Robust Output-Lifted Learning Model Predictive Control
arxiv(2023)
摘要
We propose an iterative approach for designing Robust Learning Model Predictive Control (LMPC) policies for a class of nonlinear systems with additive, unmodelled dynamics. The nominal dynamics are assumed to be difference flat, i.e., the state and input can be reconstructed using flat output sequences. For the considered class of systems, we synthesize Robust MPC policies and show how to use historical trajectory data collected during iterative tasks to 1) obtain bounds on the unmodelled dynamics and 2) construct a convex value function approximation along with a convex safe set in the space of output sequences for designing terminal components in the Robust MPC design. We show that the proposed strategy guarantees robust constraint satisfaction, asymptotic convergence to a desired subset of the state space, and non-decreasing closed-loop performance at each policy update. Finally, simulation results demonstrate the effectiveness of the proposed strategy on a minimum time control problem using a constrained nonlinear and uncertain vehicle model.
更多查看译文
关键词
predictive control,model,output-lifted
AI 理解论文
溯源树
样例
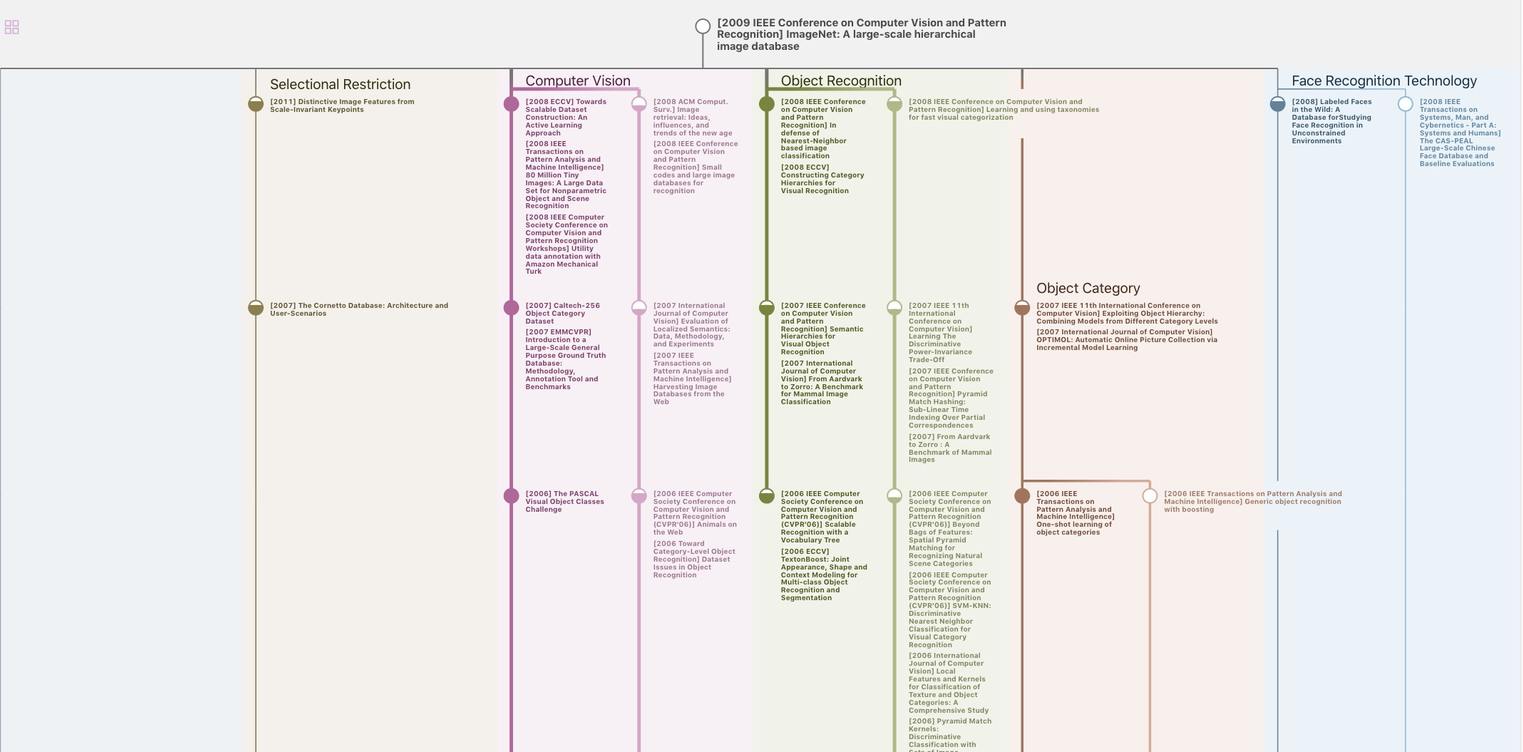
生成溯源树,研究论文发展脉络
Chat Paper
正在生成论文摘要