Federated Graph Neural Network for Fast Anomaly Detection in Controller Area Networks.
IEEE Trans. Inf. Forensics Secur.(2023)
摘要
Due to the lack of CAN frame encryption and authentication, CAN bus is vulnerable to various attacks, which can in general be divided into message injection, suspension, and falsification. Existing CAN bus anomaly detection mechanisms either can only detect one or two of these attacks, or require numerous CAN messages during predictions, which can hardly realize real-time performance. In this paper, we propose a CAN bus anomaly detection system that can detect all these attacks simultaneously in as short as 3 milliseconds (ms) based on Graph Neural Network (GNN). This work generates directed attributed graphs based on CAN message streams in given message intervals. Node attributes denote data contents in CAN messages while each edge attribute represents the frequency of a typical CAN ID pair in the given interval. Afterwards, a GNN is trained based on generated CAN message graphs. Considering highly imbalanced training data, a two-stage classifier cascade is developed in this paper, which is composed of a one-class classifier for anomaly detection and a multi-class classifier for attack classification. An openmax layer is further introduced to the multi-class classifier to tackle new anomalies from unknown classes. To take advantage of crowdsourcing while protecting user data privacy, we adopt federated learning to train a universal model that covers different driving scenarios and vehicle states. Extensive experiment results show the effectiveness and efficiency of our methodology.
更多查看译文
关键词
Intrusion detection,Anomaly detection,Training,Electronic mail,Delays,Data privacy,Data models,CAN bus intrusion detection,graph neural network,two-stage classifier cascade,federated learning
AI 理解论文
溯源树
样例
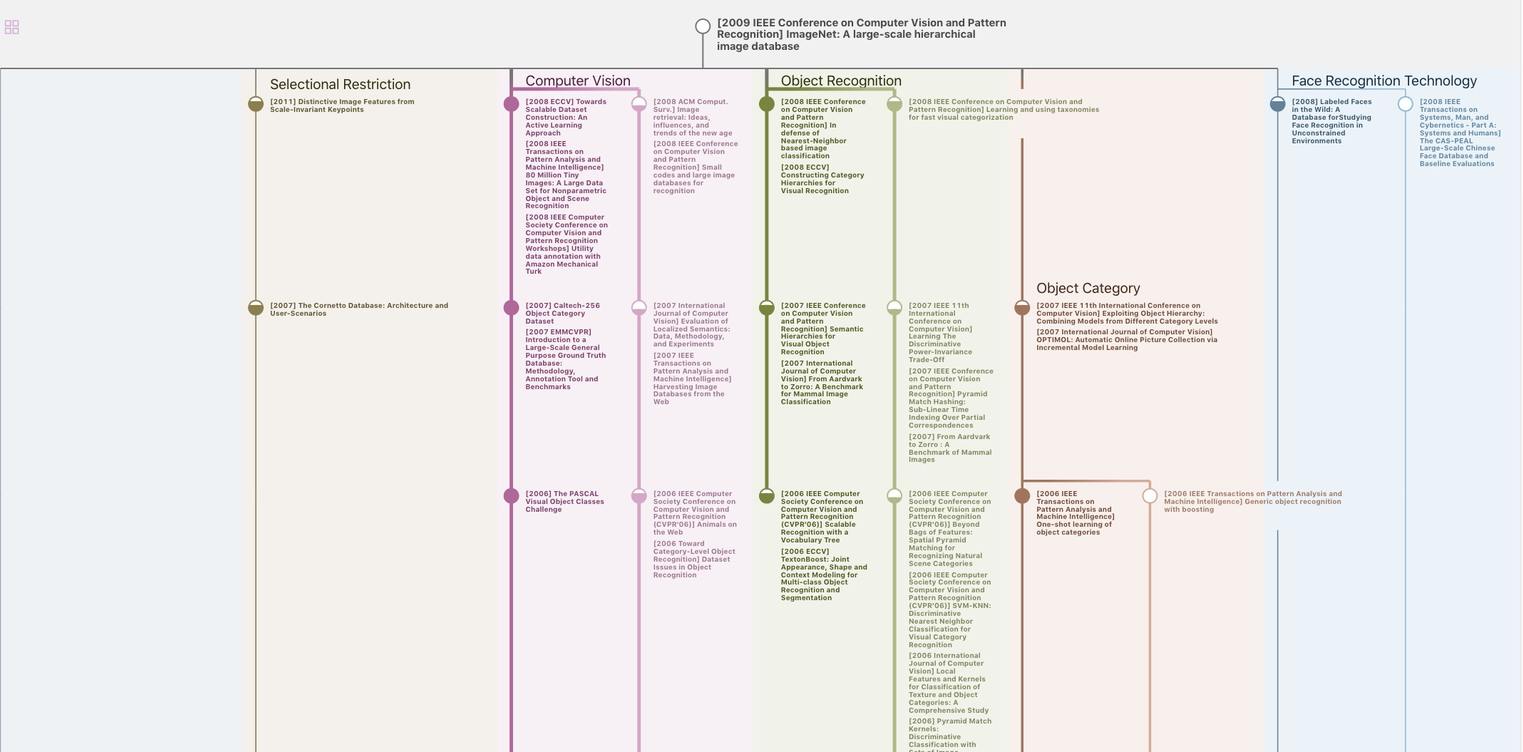
生成溯源树,研究论文发展脉络
Chat Paper
正在生成论文摘要