System and Design Technology Co-Optimization of SOT-MRAM for High-Performance AI Accelerator Memory System
IEEE TRANSACTIONS ON COMPUTER-AIDED DESIGN OF INTEGRATED CIRCUITS AND SYSTEMS(2024)
摘要
System on chips (SoCs) are now designed withtheir own artificial intelligence (AI) accelerator segment toaccommodate the ever-increasing demand of deep learning (DL)applications. With powerful multiply and accumulate (MAC)engines for matrix multiplications, these accelerators show highcomputing performance. However, because of limited memoryresources (i.e., bandwidth and capacity), they fail to achieveoptimum system performance during large batch training andinference. In this work, we propose a memory system withhigh on-chip capacity and bandwidth to shift the gear of AIaccelerators from memory-bound to achieving system-level peakperformance. We develop the memory system with design tech-nology co-optimization (DTCO)-enabled customized spin-orbittorque (SOT)-MRAM as large on-chip memory through systemtechnology co-optimization (STCO) and detailed characterizationof the DL workloads. Our workload-aware memory systemachieves 8xenergy and 9xlatency improvement on computervision (CV) benchmarks in training and 8xenergy and 4.5xlatency improvement on natural language processing (NLP)benchmarks in training while consuming only around 50% ofSRAM area at iso-capacity
更多查看译文
关键词
Random access memory,System-on-chip,Training,Magnetic tunneling,AI accelerators,Data models,Codes,Artificial intelligence (AI) accelerator,design technology co-optimization (DTCO),spin orbit torque (SOT)-MRAM,system technology co-optimization (STCO)
AI 理解论文
溯源树
样例
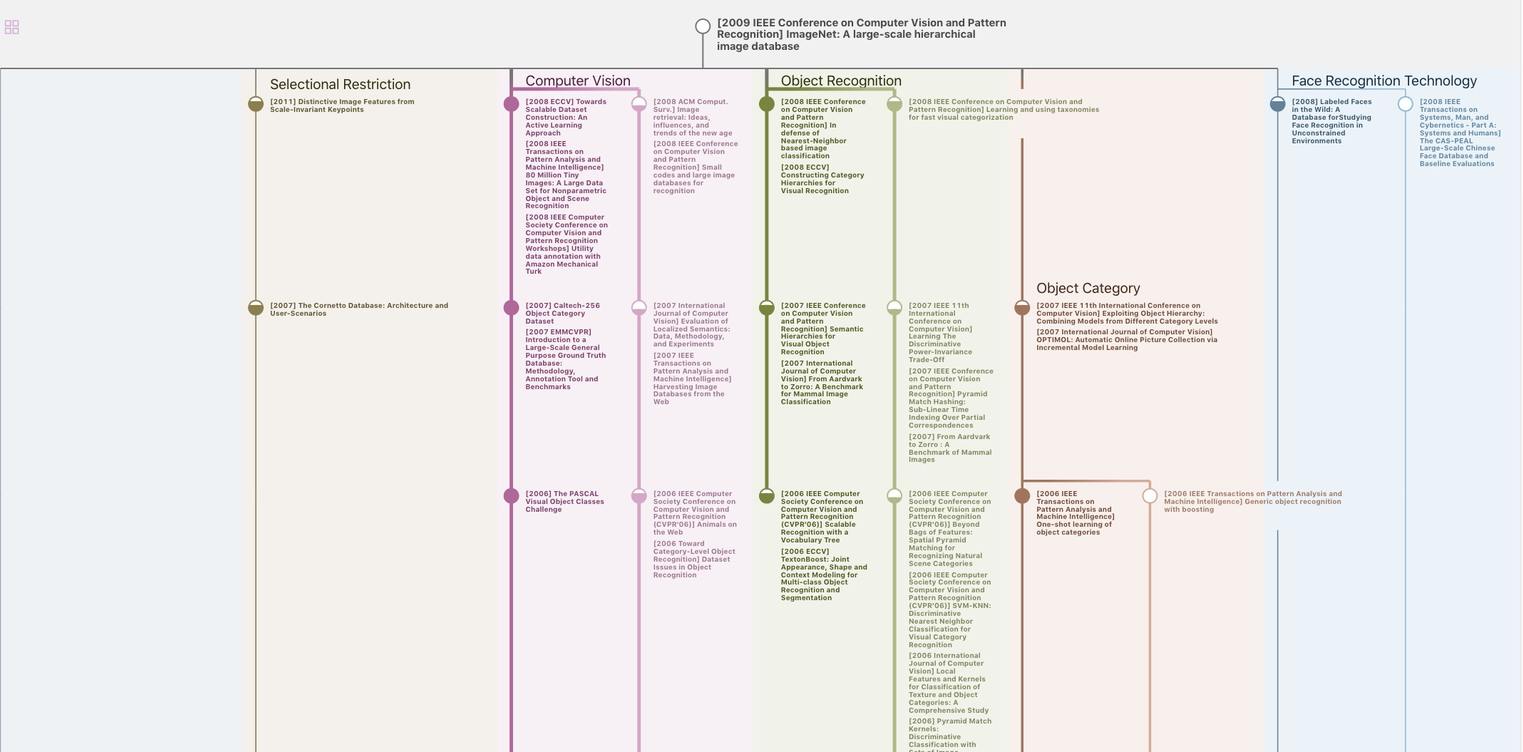
生成溯源树,研究论文发展脉络
Chat Paper
正在生成论文摘要