A Comparison of Machine Learning and Empirical Approaches for Deriving Bathymetry from Multispectral Imagery.
Remote. Sens.(2023)
摘要
Knowledge of the precise water depth in shallow areas of the ocean is of great significance to the safe navigation of ships and hydrographic surveying. Compared with traditional bathymetry, satellite remote sensing for water depth determination makes it possible to cover large areas by dynamic observation. In this paper, we conducted an optically shallow water bathymetric inversion study using a Stumpf empirical model, random forest model, neural network model, and support vector machine model based on Sentinel-2 satellite images and Ganquan Dao measured bathymetry data. We compared and analyzed the inversion results based on the empirical model and different machine learning models. The results show that the Stumpf empirical and machine learning models are capable of inverting optically shallow water depth. Moreover, the machine learning models had better fitting ability than the Stumpf empirical model with a sufficient number of samples, especially when the water depth was greater than 15 m. In addition, the random forest model had the highest overall accuracy among these models, with a root mean square error (RMSE) of 1.41 m and a regression coefficient (R-2) of 0.96 for the test data.
更多查看译文
关键词
shallow water depth inversion,satellite remote sensing,machine learning,empirical model
AI 理解论文
溯源树
样例
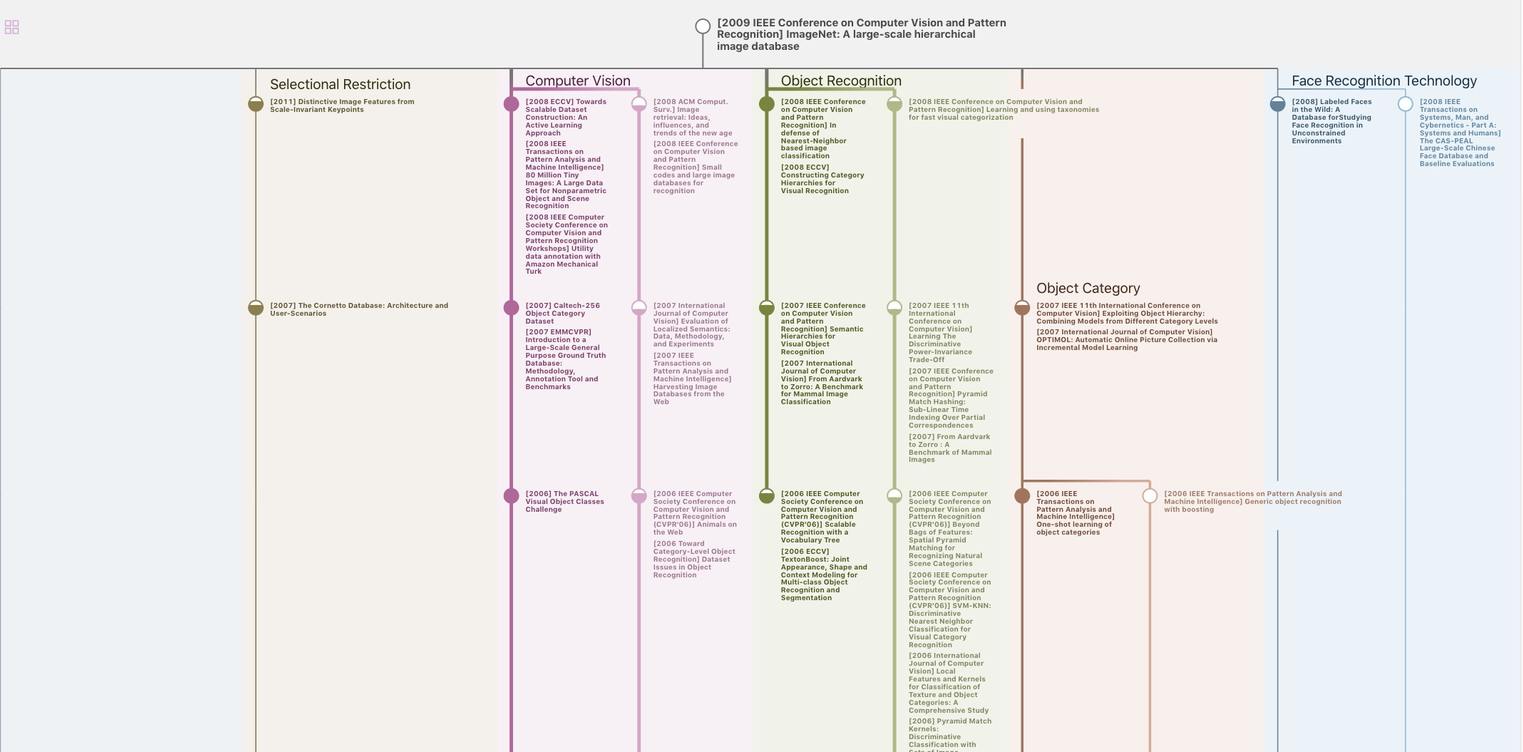
生成溯源树,研究论文发展脉络
Chat Paper
正在生成论文摘要