Skew-Robust Human-Object Interactions in Videos
WACV(2023)
摘要
Humans are, arguably, one of the most important regions of interest in a visual analysis pipeline. Detecting how the human interacts with the surrounding environment, thus, becomes an important problem and has several potential use-cases. While this has been adequately addressed in the literature in the image setting, there exist very few methods addressing the case for in-the-wild videos. The problem is further exacerbated by the high degree of label skew. To this end, we propose SERVO-HOI, a robust end-to-end framework for recognizing human-object interactions from a video, particularly in high label-skew settings. The network contextualizes multiple image representations and is trained to explicitly handle dataset skew. We propose and analyse methods to address the long-tail distribution of the labels and show improvements on the tail-labels. SERVO-HOI outperforms the state-of-the-art by a significant margin (21.1% vs 17.6% mAP) on the large-scale, in-the-wild VidHOI dataset while particularly demonstrating solid improvements in the tail-classes (19.9% vs 17.3% mAP).
更多查看译文
关键词
Algorithms: Video recognition and understanding (tracking,action recognition,etc.),Image recognition and understanding (object detection,categorization,segmentation,scene modeling,visual reasoning)
AI 理解论文
溯源树
样例
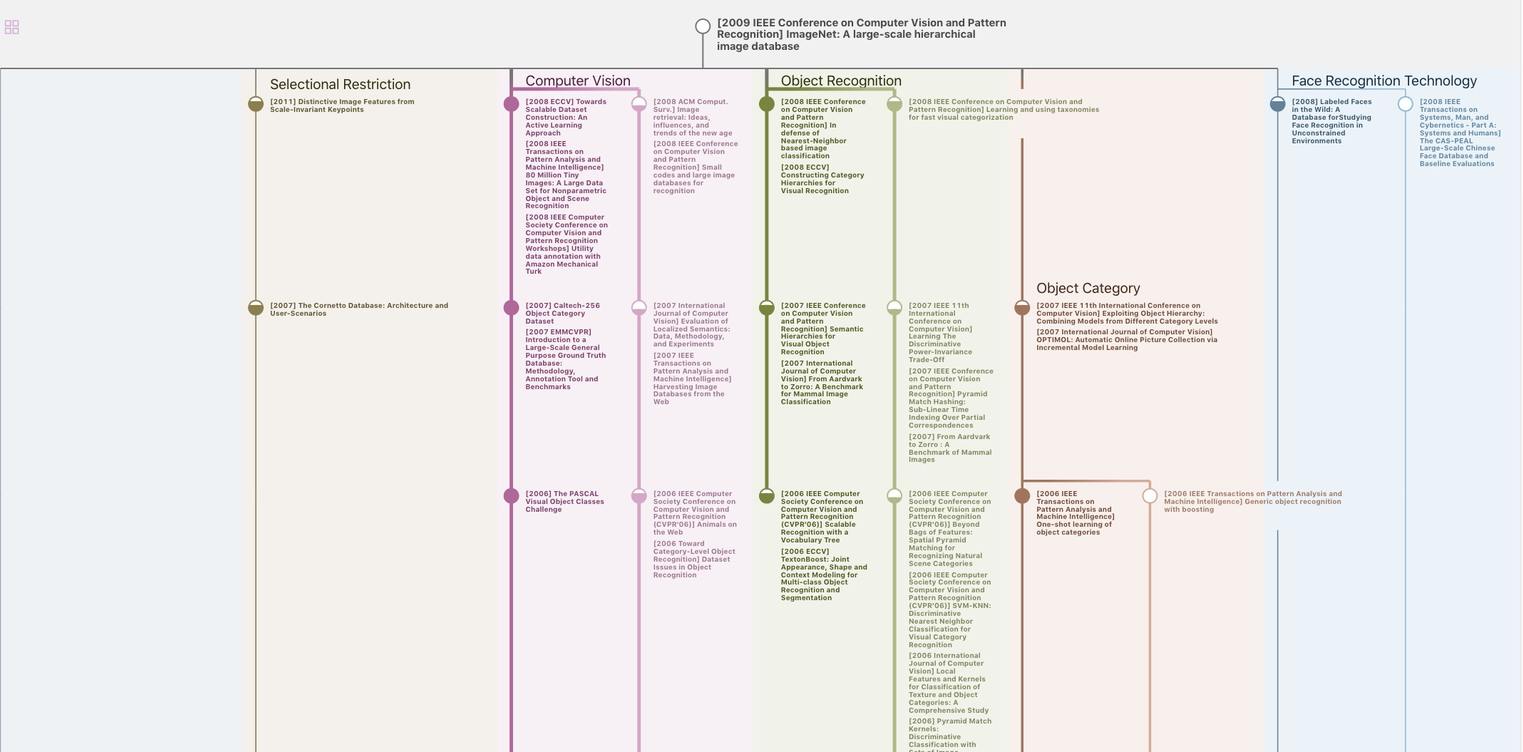
生成溯源树,研究论文发展脉络
Chat Paper
正在生成论文摘要