Self-supervised Monocular Depth Estimation from Thermal Images via Adversarial Multi-spectral Adaptation
WACV(2023)
摘要
Recently, thermal image based 3D understanding is gradually attracting attention for an illumination condition agnostic machine vision. However, the difficulty of the thermal image lies in insufficient training supervision due to its low-contrast and textureless properties. Also, introducing additional modality requires further constraints such as complicated multi-sensor calibration and synchronized data acquisition. To leverage additional modality information without such constraints, we propose a novel training framework that consists of self-supervised learning of unpaired multi-spectral images and feature-level adversarial adaptation. In the training stage, we utilize unpaired RGB/thermal video and partially shared network architecture consisting of modality-specific feature extractors and modality-independent decoder. Through the shared network design, the depth decoder can leverage the self-supervised signal of the unpaired RGB images. Feature-level adversarial adaptation minimizes the gap between RGB and thermal features and eventually makes the thermal encoder extract representative and informative features. Based on the proposed method, the trained depth network shows outperformed results than previous state-of-the-art methods.
更多查看译文
关键词
thermal images,monocular depth estimation,adaptation,self-supervised,multi-spectral
AI 理解论文
溯源树
样例
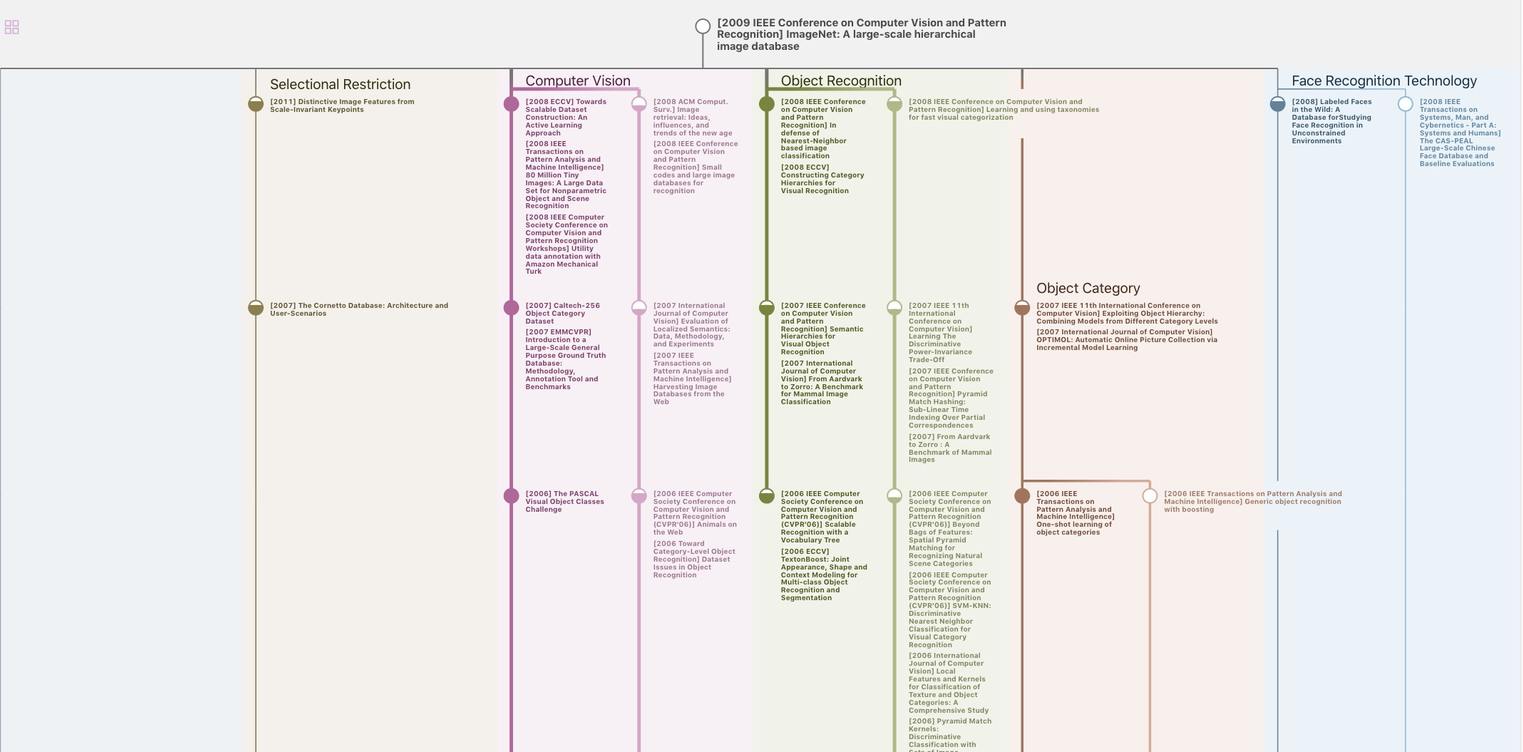
生成溯源树,研究论文发展脉络
Chat Paper
正在生成论文摘要