Machine Learning for Brain Disorders: Transformers and Visual Transformers
CoRR(2023)
摘要
Transformers were initially introduced for natural language processing (NLP) tasks, but fast they were adopted by most deep learning fields, including computer vision. They measure the relationships between pairs of input tokens (words in the case of text strings, parts of images for visual Transformers), termed attention. The cost is exponential with the number of tokens. For image classification, the most common Transformer Architecture uses only the Transformer Encoder in order to transform the various input tokens. However, there are also numerous other applications in which the decoder part of the traditional Transformer Architecture is also used. Here, we first introduce the Attention mechanism (Section 1), and then the Basic Transformer Block including the Vision Transformer (Section 2). Next, we discuss some improvements of visual Transformers to account for small datasets or less computation(Section 3). Finally, we introduce Visual Transformers applied to tasks other than image classification, such as detection, segmentation, generation and training without labels (Section 4) and other domains, such as video or multimodality using text or audio data (Section 5).
更多查看译文
关键词
brain disorders,transformers,machine learning
AI 理解论文
溯源树
样例
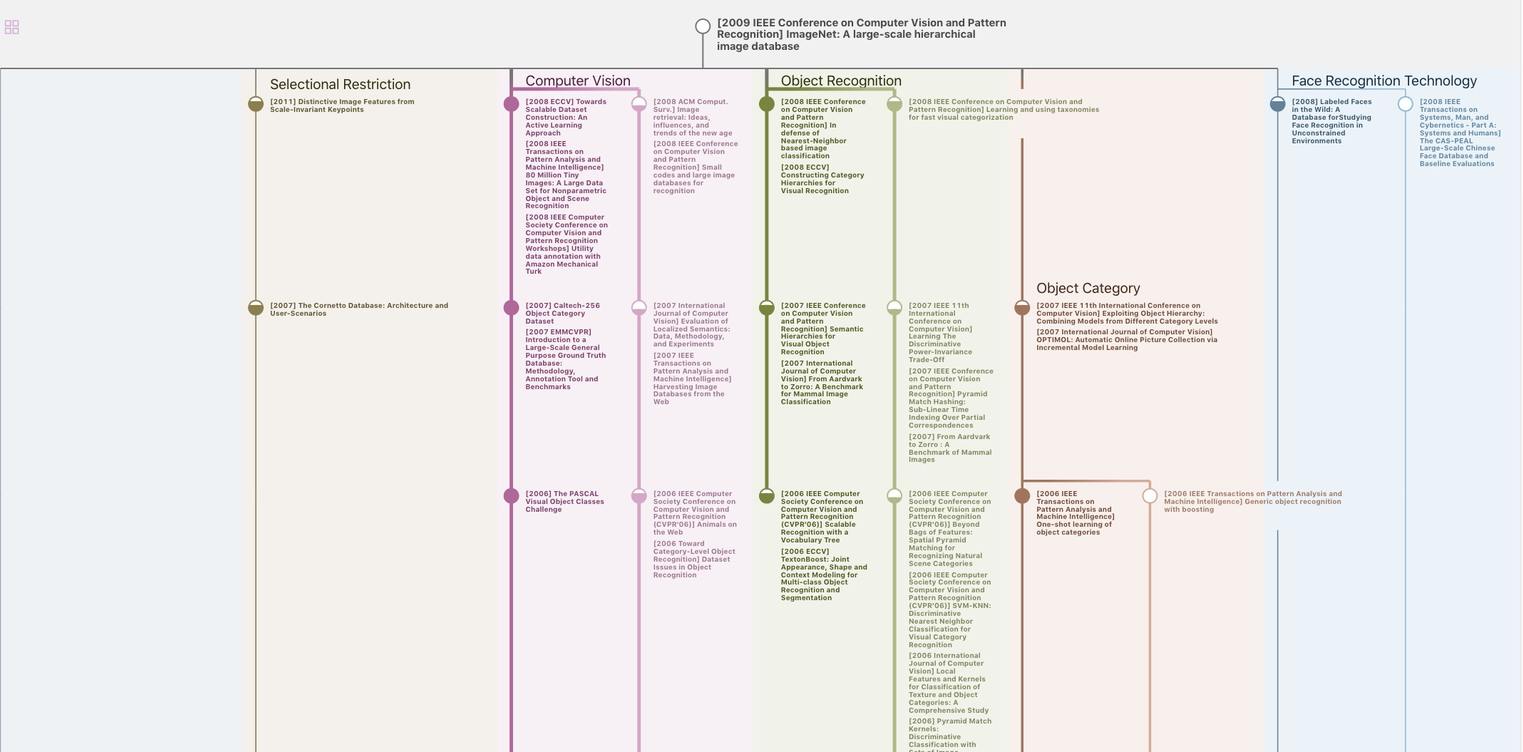
生成溯源树,研究论文发展脉络
Chat Paper
正在生成论文摘要