Infrastructure-based End-to-End Learning and Prevention of Driver Failure
2023 IEEE INTERNATIONAL CONFERENCE ON ROBOTICS AND AUTOMATION, ICRA(2023)
摘要
Intelligent intersection managers can improve safety by detecting dangerous drivers or failure modes in autonomous vehicles, warning oncoming vehicles as they approach an intersection. In this work, we present FailureNet, a recurrent neural network trained end-to-end on trajectories of both nominal and reckless drivers in a scaled miniature city. FailureNet observes the poses of vehicles as they approach an intersection and detects whether a failure is present in the autonomy stack, warning cross-traffic of potentially dangerous drivers. FailureNet can accurately identify control failures, upstream perception errors, and speeding drivers, distinguishing them from nominal driving. The network is trained and deployed with autonomous vehicles in the MiniCity. Compared to speed or frequency-based predictors, FailureNet's recurrent neural network structure provides improved predictive power, yielding upwards of 84% accuracy when deployed on hardware.
更多查看译文
AI 理解论文
溯源树
样例
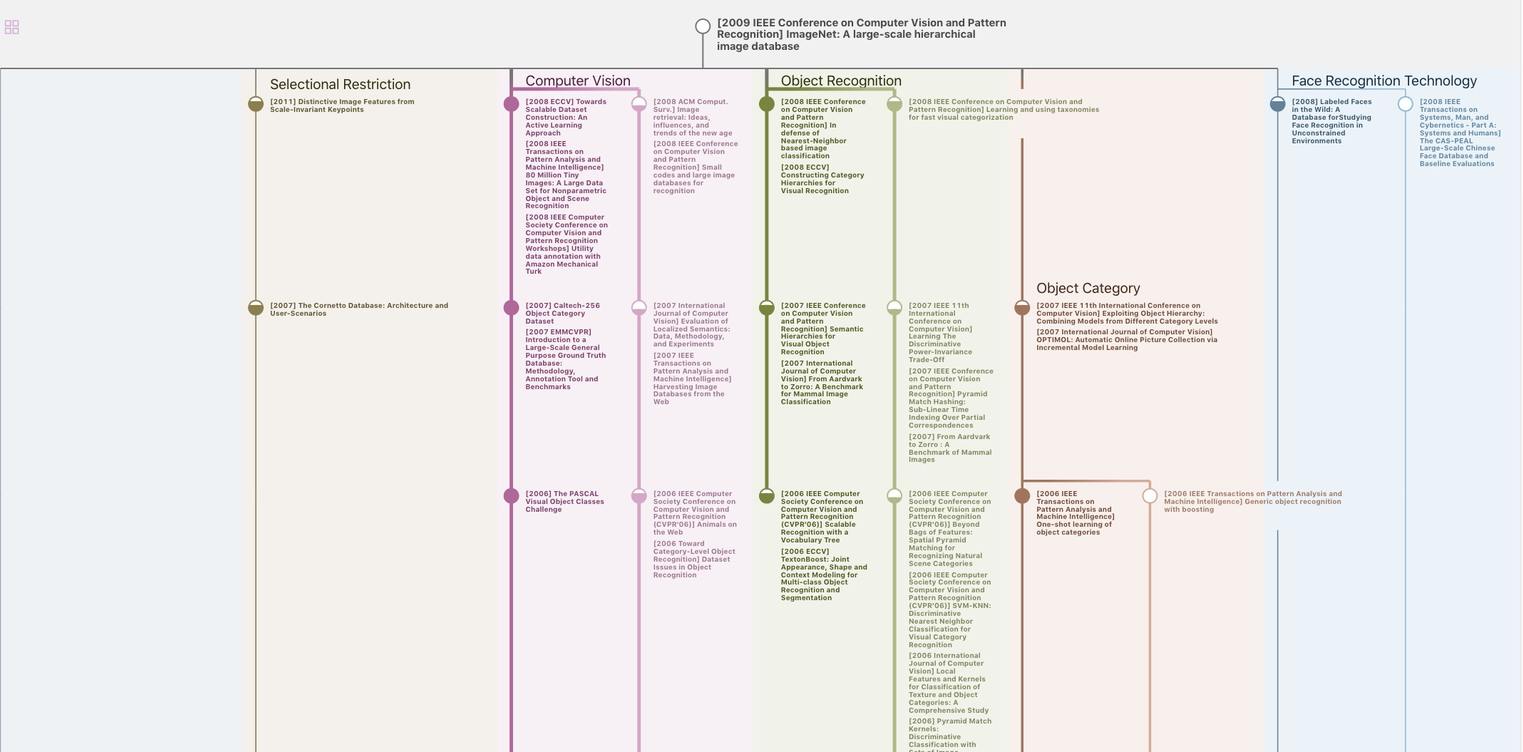
生成溯源树,研究论文发展脉络
Chat Paper
正在生成论文摘要