Accurate estimation of rare cell-type fractions from tissue omics data via hierarchical deconvolution
ANNALS OF APPLIED STATISTICS(2024)
摘要
Bulk transcriptomics in tissue samples reflects the average expression levels across different cell types and is highly influenced by cellular fractions. As such, it is critical to estimate cellular fractions to both deconfound differential expression analyses and infer cell type-specific differential expression. Since experimentally counting cells is infeasible in most tissues and studies, in silico cellular deconvolution methods have been developed as an alternative. However, existing methods are designed for tissues consisting of clearly distinguishable cell types and have difficulties estimating highly correlated or rare cell types. To address this challenge, we propose hierarchical deconvolution (HiDecon) that uses single -cell RNA sequencing references and a hierarchical cell -type tree, which models the similarities among cell types and cell differentiation relationships, to estimate cellular fractions in bulk data. By coordinating cell fractions across layers of the hierarchical tree, cellular fraction information is passed up and down the tree, which helps correct estimation biases by pooling information across related cell types. The flexible hierarchical tree structure also enables estimating rare cell fractions by splitting the tree to higher resolutions. Through simulations and real data applications with the ground truth of measured cellular fractions, we demonstrate that HiDecon outperforms existing methods and accurately estimates cellular fractions. Finally, we show the utility of HiDecon estimates in identifying the associations between cellular fractions and Alzheimer's disease.
更多查看译文
关键词
Cellular deconvolution,single-cell data,RNA sequencing,hierarchical tree,penalized regression
AI 理解论文
溯源树
样例
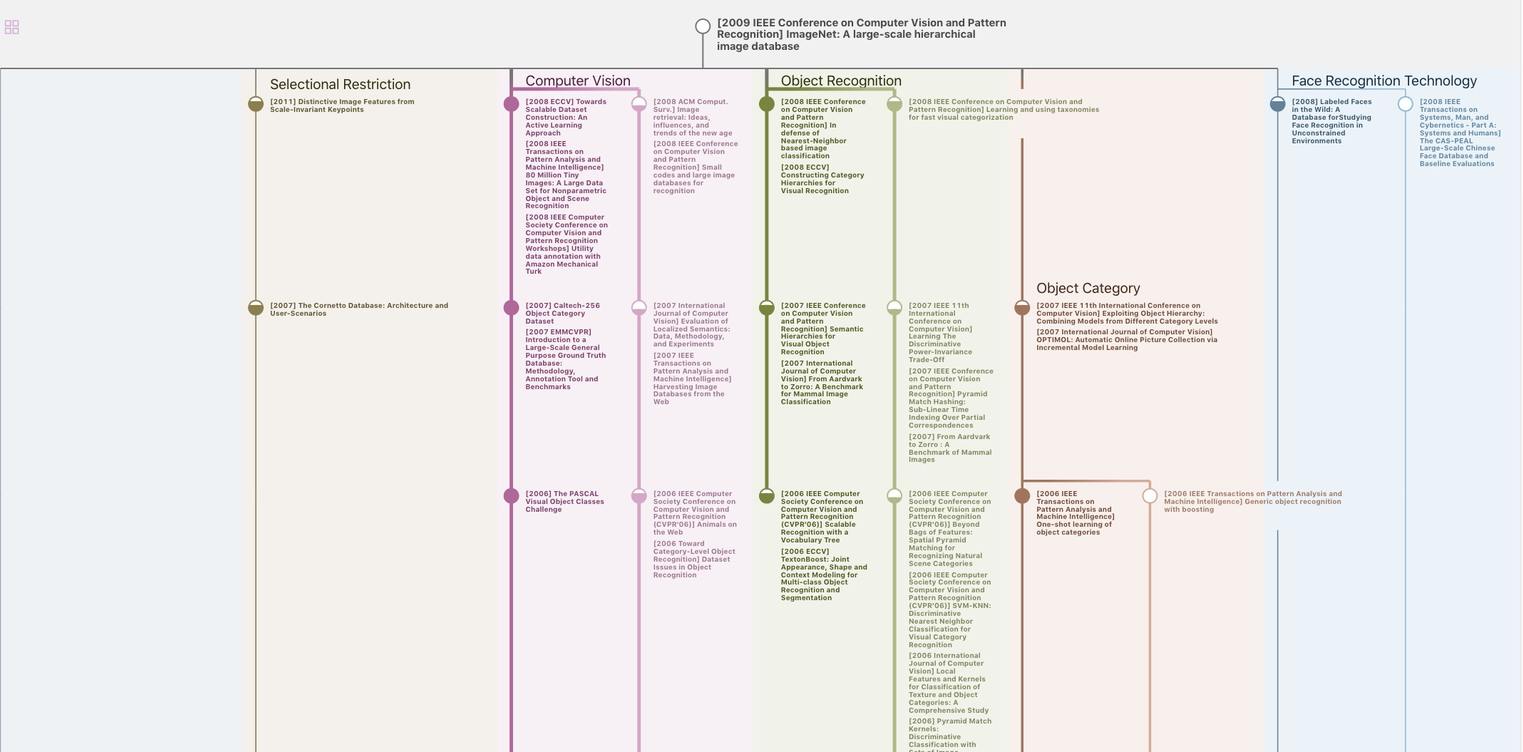
生成溯源树,研究论文发展脉络
Chat Paper
正在生成论文摘要