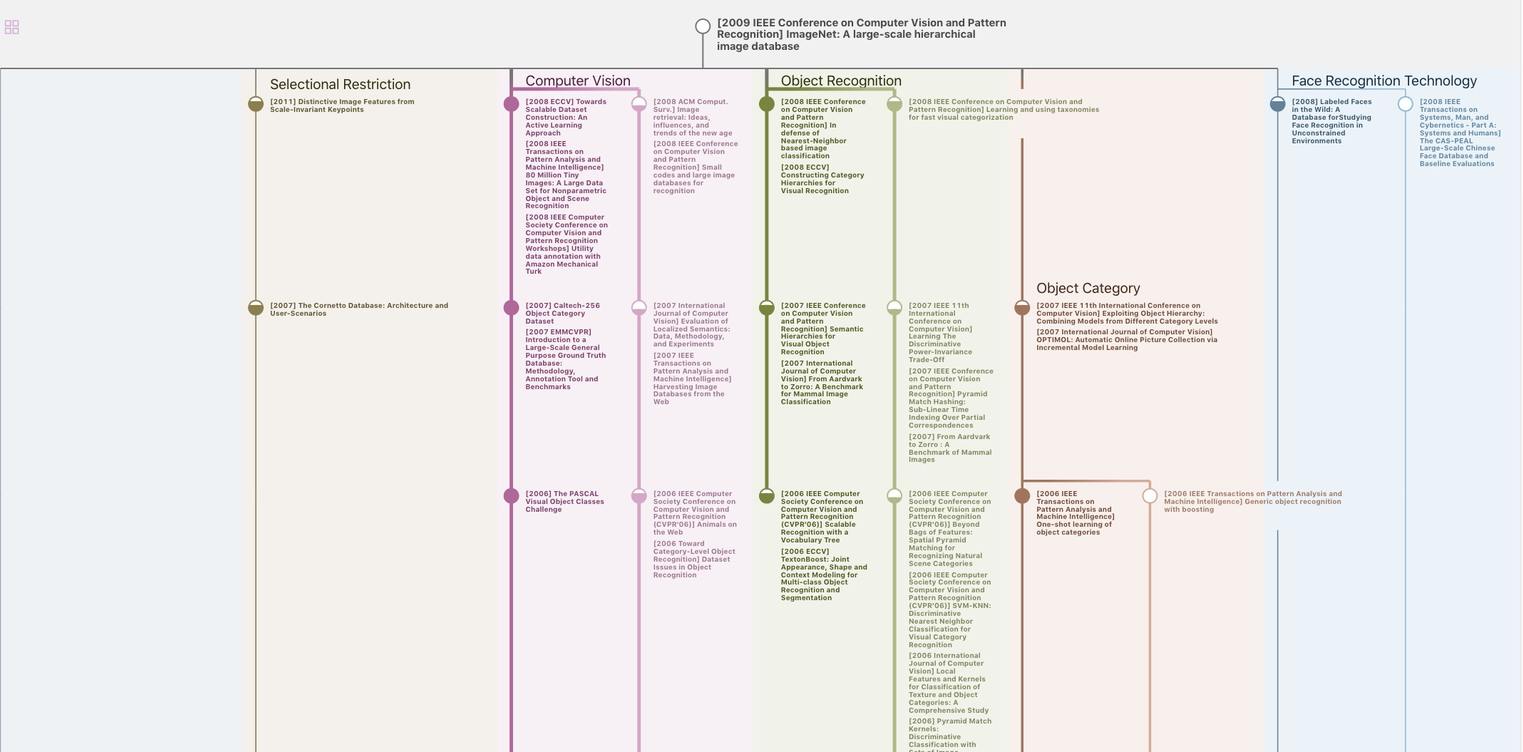
生成溯源树,研究论文发展脉络
Chat Paper
正在生成论文摘要