HandGCNFormer: A Novel Topology-Aware Transformer Network for 3D Hand Pose Estimation
WACV(2023)
摘要
Despite the substantial progress in 3D hand pose estimation, inferring plausible and accurate poses in the presence of severe self-occlusion and high self-similarity remains an inherent challenge. To mitigate the ambiguity arising from invisible and similar joints, we propose a novel Topologyaware Transformer network named HandGCNFormer, incorporating the prior knowledge of hand kinematic topology into the network while modeling long-range context information. Specifically, we present a novel Graphformer decoder with an additional node-offset graph convolutional layer (NoffGConv) that optimizes the synergy of Transformer and GCN, capturing long-range dependencies as well as local topology connection between joints. Furthermore, we replace the standard MLP prediction head with a novel Topology-aware head to better utilize local topology constraints for more plausible and accurate poses. Our method achieves state-of-the-art performance on four challenging datasets including Hands2017, NYU, ICVL, and MSRA.
更多查看译文
关键词
Algorithms: Biometrics,face,gesture,body pose,3D computer vision
AI 理解论文
溯源树
样例
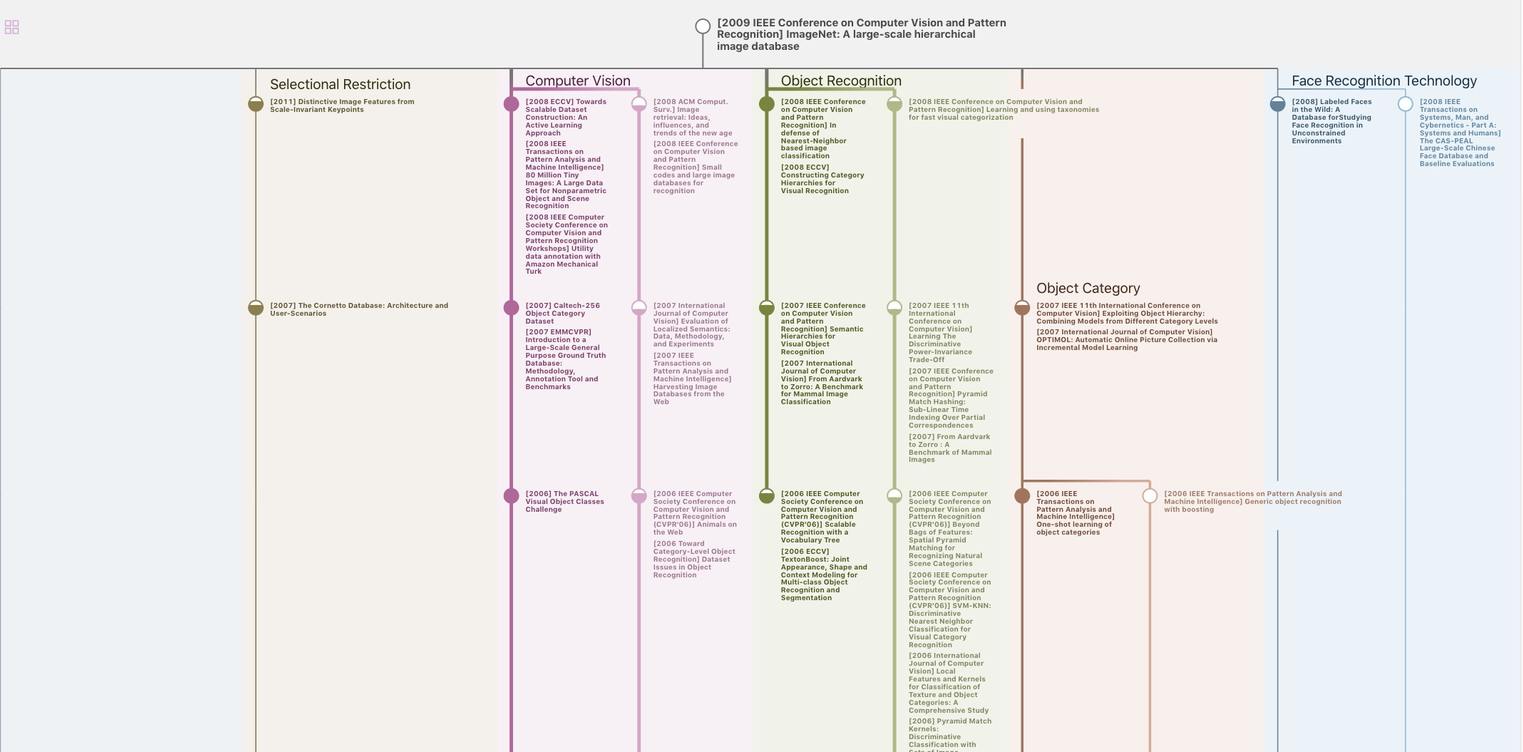
生成溯源树,研究论文发展脉络
Chat Paper
正在生成论文摘要