Topic Classification of Online News Articles Using Optimized Machine Learning Models.
Comput.(2023)
摘要
Much news is available online, and not all is categorized. A few researchers have carried out work on news classification in the past, and most of the work focused on fake news identification. Most of the work performed on news categorization is carried out on a benchmark dataset. The problem with the benchmark dataset is that model trained with it is not applicable in the real world as the data are pre-organized. This study used machine learning (ML) techniques to categorize online news articles as these techniques are cheaper in terms of computational needs and are less complex. This study proposed the hyperparameter-optimized support vector machines (SVM) to categorize news articles according to their respective category. Additionally, five other ML techniques, Stochastic Gradient Descent (SGD), Random Forest (RF), Logistic Regression (LR), K-Nearest Neighbor (KNN), and Naive Bayes (NB), were optimized for comparison for the news categorization task. The results showed that the optimized SVM model performed better than other models, while without optimization, its performance was worse than other ML models.
更多查看译文
关键词
topic categorization,model parameter tuning,hyperparameter optimization,grid search,SVM,NLP,TF-IDF,human rights,fair societies
AI 理解论文
溯源树
样例
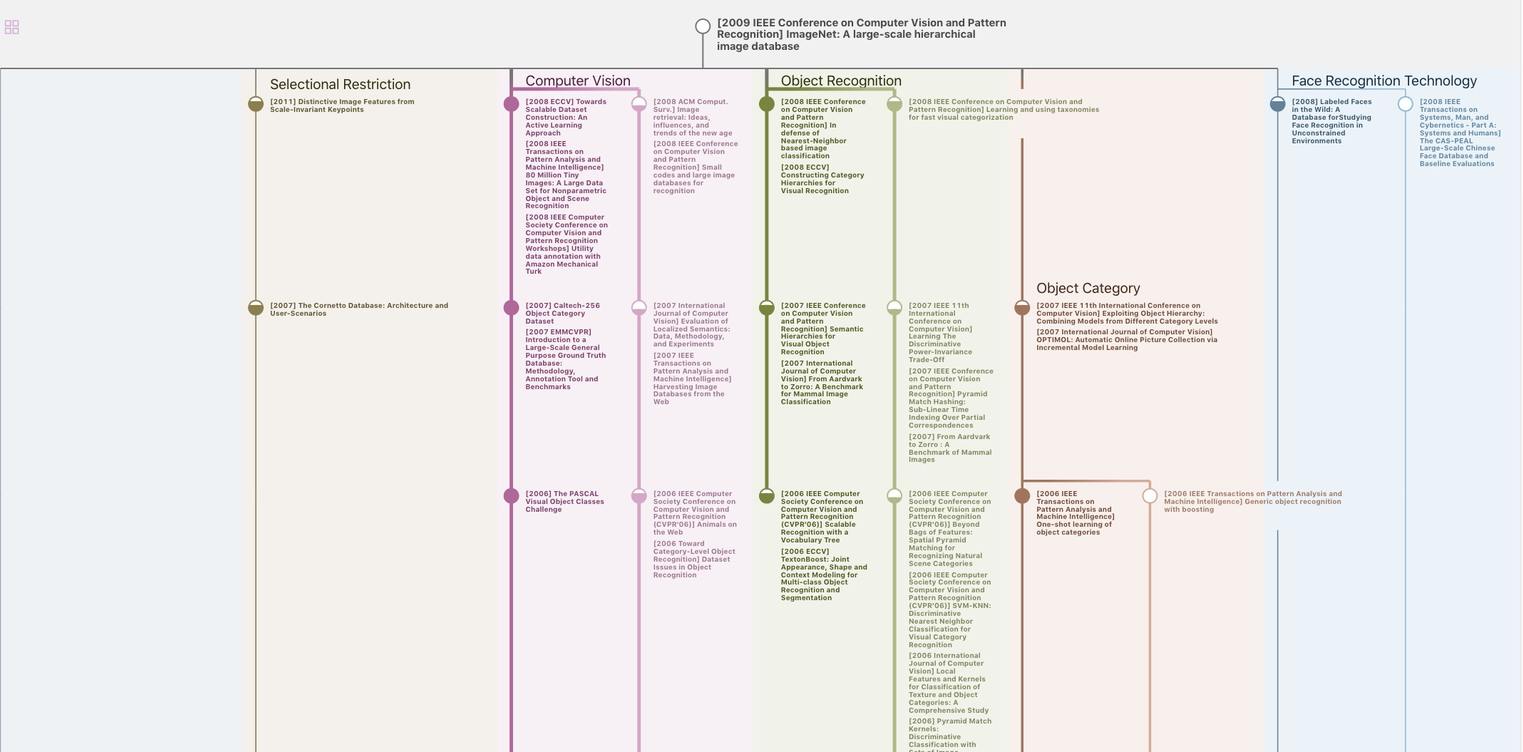
生成溯源树,研究论文发展脉络
Chat Paper
正在生成论文摘要