Prediction of estimated time of arrival for multi-airport systems via “Bubble” mechanism
Transportation Research Part C: Emerging Technologies(2023)
摘要
Predicting Estimated Time of Arrival (ETA) for a Multi-Airport System (MAS) is much more challenging than for a single airport system because of complex air route structure, dense air traffic volume and vagaries of traffic conditions in an MAS. In this work, we propose a novel “Bubble” mechanism to accurately predict medium-term ETA for a Multi-Airport System (MAS), in which the prediction of travel time of an origin–destination (OD) pair is decomposed into two stages, termed as out-MAS and in-MAS stages. For the out-MAS stage, Auto-Regressive Integrated Moving Average (ARIMA) is used to predict the travel time of a flight to reach the MAS boundary. For the in-MAS stage, we construct new spatio-temporal features based on clustering analysis of trajectory patterns facilitated by a novel data-driven hybrid polar sampling method. A sequence-to-sequence prediction model, Multi-variate Stacked Fully connected Bidirectional Long–Short Term Memory, is further developed to achieve multi-step-ahead predictions of in-MAS travel time for each trajectory pattern using the spatio-temporal features as input. Finally, the medium-term ETA prediction for an MAS is achieved by integrating the out-MAS and in-MAS prediction with the help of trajectory pattern prediction via random forest. A case study of predicting medium-term ETA for a typical MAS in China, Guangdong–Hong Kong–Macao Greater Bay Area, is conducted to demonstrate the usage and promising performance of the proposed method in comparison to several commonly used end-to-end learning methods.
更多查看译文
关键词
Multi-airport systems,Flight estimated time of arrival,Medium-term prediction,Trajectory pattern clustering,Sequence-to-sequence model,Spatio-temporal features
AI 理解论文
溯源树
样例
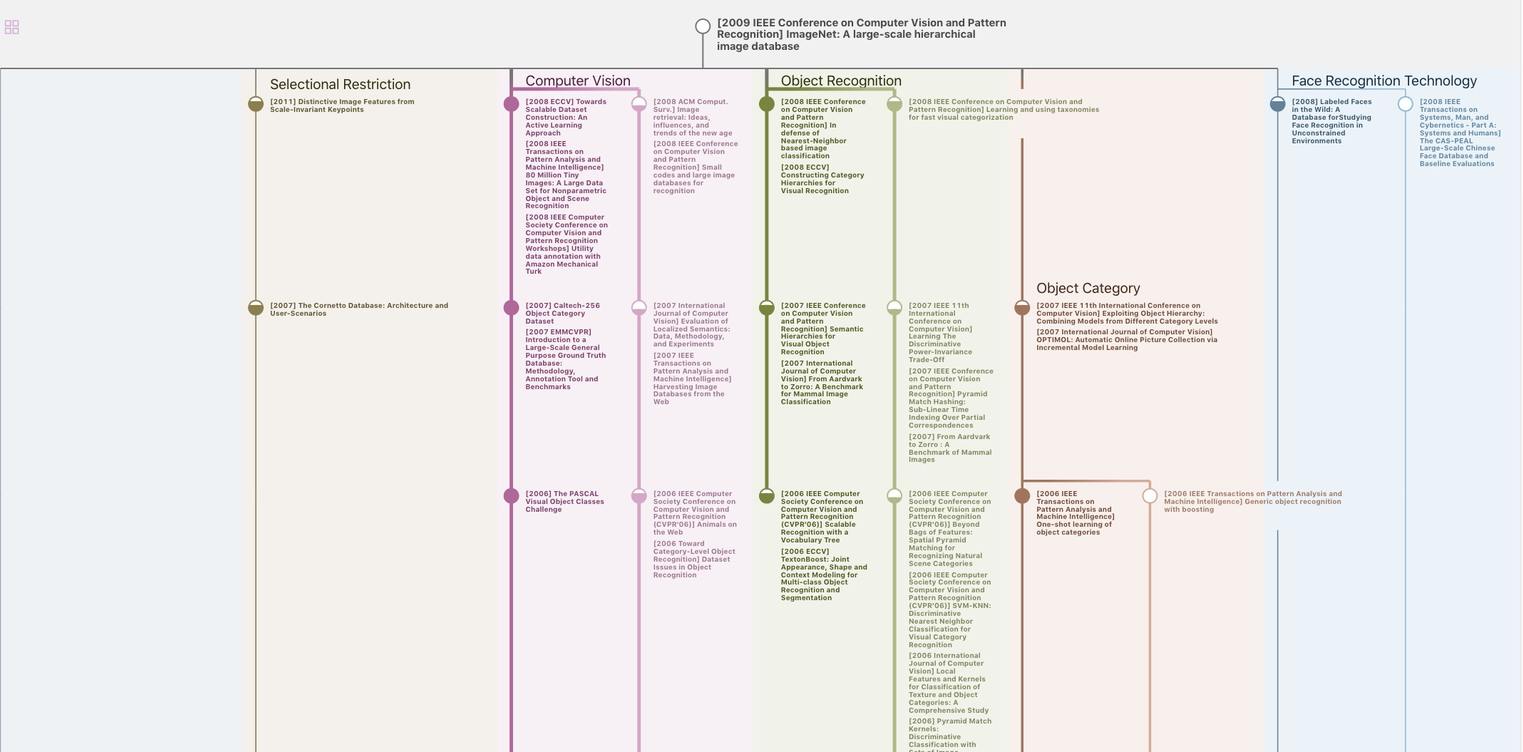
生成溯源树,研究论文发展脉络
Chat Paper
正在生成论文摘要