One-Step Detection Paradigm for Hyperspectral Anomaly Detection via Spectral Deviation Relationship Learning
IEEE TRANSACTIONS ON GEOSCIENCE AND REMOTE SENSING(2024)
摘要
Hyperspectral anomaly detection (HAD) aims to find small targets deviating from surroundings in an unsupervised manner. Recently, various deep models have been applied to HAD, such as autoencoder series and generative adversarial networks (GANs) series, which mainly use a proxy task, i.e., iteratively reconstructing low-frequency components (backgrounds) to separate anomalies (two-step paradigm). However, in such an unsupervised manner, most deep HAD model is trained and tested on the same image. Since the learned low-frequency background varies from image to image and the trained model cannot be directly transferred to unseen images. In this article, the one-step detection paradigm is first proposed, where the model is optimized directly for the HAD task and can be transferred to unseen datasets. The one-step paradigm is optimized to identify the spectral deviation relationship according to the anomaly definition. Compared with learning the specific background distribution in the two-step paradigm, the spectral deviation relationship is universal for different images and guarantees transferability. Furthermore, we instantiated the one-step paradigm as an unsupervised transferred direct detection (TDD) model. To train the TDD model in an unsupervised manner, an anomaly sample simulation strategy is proposed to generate numerous pairs of anomaly samples. A global self-attention module (GAM) and a local self-attention module (LAM) are designed to help the model focus on the "spectrally deviating" relationship. The TDD model was validated on six public datasets. The results show that TDD is superior to the recent two-step methods in detection and transferability aspects.
更多查看译文
关键词
Anomaly detection,deep learning,hyperspectral imagery (HSI),spectral deviation,unified model
AI 理解论文
溯源树
样例
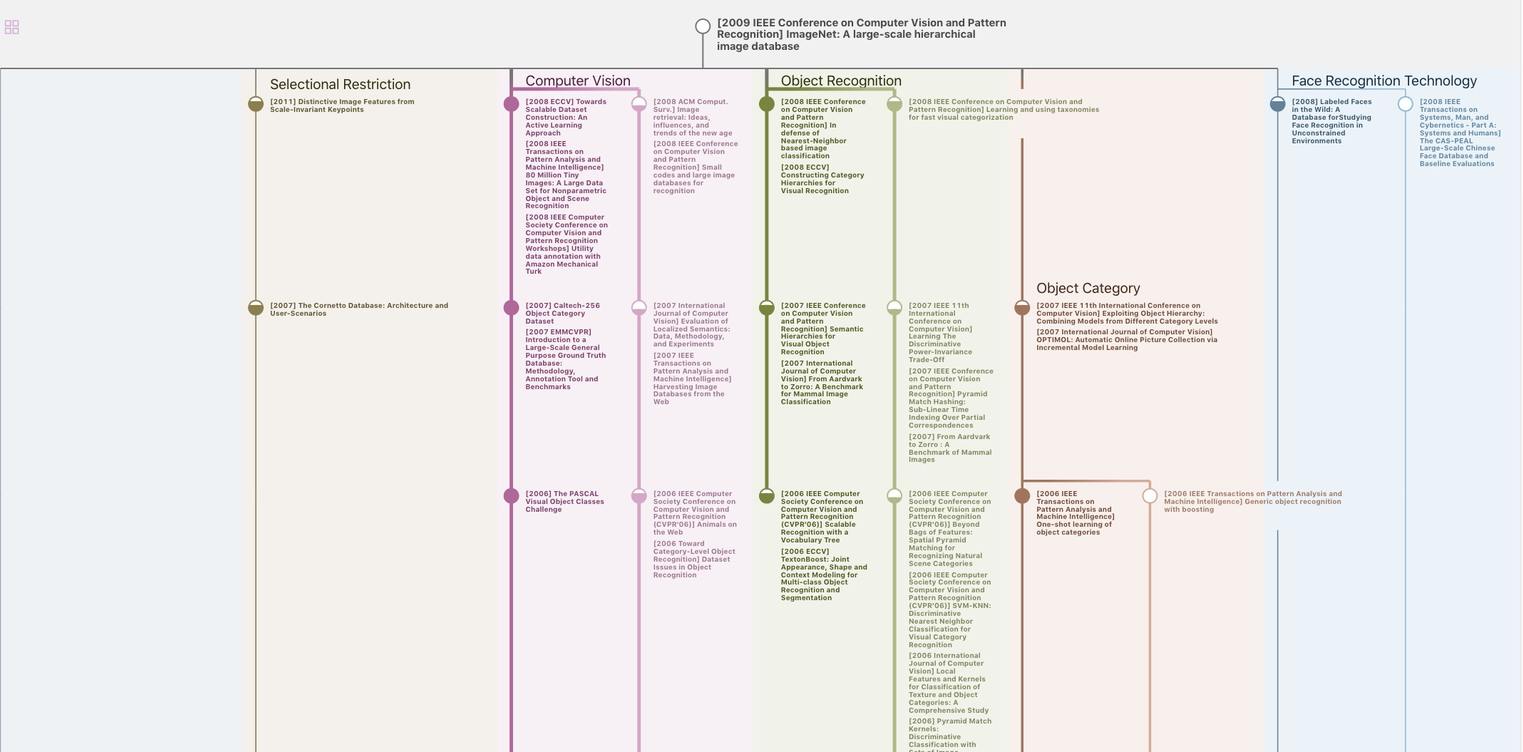
生成溯源树,研究论文发展脉络
Chat Paper
正在生成论文摘要