Advanced variant classification framework reduces the false positive rate of predicted loss of function (pLoF) variants in population sequencing data
medRxiv : the preprint server for health sciences(2023)
摘要
Predicted loss of function (pLoF) variants are highly deleterious and play an important role in disease biology, but many of these variants may not actually result in loss-of-function. Here we present a framework that advances interpretation of pLoF variants in research and clinical settings by considering three categories of LoF evasion: (1) predicted rescue by secondary sequence properties, (2) uncertain biological relevance, and (3) potential technical artifacts. We also provide recommendations on adjustments to ACMG/AMP guidelines’s PVS1 criterion. Applying this framework to all high-confidence pLoF variants in 22 autosomal recessive disease-genes from the Genome Aggregation Database (gnomAD, v2.1.1) revealed predicted LoF evasion or potential artifacts in 27.3% (304/1,113) of variants. The major reasons were location in the last exon, in a homopolymer repeat, in low per-base expression (pext) score regions, or the presence of cryptic splice rescues. Variants predicted to be potential artifacts or to evade LoF were enriched for ClinVar benign variants. PVS1 was downgraded in 99.4% (162/163) of LoF evading variants assessed, with 17.2% (28/163) downgraded as a result of our framework, adding to previous guidelines. Variant pathogenicity was affected (mostly from likely pathogenic to VUS) in 20 (71.4%) of these 28 variants. This framework guides assessment of pLoF variants beyond standard annotation pipelines, and substantially reduces false positive rates, which is key to ensure accurate LoF variant prediction in both a research and clinical setting.
### Competing Interest Statement
S.H. is an employee of Ambry Genetics. D.G.M. is a founder with equity of Goldfinch Bio, serves as a paid advisor to GSK, Variant Bio, Insitro, and Foresite Labs, and received research funding from Genzyme. H.L.R. receives funding from Illumina to support rare disease gene discovery and diagnosis. A.O-D.L. is a paid advisor of Tome Biosciences, Congenica, and the Simons Foundation SPARK for Autism study.
### Funding Statement
This work was supported in part by the following NIH grants: NHGRI U24HG011450, NHGRI U24HG006834, NHGRI U01HG011755, and NHLBI R01HL143295, and by research funding from the Chan Zuckerberg Initiative to H.L.R. and from Genzyme Corporation to D.G.M. S.G. was supported by The Knut and Alice Wallenberg Foundation scholarship program for postdoctoral studies at the Broad Institute. Its contents are solely the responsibility of the authors and do not necessarily represent the official views of NIH, CZI, or Genzyme.
### Author Declarations
I confirm all relevant ethical guidelines have been followed, and any necessary IRB and/or ethics committee approvals have been obtained.
Yes
I confirm that all necessary patient/participant consent has been obtained and the appropriate institutional forms have been archived, and that any patient/participant/sample identifiers included were not known to anyone (e.g., hospital staff, patients or participants themselves) outside the research group so cannot be used to identify individuals.
Yes
I understand that all clinical trials and any other prospective interventional studies must be registered with an ICMJE-approved registry, such as ClinicalTrials.gov. I confirm that any such study reported in the manuscript has been registered and the trial registration ID is provided (note: if posting a prospective study registered retrospectively, please provide a statement in the trial ID field explaining why the study was not registered in advance).
Yes
I have followed all appropriate research reporting guidelines, such as any relevant EQUATOR Network research reporting checklist(s) and other pertinent material, if applicable.
Yes
All data produced in the present work are contained in the manuscript
更多查看译文
关键词
advanced variant classification framework,predicted,loss-of-function
AI 理解论文
溯源树
样例
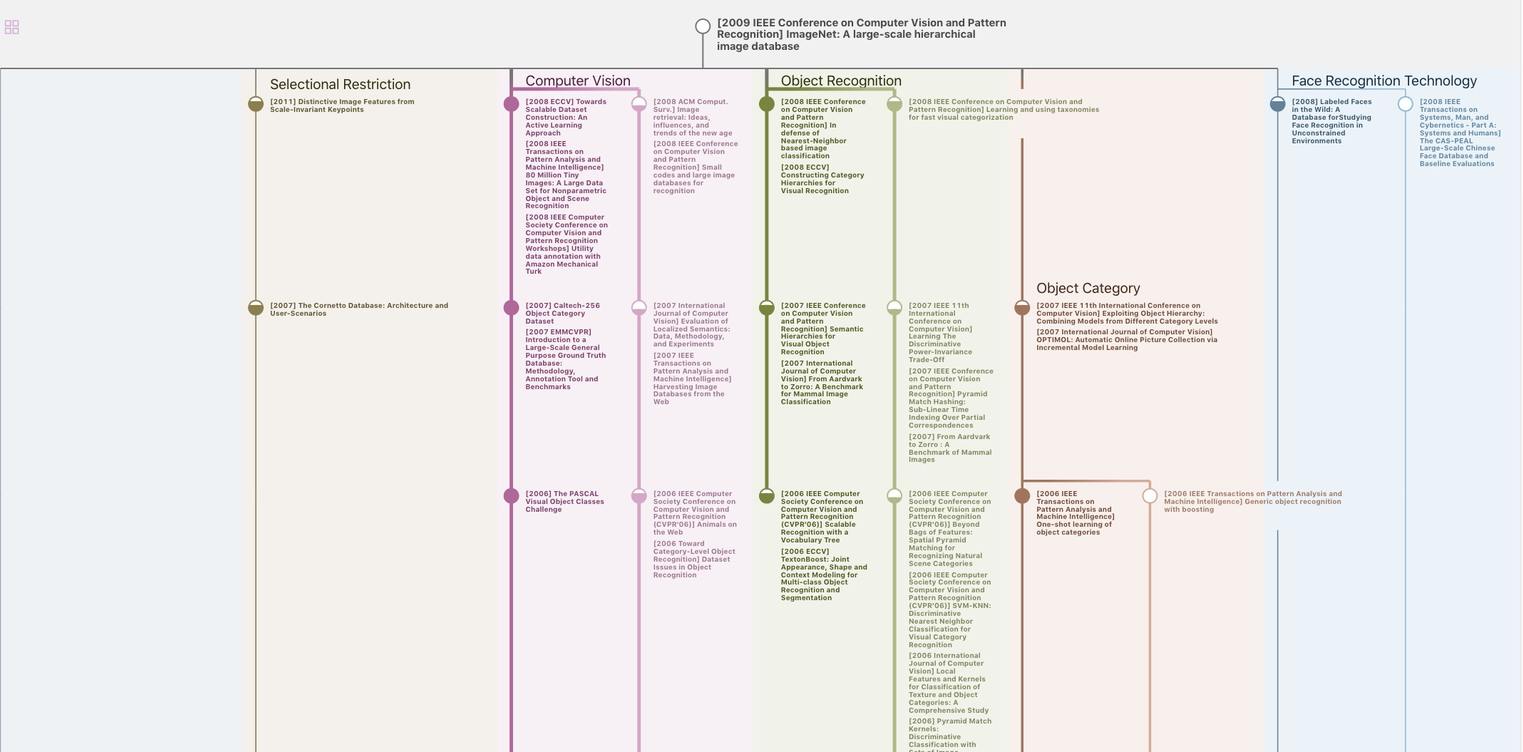
生成溯源树,研究论文发展脉络
Chat Paper
正在生成论文摘要