A local spline regression-based framework for semi-supervised sparse feature selection
Knowledge-Based Systems(2023)
摘要
Feature selection (FS) is extensively applied in many machine learning applications for the selection of relevant features from data sets. A lot of unlabeled data are available in a variety of applications that can be exploited for semi-supervised FS to address the lack of labeled data and improve learning performance. Recently, semi-supervised sparse FS based on graph Laplacian has obtained considerable research interest, which uses the correlation between features in the process of FS. However, the Laplacian regularization has a weak extrapolating power and a bias towards the constant geodesic function, and cannot retain the local topology well. In this paper, a spline regression-based framework for semi-supervised sparse FS (SRS3FS) is proposed, which uses the mixed convex and non-convex ℓ2,p-norm (0 < p ≤1) regularization to select the relevant features and consider the correlation between features. The framework exploits local spline regression to retain the geometry structure of labeled and unlabeled data and encodes the data distribution. A unified iterative algorithm is presented to solve the proposed framework for the convex and non-convex cases, and its convergence is theoretically and experimentally proved. Experiments on several data sets illustrate the effectiveness of our framework in the selection of the most relevant and discriminative features.
更多查看译文
关键词
Semi-supervised feature selection,Spline regression,Sparse models
AI 理解论文
溯源树
样例
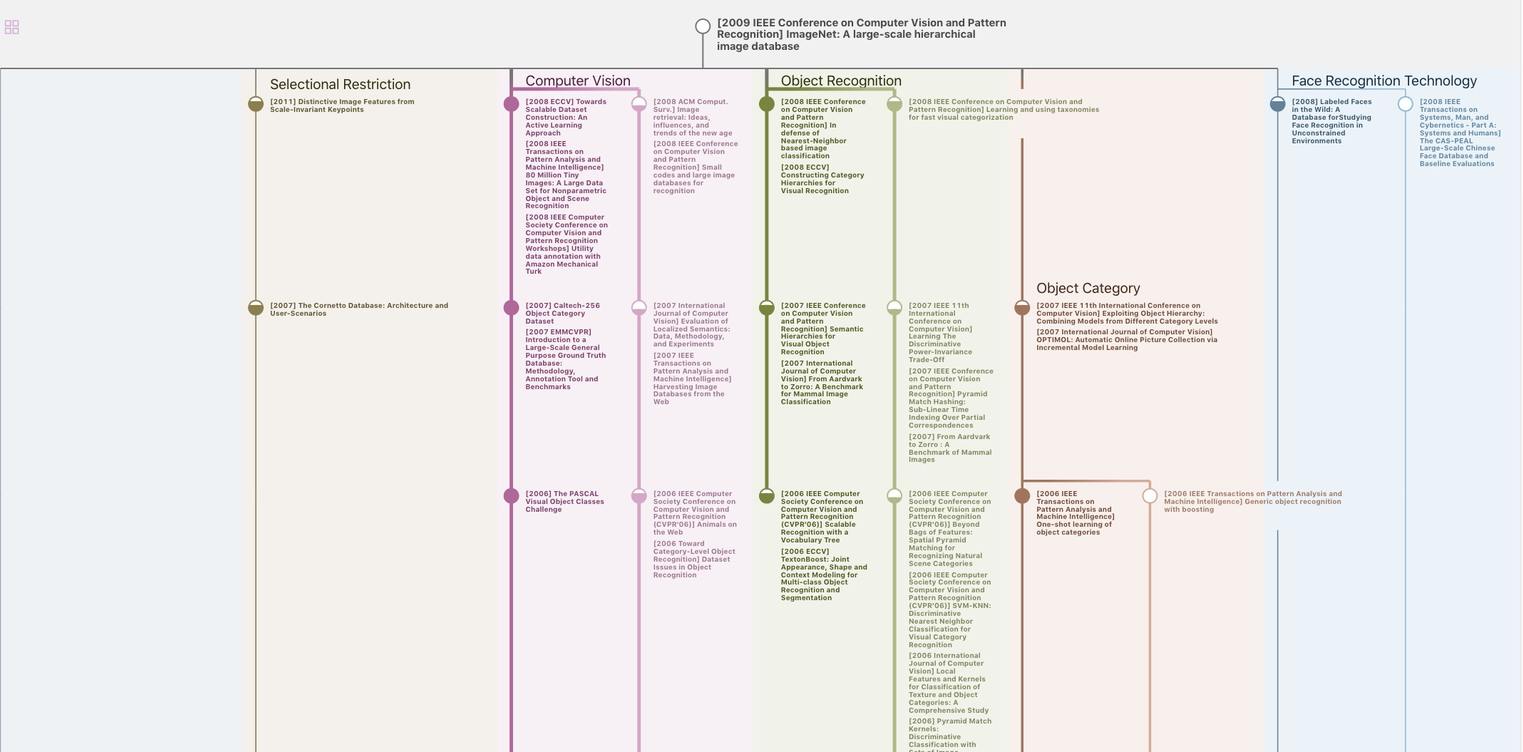
生成溯源树,研究论文发展脉络
Chat Paper
正在生成论文摘要