Surface Material Classification Based on Unbalanced Visual and Haptic Data: A Double-DQN Method.
IEEE Trans. Instrum. Meas.(2023)
摘要
The article focuses on surface material classification with unbalanced visual and haptic data, which is important in teleoperation and robotic recognition. For this problem, existent classification methods inevitably suffer from performance degradation, as they tend to emphasize the major classes and ignore the minor ones. To overcome such an issue, we address this classification problem by the double deep $Q$ -learning network (DDQN) method which not only offers strong representation ability but also avoids overestimation. Specifically, we first transform haptic accelerations to their spectrograms by short-time Fourier transform (STFT), and, respectively, feed visual images and haptic spectrograms to pretrained ResNet50 for extracting low-dimensional feature vectors. Then, the hybrid visual-haptic feature vectors are fed into DDQN as a sequence of states. With respect to each state, DDQN assigns it with an estimated class label. By comparing with the true label, DDQN earns a reward that is dependent on the imbalance ratio of the class. Through maximization of the cumulative rewards, DDQN enhances its classification performance. For the purposes of validation, numerical evaluations are carried out on the TUM69, LMT108, and HaTT datasets. The results show that DDQN outperforms the existent methods in both classification performance and computational complexity.
更多查看译文
关键词
Haptic interfaces,Visualization,Feature extraction,Residual neural networks,Spectrogram,Deep learning,Convolutional neural networks,Double deep Q-learning network (DDQN),hybrid visual-haptic data,residual network,surface material classification,unbalanced measurement
AI 理解论文
溯源树
样例
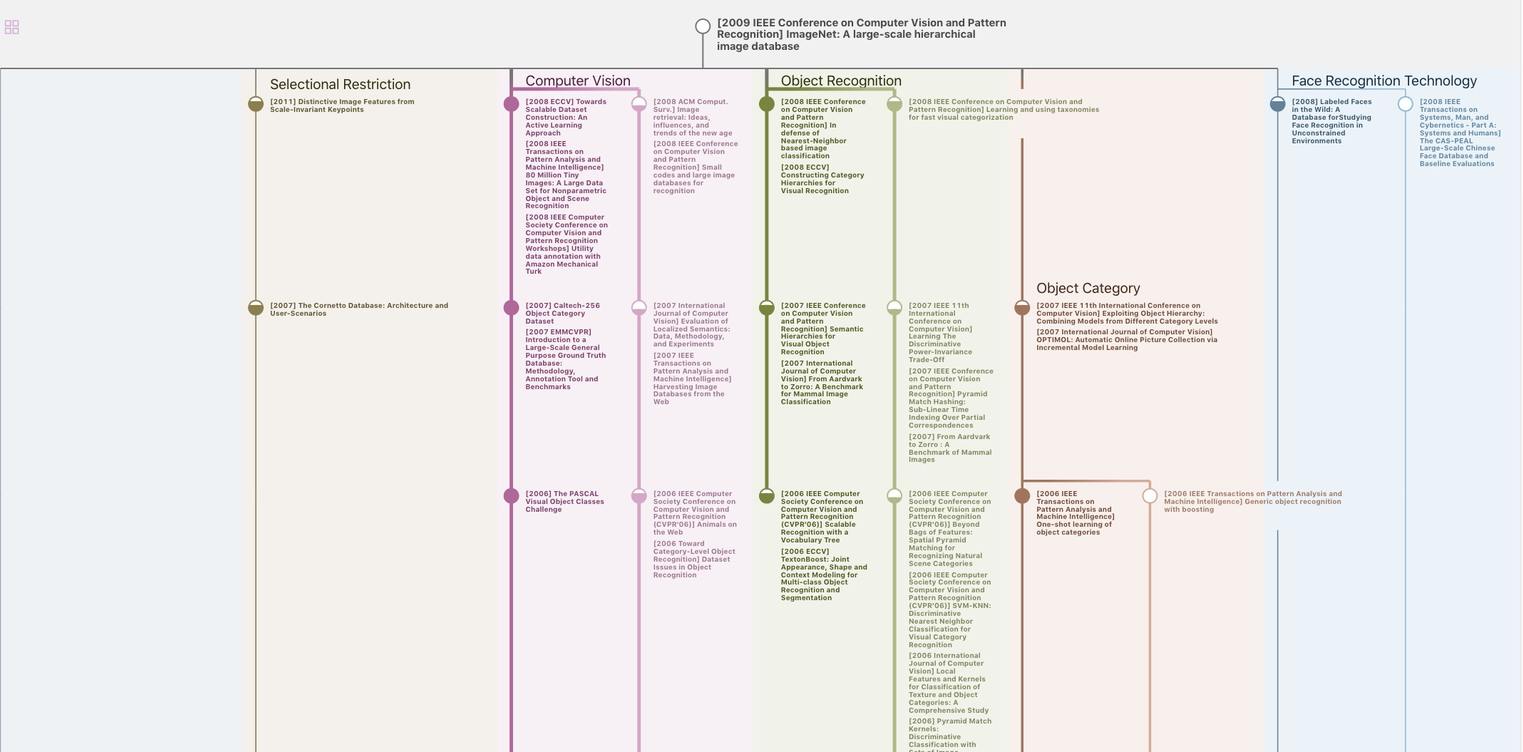
生成溯源树,研究论文发展脉络
Chat Paper
正在生成论文摘要