Dynamic differential entropy and brain connectivity features based EEG emotion recognition.
Int. J. Intell. Syst.(2022)
摘要
Emotion recognition has become a research focus in the brain-computer interface and cognitive neuroscience. Electroencephalogram (EEG) is employed for its advantages as accurate, objective, and noninvasive nature. However, many existing research only focus on extracting the time and frequency domain features of the EEG signals while failing to utilize the dynamic temporal changes and the positional relationships between different electrode channels. To fill this gap, we develop the dynamic differential entropy and brain connectivity features based EEG emotion recognition using linear graph convolutional network named DDELGCN. First, the dynamic differential entropy feature which represents the frequency domain feature as well as time domain feature is extracted based on the traditional differential entropy feature. Second, brain connectivity matrices are constructed by calculating the Pearson correlation coefficient, phase-locked value and transfer entropy, and then are used to denote the connectivity features of all electrode combinations. Finally, a linear graph convolutional network is customized and applied to aggregate the features from total electrode combinations and then classifies the emotional states, which consists of five layers, namely, an input layer, two linear graph convolutional layers, a fully connected layer, and a softmax layer. Extensive experiments show that the accuracies in the valence and arousal dimensions reach 90.88% and 91.13%, and the precision reaches 96.66% and 97.02% on the DEAP dataset, respectively. On the SEED dataset, the accuracy and precision reach 91.56% and 97.38%, respectively.
更多查看译文
关键词
brain connectivity feature,dynamic differential entropy,electroencephalography,emotion recognition,linear graph convolution network
AI 理解论文
溯源树
样例
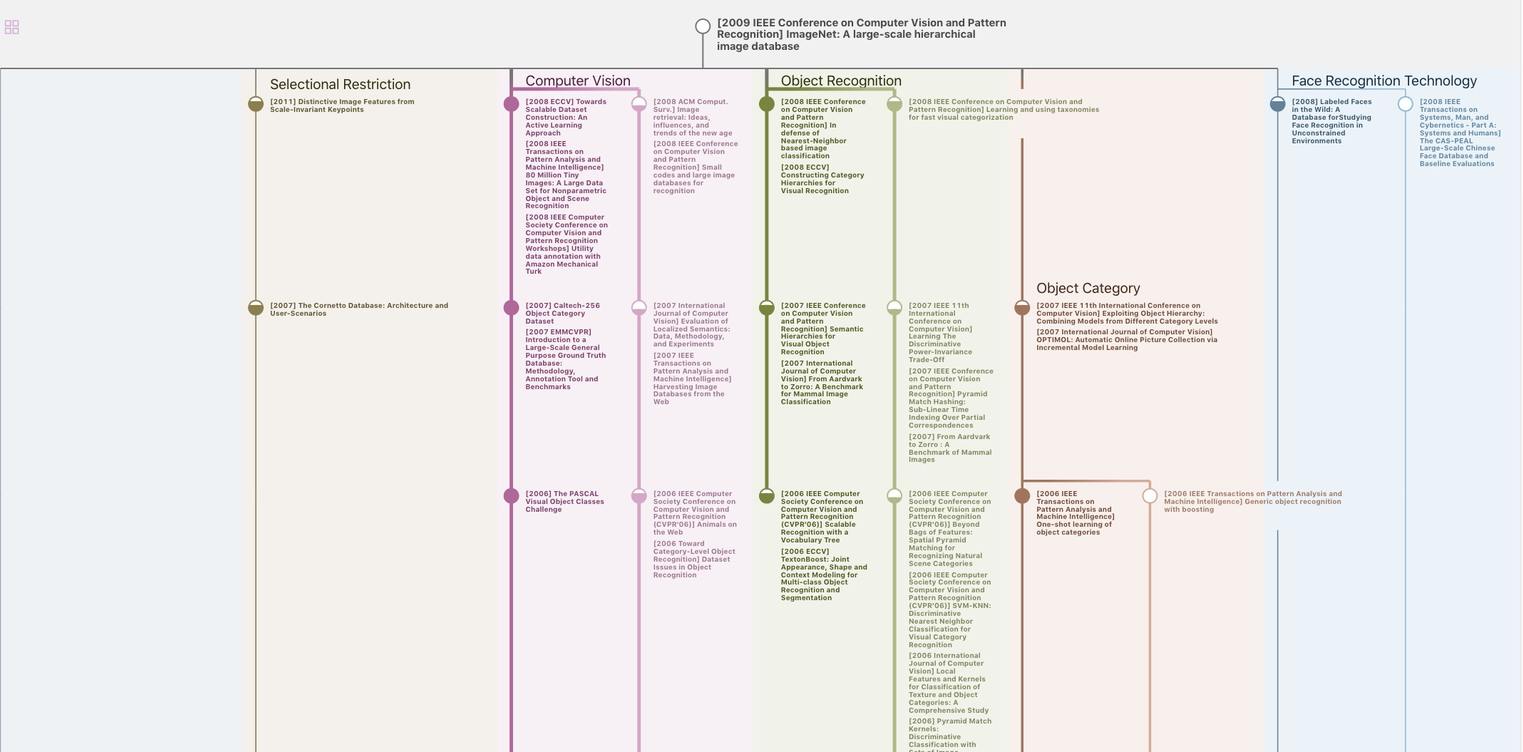
生成溯源树,研究论文发展脉络
Chat Paper
正在生成论文摘要