Online Learning of Time-Varying Unbalanced Networks in Non-Convex Environments: A Multi-Armed Bandit Approach.
IEEE Access(2023)
摘要
This study discusses how agents in a time-varying distributed network can converge to the global minimizer of a time-varying graph network. Each agent knows only the local loss of its observation and must cooperate constructively with other agents to find the global minimizer of the network. Unlike most existing works in the literature that consider a convex loss function, this study assumes a generalized local Lipschitz loss function for each agent, which can be convex or non-convex. We propose a multi-armed bandit algorithm CD EXP3 where each agent does not know its loss function but only observes its losses. Through simulations using two different time-varying graph topologies, we show that the algorithm helps all agents converge to the minimizer of the network. In addition, we discuss the effects of the two different topologies and various simulation parameters on convergence. We obtain an upper bound on the expected regret and compare it with the sublinearity of the regret bounds of well-known online distributed algorithms.
更多查看译文
关键词
Online services,Games,Multi-armed bandit problem,Probability distribution,Noise measurement,Network topology,Knowledge engineering,Online learning,multi-armed bandit,Lipschitz,regret,strongly connected graph
AI 理解论文
溯源树
样例
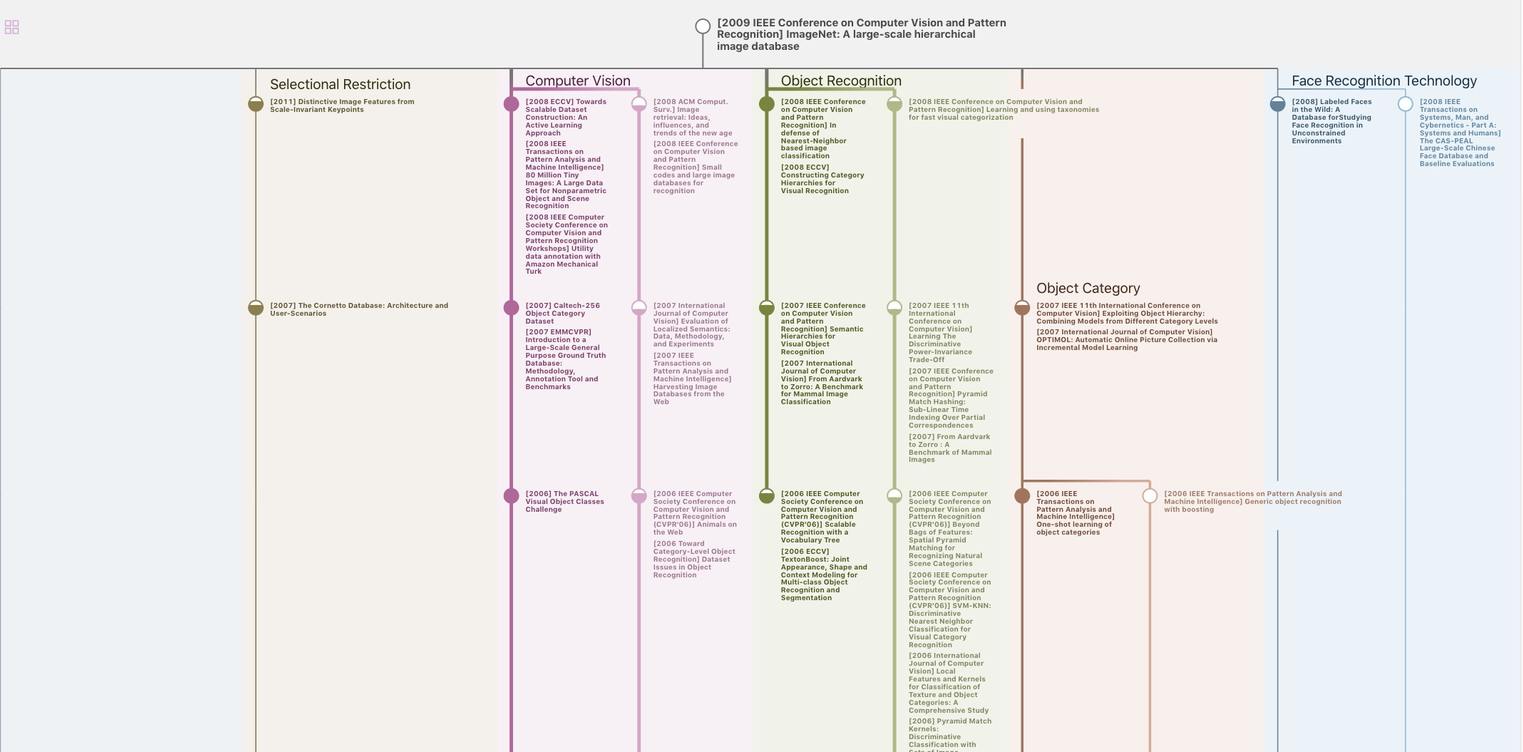
生成溯源树,研究论文发展脉络
Chat Paper
正在生成论文摘要