Wide & deep generative adversarial networks for recommendation system.
Intell. Data Anal.(2023)
摘要
Generative Adversarial Networks (GANs) has achieved great success in computer vision like Image Inpainting, Image Super-Resolution. Many researchers apply it to improve the effectiveness of recommendation system. However, GANs-based methods obtain users' preferences using a single Neural Network framework in generative model, which may not be fully mined. Furthermore, most GANs-based algorithms adopt cross-entropy loss to get pair-wise bias, but these methods don't reveal global data distribution loss when data are sparse. Those problems will influence the performance of the algorithm and result in poor accuracy. To address these problems, we introduce Wide & Deep Generative Adversarial Networks for Recommendation System (a.k.a W & DGAN) in this paper. On the one hand, we employ Wide & Deep Learning as a generative model capable of extracting both explicit and implicit information of user preferences. Furthermore, we combine Cross-Entropy loss in G with Wasserstein loss in D to get data distribution, then, the joint loss will be to receive the training information feedback from data distribution. Empirical results on three public benchmarks show that W&DGAN significantly outperforms state-of-the-art methods.
更多查看译文
关键词
Wide & deep learning,Generative Adversarial Networks,joint loss,recommendation system
AI 理解论文
溯源树
样例
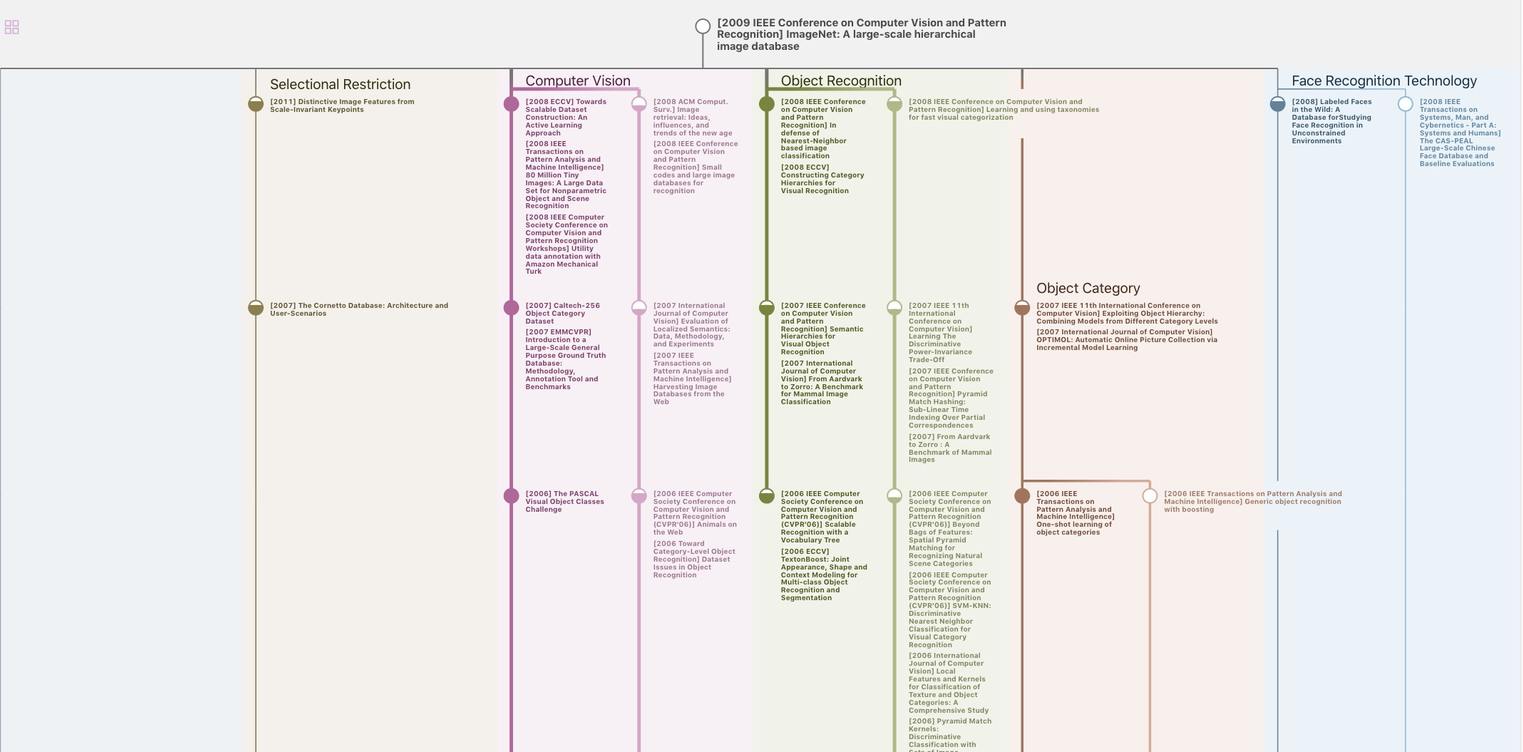
生成溯源树,研究论文发展脉络
Chat Paper
正在生成论文摘要