Physical-priors-guided DehazeFormer
Knowledge-Based Systems(2023)
Abstract
Single-image dehazing is a challenging task in several machine-vision applications. Methods based on physical models and prior knowledge fail under certain conditions, resulting in defects such as color distortion. Transformer-based methods have a strong representation ability owing to their self-attention mechanism that can effectively obtain global information. However, this approach is computationally expensive, and its weak inductive bias capability increases the risk of overfitting on small-sample datasets. To address these problems, in this study, we propose a novel DehazeFormer guided by physical priors, named SwinTD-Net, which is trained according to supervised and self-supervised learning, and combines the advantages of physical priors and transformers. The proposed DehazeFormer learns features guided by physical priors, which improves the generalization ability of the network and enables it to achieve good restoration effects on both synthetic and real-world hazy images. In addition, we propose a more appropriate prior input to better use physical priors, and we design a multi-scale dark-light enhancement algorithm for image restoration post-processing, which can improve the visual perception quality for human observers while performing some local enhancements. Extensive experiments illustrate that the proposed method outperforms state-of-the-art methods. The code and pre-trained models are available to academics so that they can reproduce our results and test them (https://github.com/hocking-cloud/SwinTD_Net).
MoreTranslated text
Key words
Image dehazing,Dark channel prior,Swin Transformer,Deep learning,Self-supervised learning
AI Read Science
Must-Reading Tree
Example
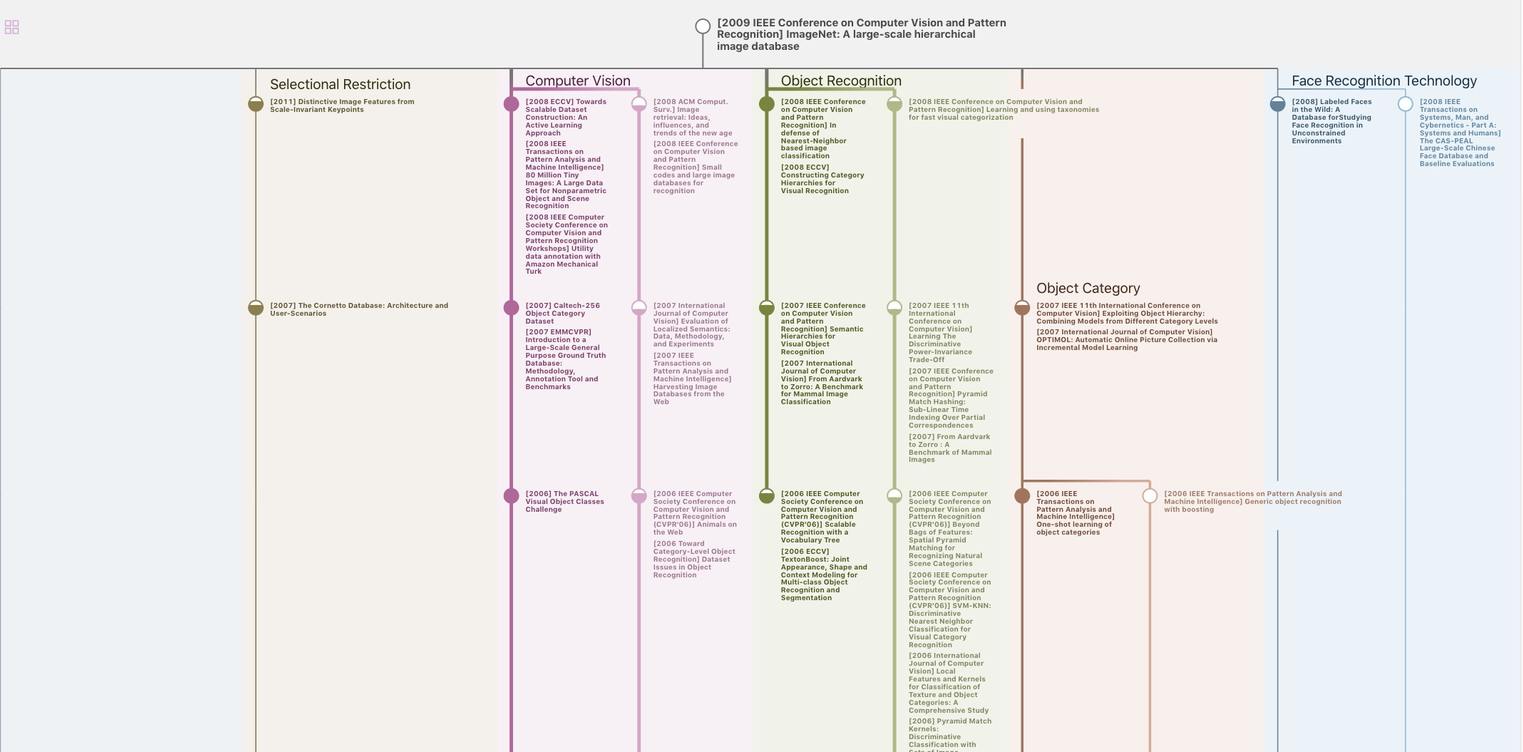
Generate MRT to find the research sequence of this paper
Chat Paper
Summary is being generated by the instructions you defined