Modeling Relational Patterns for Logical Query Answering over Knowledge Graphs
arxiv(2023)
摘要
Answering first-order logical (FOL) queries over knowledge graphs (KG) remains a challenging task mainly due to KG incompleteness. Query embedding approaches this problem by computing the low-dimensional vector representations of entities, relations, and logical queries. KGs exhibit relational patterns such as symmetry and composition and modeling the patterns can further enhance the performance of query embedding models. However, the role of such patterns in answering FOL queries by query embedding models has not been yet studied in the literature. In this paper, we fill in this research gap and empower FOL queries reasoning with pattern inference by introducing an inductive bias that allows for learning relation patterns. To this end, we develop a novel query embedding method, RoConE, that defines query regions as geometric cones and algebraic query operators by rotations in complex space. RoConE combines the advantages of Cone as a well-specified geometric representation for query embedding, and also the rotation operator as a powerful algebraic operation for pattern inference. Our experimental results on several benchmark datasets confirm the advantage of relational patterns for enhancing logical query answering task.
更多查看译文
关键词
relational patterns,logical query,knowledge
AI 理解论文
溯源树
样例
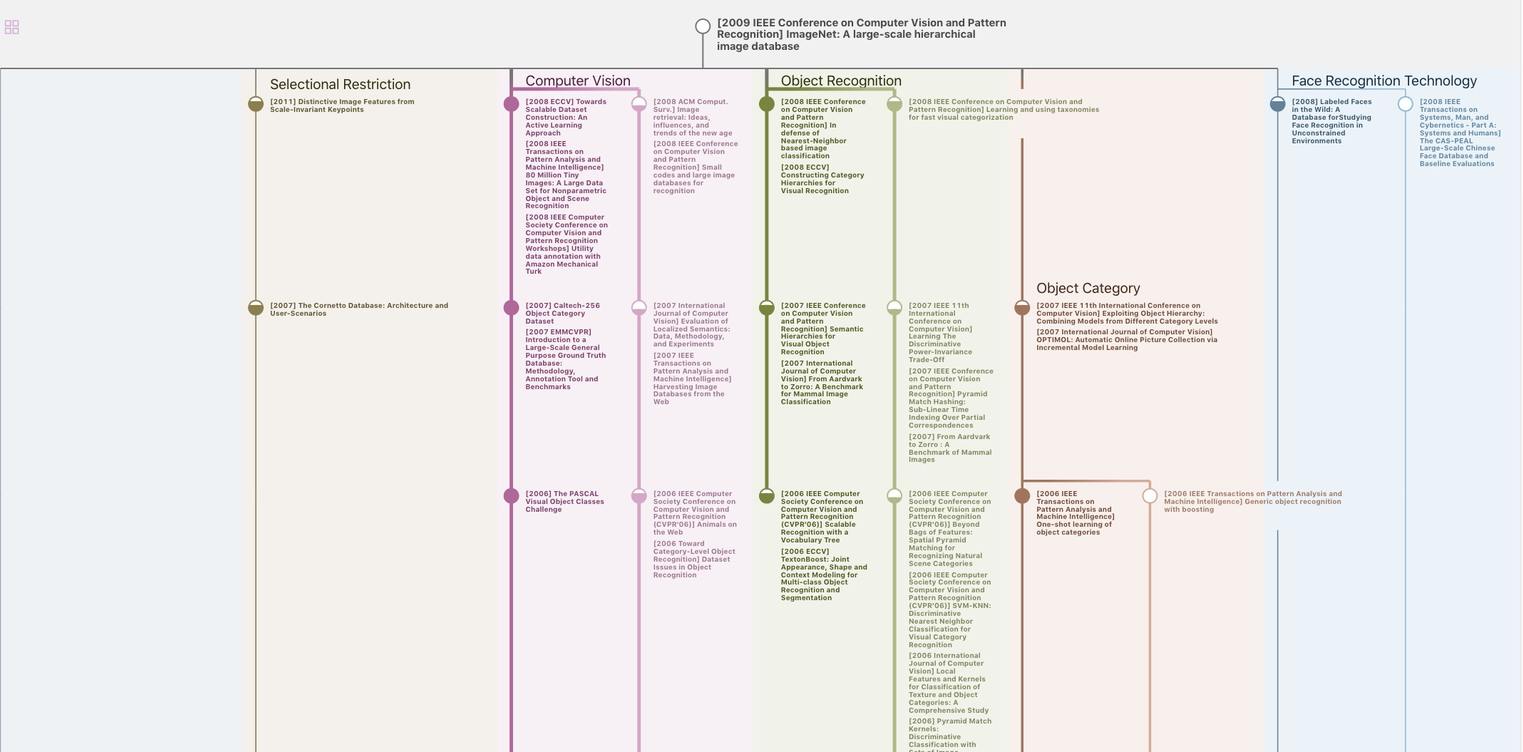
生成溯源树,研究论文发展脉络
Chat Paper
正在生成论文摘要