Neural Selectivity for Real-World Object Size In Natural Images
biorxiv(2023)
摘要
Real-world size is a functionally important high-level visual property of objects that supports interactions with our physical environment. Critically, real-world-size is robust over changes in visual appearance as projected onto our retinae such that large and small objects are correctly perceived to have different real-world sizes. To better understand the neural basis of this phenomenon, we examined whether the neural coding of real-world size holds for objects embedded in complex natural scene images, as well as whether real-world size effects are present for both inanimate and animate objects, whether low- and mid-level visual features can account for size selectivity, and whether neural size tuning is best described by a linear, logarithmic, or exponential neural coding function. To address these questions, we used a large-scale dataset of fMRI responses to natural images combined with per-voxel regression and contrasts. Importantly, the resultant pattern of size selectivity for objects embedded in natural scenes was aligned with prior results using isolated objects. Extending this finding, we also found that size coding exists for both animate and inanimate objects, that low-level visual features cannot account for neural size preferences, and size tuning functions have different shapes for large versus small preferring voxels. Together, these results indicate that real-world size is an ecologically significant dimension in the larger space of behaviorally-relevant cortical representations that support interactions with the world around us.
### Competing Interest Statement
The authors have declared no competing interest.
更多查看译文
关键词
images,selectivity,object,size,real-world
AI 理解论文
溯源树
样例
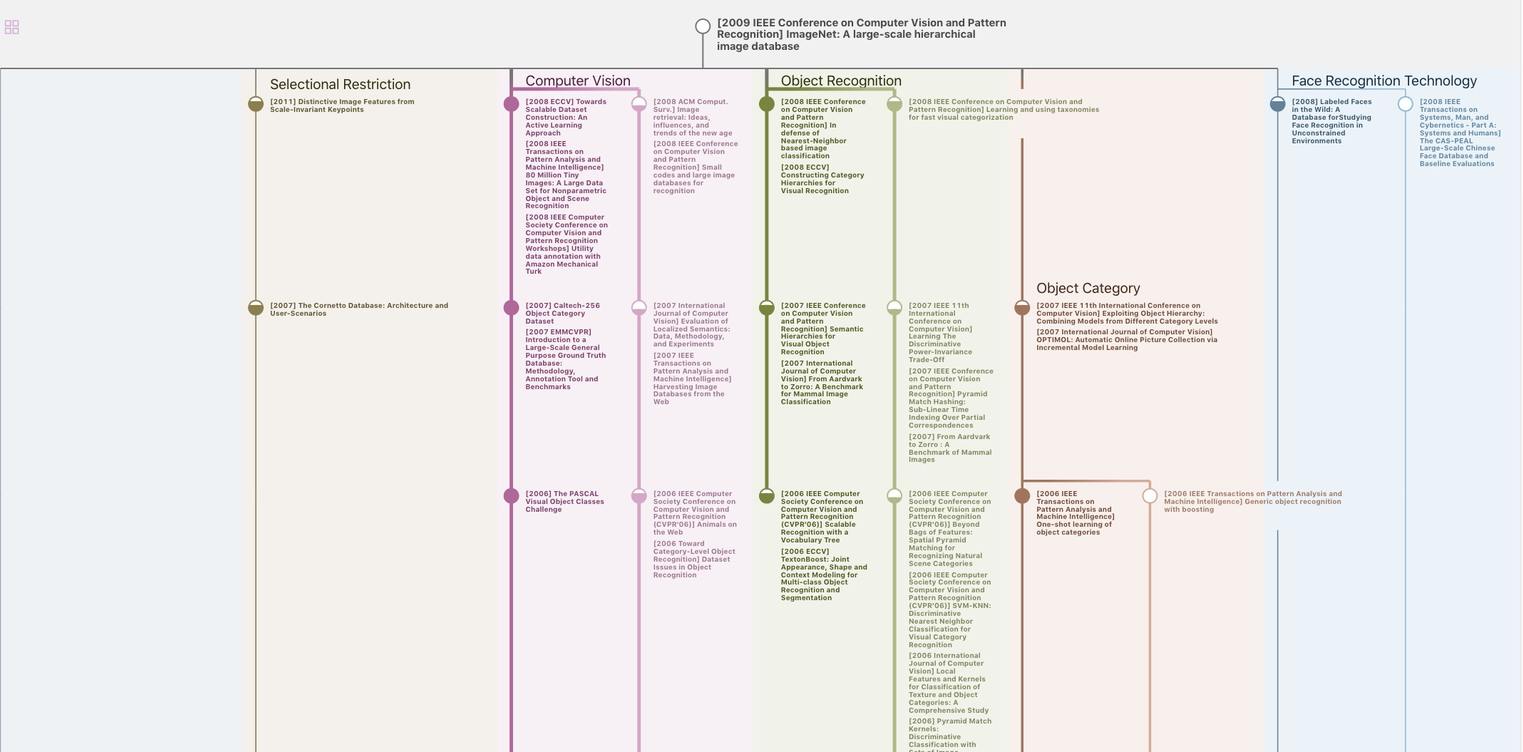
生成溯源树,研究论文发展脉络
Chat Paper
正在生成论文摘要