Unifying Margin-Based Softmax Losses in Face Recognition
WACV(2023)
摘要
In this work, we develop a theoretical and experimental framework to study the effect of margin penalties on angular softmax losses, which have led to state-of-the-art performance in face recognition. We also introduce a new multiplicative margin which performs comparably to previously proposed additive margins when the model is trained to convergence. A regime of the margin parameters can lead to degenerate minima, but these can be reliably avoided through the use of two regularization techniques that we propose. Our theory predicts the minimal angular distance between sample embeddings and the correct and wrong class prototype vectors learned during training, and it suggests a new method to identify optimal margin parameters without expensive tuning. Finally, we conduct a thorough ablation study of the margin parameters in our proposed framework, and we characterize the sensitivity of generalization to each parameter both theoretically and through experiments on standard face recognition benchmarks.
更多查看译文
关键词
softmax losses,recognition,face,margin-based
AI 理解论文
溯源树
样例
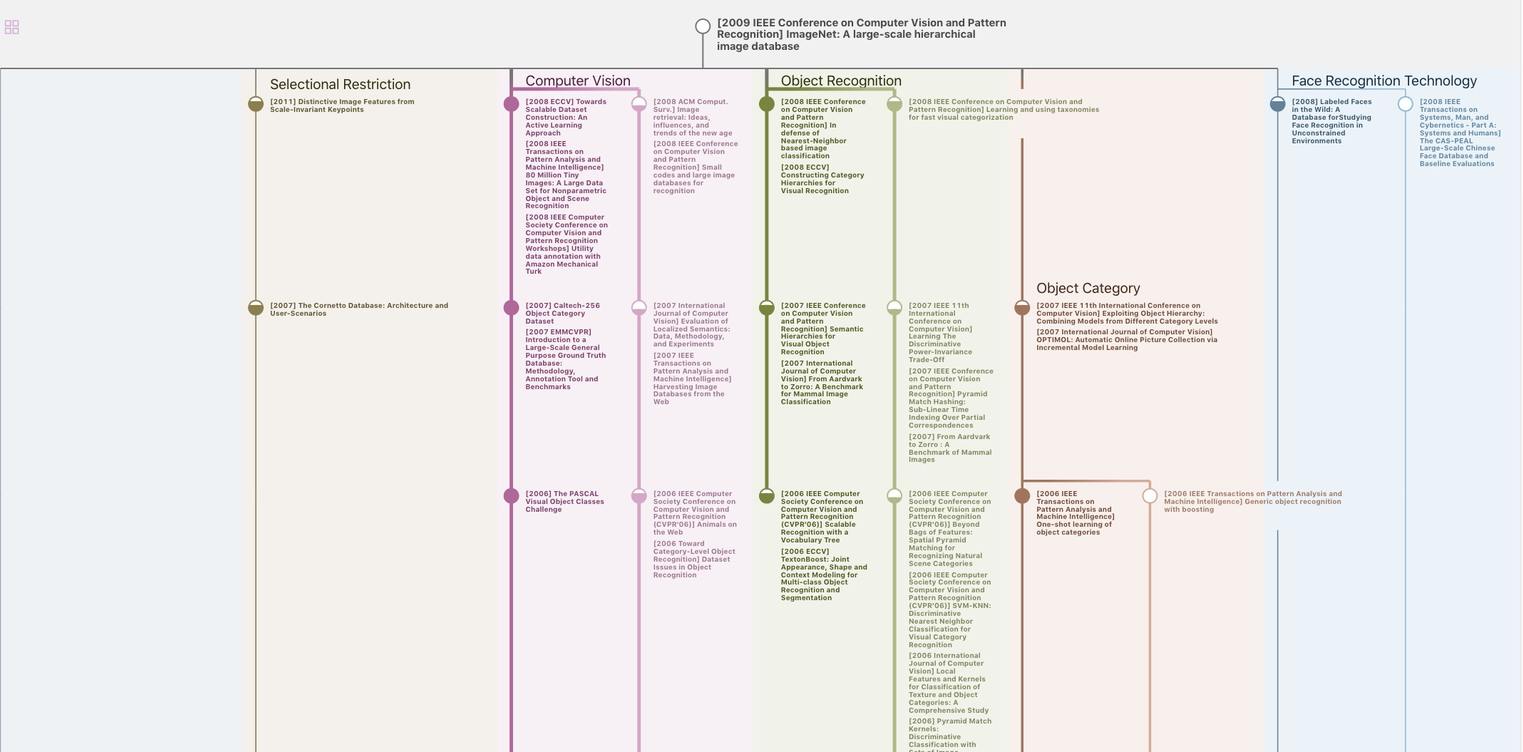
生成溯源树,研究论文发展脉络
Chat Paper
正在生成论文摘要