Revisiting DeepFool: generalization and improvement
arxiv(2023)
摘要
Deep neural networks have been known to be vulnerable to adversarial examples, which are inputs that are modified slightly to fool the network into making incorrect predictions. This has led to a significant amount of research on evaluating the robustness of these networks against such perturbations. One particularly important robustness metric is the robustness to minimal l2 adversarial perturbations. However, existing methods for evaluating this robustness metric are either computationally expensive or not very accurate. In this paper, we introduce a new family of adversarial attacks that strike a balance between effectiveness and computational efficiency. Our proposed attacks are generalizations of the well-known DeepFool (DF) attack, while they remain simple to understand and implement. We demonstrate that our attacks outperform existing methods in terms of both effectiveness and computational efficiency. Our proposed attacks are also suitable for evaluating the robustness of large models and can be used to perform adversarial training (AT) to achieve state-of-the-art robustness to minimal l2 adversarial perturbations.
更多查看译文
关键词
deepfool,generalization
AI 理解论文
溯源树
样例
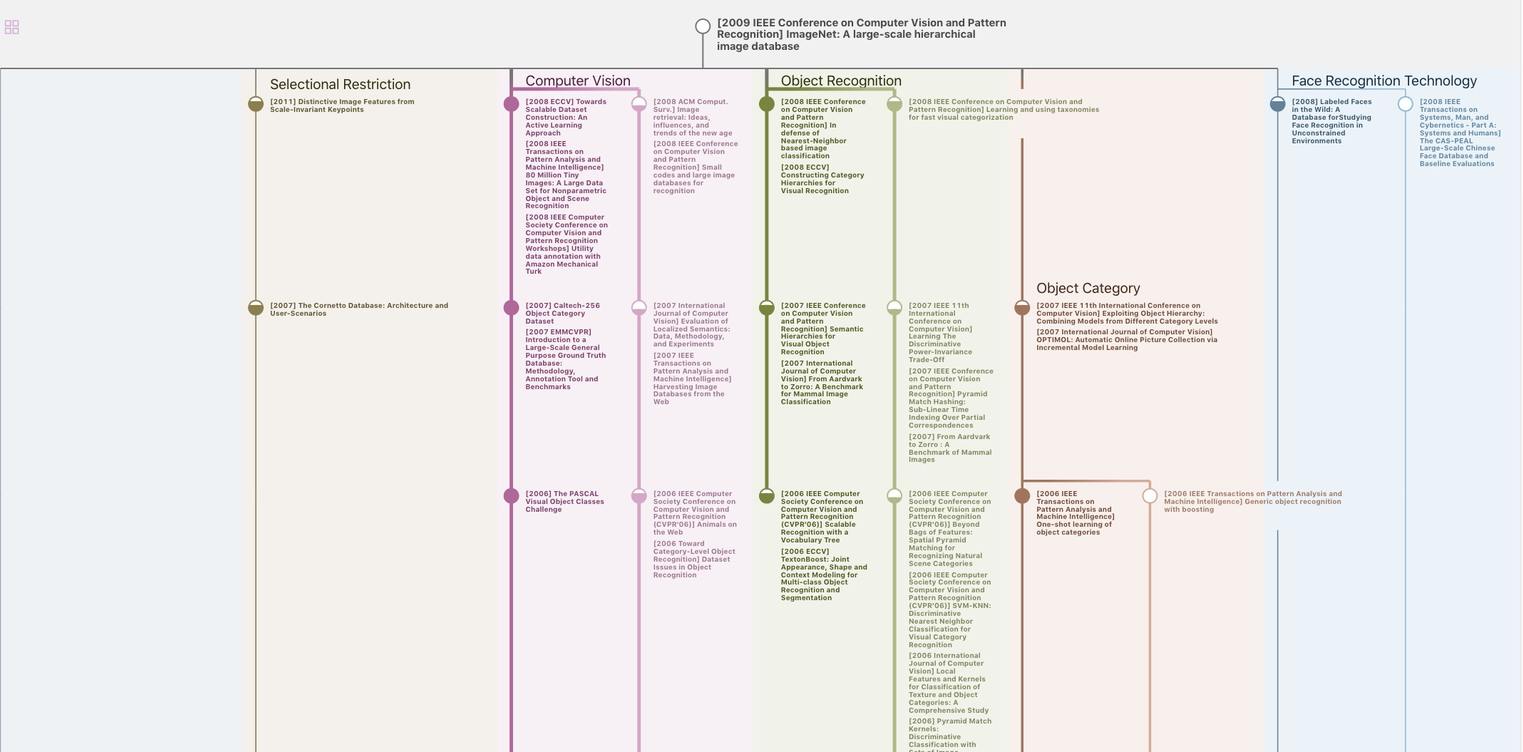
生成溯源树,研究论文发展脉络
Chat Paper
正在生成论文摘要