Learning Human-Inspired Force Strategies for Robotic Assembly
arXiv (Cornell University)(2023)
摘要
The programming of robotic assembly tasks is a key component in manufacturing and automation. Force-sensitive assembly, however, often requires reactive strategies to handle slight changes in positioning and unforeseen part jamming. Learning such strategies from human performance is a promising approach, but faces two common challenges: the handling of low part clearances which is difficult to capture from demonstrations and learning intuitive strategies offline without access to the real hardware. We address these two challenges by learning probabilistic force strategies from data that are easily acquired offline in a robot-less simulation from human demonstrations with a joystick. We combine a Long Short Term Memory (LSTM) and a Mixture Density Network (MDN) to model human-inspired behavior in such a way that the learned strategies transfer easily onto real hardware. The experiments show a UR10e robot that completes a plastic assembly with clearances of less than 100 micrometers whose strategies were solely demonstrated in simulation.
更多查看译文
关键词
robotic assembly,force,learning,human-inspired
AI 理解论文
溯源树
样例
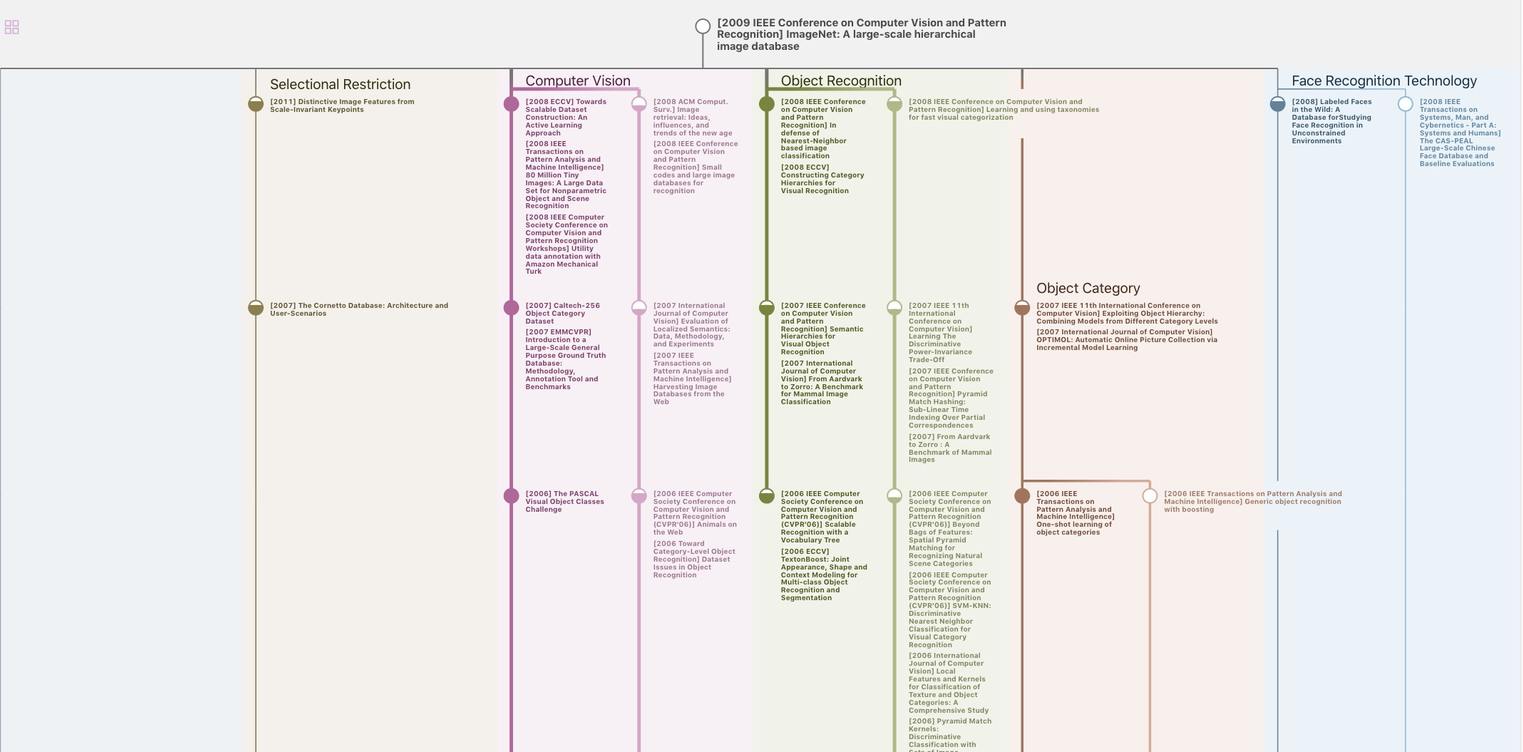
生成溯源树,研究论文发展脉络
Chat Paper
正在生成论文摘要