Pronunciation Scoring With Goodness of Pronunciation and Dynamic Time Warping.
IEEE Access(2023)
摘要
The current pronunciation scoring based on Goodness of Pronunciation (GOP) uses posterior probabilities of the Acoustic Models. Such algorithms suffer from generalization since they are utilized to determine a score metric for each phoneme rather than on the completeness or comparison with the ideal utterance of the words. This paper proposes a novel method to overcome such limitations by using combined scores of prosodic, fluency, completeness, and accuracy. This is achieved using context-aware GOP in conjugation with dynamic time warping (DTW) matching of the pitch contours of a weighted average of the context tokens found in the audio file that is rich in mispronounced phonemes. The proposed work gives flexibility in tuning the results according to different speech aspects based on a single hyperparameter. The results achieved are encouraging and have been validated on the speechocean762 dataset, where Automatic Speech Recognition (ASR) model has been trained on the Librispeech dataset. The resultant mean error of the proposed approach is 3.38% and the value of the correlation coefficient achieved is 0.652.
更多查看译文
关键词
Hidden Markov models,Acoustics,Mathematical models,Feature extraction,Mel frequency cepstral coefficient,Computer science,Computational modeling,Pronunciation scoring,goodness of pronunciation,hidden Markov model-deep neural network,dynamic time warping,kaldi,speechocean762
AI 理解论文
溯源树
样例
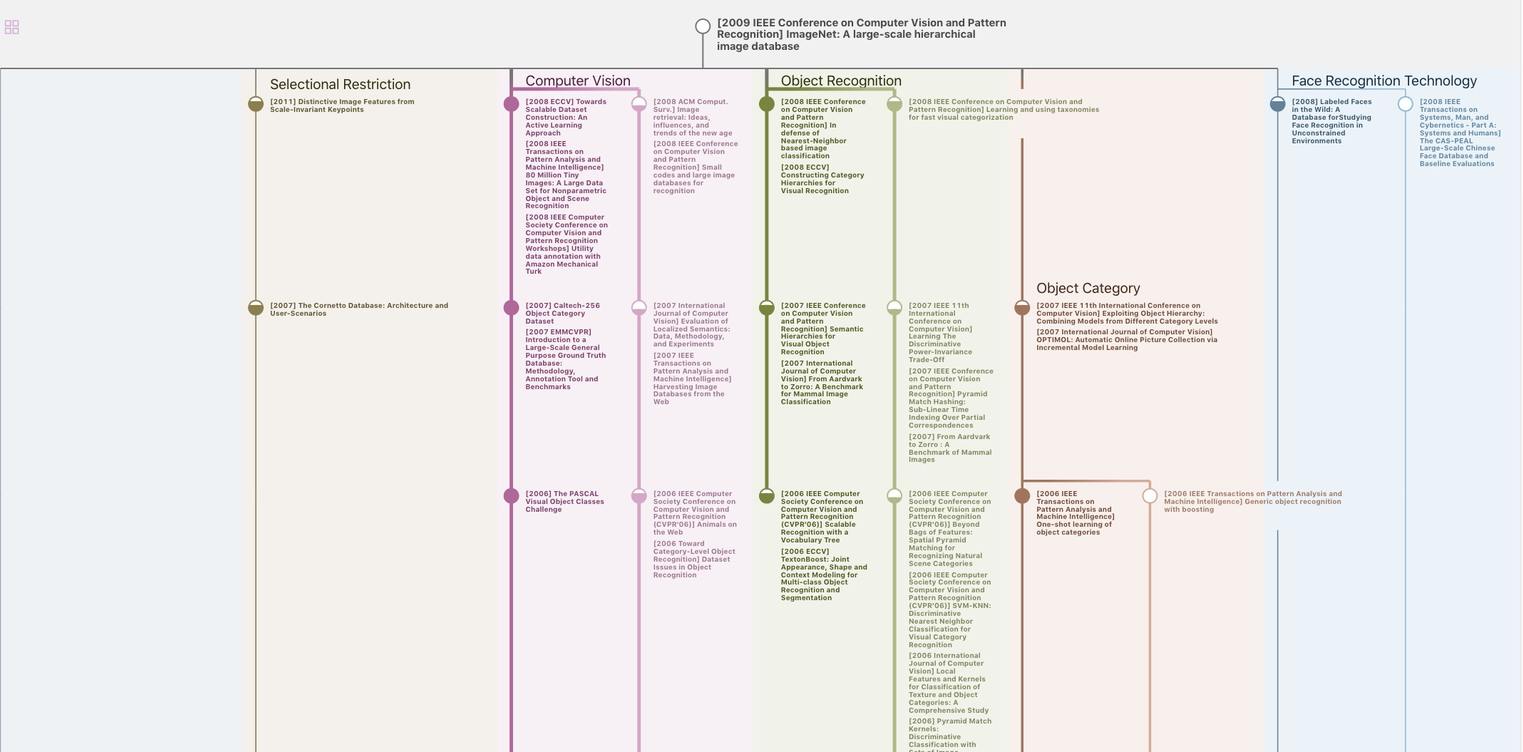
生成溯源树,研究论文发展脉络
Chat Paper
正在生成论文摘要